Tag: <span>autonomous vehicles</span>
TQ of Robotics
Booming robot market
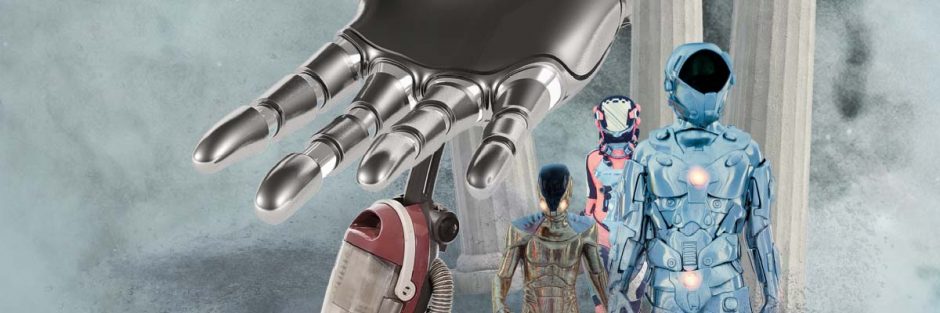
It started around 50 years ago with just a narrow range of applications for the first robots. Since then, they have been growing ever more flexible and cheaper. Robotics applications and market volumes are rising steadily. With the service robots that will soon be on sale, the mechatronic helpers will have finally arrived on the mass market.
The robot market is in a period of change. As personal assistants, autonomous vehicles, surgical assistants or flying drones, robots are now also invading areas beyond their original industrial applications. According to the market research organisation Tractica, in 2016 for the first time more money was earned from non-industrial robots than robots working in factories.
That does not mean, however, that fewer industrial robots are being used. The International Federation of Robotics (IFR) forecasts global growth of at least 13 per cent a year on average through to 2019. By then, more than 1.4 million new industrial robots in total will have been installed in factories around the globe. Market analyst MarketsandMarkets forecasts that the industrial robot market will be worth 79.58 billion US dollars by 2022. According to IFR, the strongest driver of growth in the robotics sector is China, which is forecast to account for 40 per cent of global industrial robot sales alone by 2019.
Alongside industrial robots, service robots are conquering the market. According to IFR, their sales for professional applications such as in medicine, agriculture and logistics totalled 4.6 billion dollars in 2015. Further dynamic growth in demand is forecast for the period from 2016 to 2019. The cumulative value will rise to 23 billion dollars. In addition to the established market for professional service robots, the consumer segment – from vacuum cleaners to technical entertainment artistes – is now also growing steadily. According to IFR, sales of such personal-use service robots increased by 16 per cent in 2015, reaching a cumulative value of 22 billion dollars. An interesting question in relation to this comparatively new market segment is how the start-up scene will develop – given that it offers unique opportunities for innovative new businesses to conquer a market on which no major robot manufacturers are yet established.
Tractica predicts that the robotics industry in general – including autonomous vehicles and aircraft – is going to see a real boom, with global robot sales rising from 34.1 billion dollars in 2016 to 226.2 billion dollars by 2021 – representing an impressive 46 per cent average annual growth rate.
From pure fiction to a real market opportunity
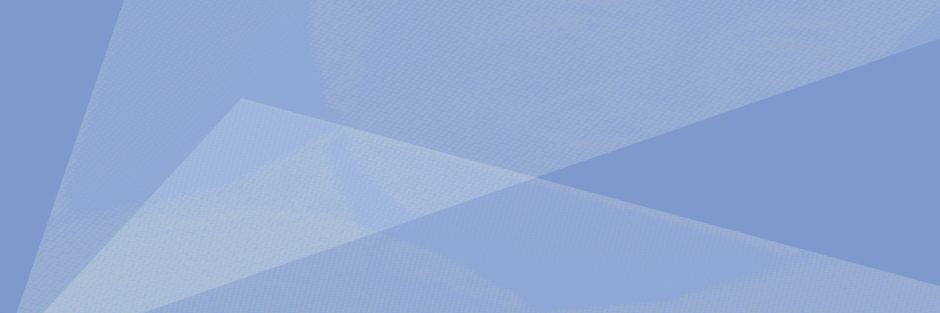
Intelligent machines and self-teaching computers will open up exciting prospects for the electronics industry.
The idea of thinking, or even feeling, machines was long merely a vision of science-fiction authors. But thanks to rapid developments in semiconductors and new ideas for the programming of self-teaching algorithms, Artificial Intelligence (AI) is today a real market, opening up exciting prospects for businesses.
According to management consultants McKinsey, the global market for AI-based services, software and hardware is set to grow by as much as 25 per cent a year, and is projected to be worth USD 130 billion by 2025. Investment in AI is therefore booming, as the survey “Artificial Intelligence: the next digital frontier” by the McKinsey Global Institute affirms. It reports that, in the last year, businesses – primarily major tech corporations such as Google and Amazon – spent as much as USD 27 billion on in-house research and development in the field of intelligent robots and self-teaching computers. A further USD 12 billion was invested in AI in 2016 externally – that is, by private equity companies, by risk capital funds, or through mergers and acquisitions. This amounted in total to some USD 39 billion – triple the volume seen in 2013. Most current external investments (about 60 per cent) is flowing into machine learning (totalling as much as USD 7 billion). Other major areas of investment include image recognition (USD 2.5 to 3.5 billion) and voice recognition (USD 600 to 900 million).
Intelligent machines and self-teaching computers are opening up new market opportunities in the electronics industry. Market analyst TrendForce predicts that global revenues from chip sales will increase by 3.1 per cent a year between 2018 and 2022. It is not just the demand for processors that is rising, however; applications of AI are also driving new solutions in electronics fields such as sensor technology, hardware accelerators and digital storage media. Market research organisation marketsandmarkets, for example, forecasts a rise from USD 2.35 billion in 2017 to USD 9.68 billion by 2023 – among other reasons as a result of big data, the Internet of Things, and applications relating to Artificial Intelligence. The creation of AI-based services is also increasing demand for higher-performance network infrastructures, data centres and server systems.
AI is thus a vital market for the electronics industry as well. With our semiconductor solutions, experienced experts and extensive partner network, we will be glad to help you develop exciting new products.
Sensors as a basis for AI
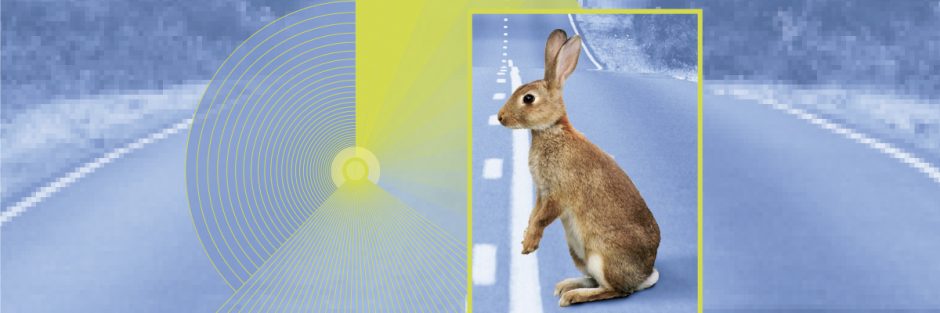
Sensor fusion allows increasingly accurate images of the environment to be developed by fusing data from different sensors. To achieve faster results and reduce the flood of data, the sensors themselves are becoming intelligent too.
Systems with Artificial Intelligence need data. The more data, the better the results. This data can either originate in databases – or it can be recorded using sensors. Sensors measure vibrations, currents and temperatures on machines, for example, and thus provide an AI system with information for predicting when maintenance is due. Others – integrated in wearables – record pulse, blood pressure and perhaps blood sugar levels in people in order to draw conclusions regarding the state of health.
Sensor technology has gained considerable momentum in recent years from areas such as mobile robotics and autonomous driving: for vehicles to move autonomously through an environment, they have to recognise the surroundings and be able to determine the precise position. To do this, they are equipped with the widest array of sensors: ultrasound sensors record obstacles at a short distance, for example when parking. Radar sensors measure the position and speed of objects at a greater distance. Lidar sensors (light detection and ranging) use invisible laser light to scan the environment and deliver a precise 3D image. Camera systems record important optical information such as the colour and contour of an object and can even measure the distance over the travel time of a light pulse.
More information is needed
Attention today is no longer focusing solely on the positioning of an object, rather information such as orientation, size or also colour and texture is also becoming increasingly important. Various different sensors have to work together to ensure this information is captured reliably. That’s because every sensor system offers specific advantages. However, it is only by fusing the information from the different sensors – in a process known as sensor fusion – that a precise, complete and reliable image of the surroundings is generated. A simple example of this are motion sensors, such as those used in smartphones, among other devices: only by combining accelerometer, magnetic field recognition and gyroscope can these sensors measure the direction and speed of a movement.
Sensors are also becoming intelligent
Not only can modern sensor systems deliver data for AI, they can also use it: such sensors can therefore pre-process the measurement data and thus ease the burden on the central processor unit. The AEye start-up developed an innovative hybrid sensor, for example, which combines camera, solid-state lidar and chips with AI algorithms. It overlays the 3D pixel cloud of the lidar with the camera’s 2D pixels and thus delivers a 3D image of the environment in colour. The relevant information is then filtered from the vehicle’s environment using AI algorithms and evaluated. The system is not only more precise by a factor of 10 to 20 and three times faster than individual lidar sensors, it also reduces the flood of data to central processor units.
Sensors supply a variety of information to the AI system
- Vibration
- Currents
- Temperature
- Position
- Size
- Colour
- Texture
- and much more…
AI smarter than humans?
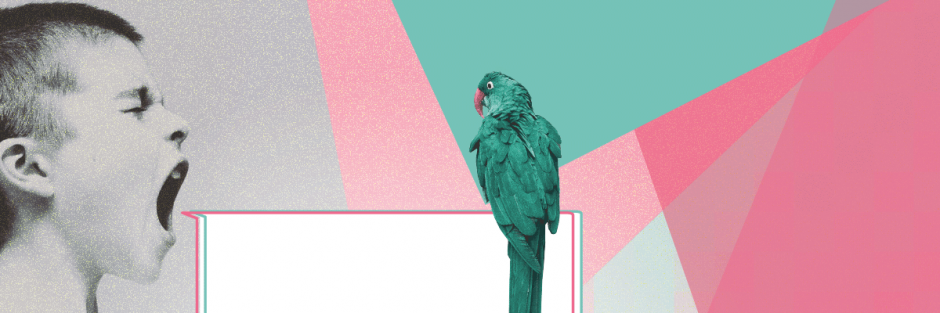
What began in the 1950s with a conference has grown into a key technology. It is already influencing our lives today, and as the intelligence of machines increases in the future that influence is bound to spread much more. But is AI smarter than humans?
Smart home assistants order products online on demand. Chatbots talk to customers with no human intermediary. Self-driving cars transport the occupants safely to their destination, while the driver is engrossed in a newspaper. All of those are applications that are already encountered today in our everyday lives – and they all have something in common: they would not be possible without Artificial Intelligence.
Artificial Intelligence is a key technology which, in the years ahead, will have a major impact not only on our day-to-day lives but also on the competitiveness of the economy as a whole. “Artificial Intelligence has enormous potential for improving our lives – in the healthcare sector, in education, or in public administration, for example. It offers major opportunities to businesses, and has already attained an astonishingly high degree of acceptance among the public at large,” says Achim Berg, president of industry association Bitkom.
How everything began
Developments in this technology began as far back as the 1950s. The term Artificial Intelligence was actually coined even before the technology had truly existed, by computer scientist John McCarthy at a conference at Dartmouth University in the USA in 1956. The US government became aware of AI, and saw potential advantages from it in the Cold War, so it provided McCarthy and his colleague Marvin Minsky with the financial resources necessary to advance the new technology. By 1970, Minsky was convinced: “In from three to eight years, we will have a machine with the general intelligence of an average human being.” But that was to prove excessively optimistic. Scientists around the world made little progress in advancing AI, so governments began cutting funding. A kind of AI winter closed in. It was only in 1980 that efforts to develop intelligent machines picked up pace again. They culminated in a spectacular battle: in 1997, IBM’s supercomputer Deep Blue defeated chess world champion Garry Kasparov.
Bots are better gamers
AI began to advance rapidly from then on. Staying with games of a kind, the progress being made was demonstrated by the victory of a bot developed by OpenAI against a team of professional players in the multiplayer game Dota 2 – one of the most complex of all video games. What was so special about this triumph was that the bot taught itself the game in just four months. By continuous trial and error over huge numbers of rounds played against itself, the bot discovered what it needed to do to win. The bot was only set up to play a one-to-one game, however – normally two teams of five play against each other. Creating a team of five bots is the OpenAI developers’ next objective. OpenAI is a non-profit research institute founded by Elon Musk with the stated aim of developing safe AI for the good of all humanity.
Intelligence doubled in two years
So, is AI already as clever as a person today? To find that out, researchers headed by Feng Liu at the Chinese Academy of Science in Beijing devised a test which measures the intelligence of machines and compares it to human intelligence. The test focused on digital assistants such as Siri and Cortana. It found that the cleverest helper of all is the Google Assistant. With a score of 47.28 points, its intelligence is ranked just below that of a six year-old human (55.5 points). That’s pretty impressive. But what is much more impressive is the rate at which the Google Assistant is becoming more intelligent. When Feng Liu first conducted the test back in 2014, the Google Assistant scored just 26.4 points – meaning it almost doubled its intelligence in two years. If the system keeps on learning at that rate, it won’t be long before Minsky’s vision expressed back in 1970 of a machine with the intelligence of a human adult becomes reality.
Simulating a human
Surprisingly, despite the long history of the development of intelligent machines, there is still no scientifically recognised definition of AI today. The term is generally used to describe systems which simulate human intelligence and behaviour. The most fitting explanation comes from renowned MIT professor Marvin Minsky, who defined AI as “the science of making machines do those things that would be considered intelligent if they were done by people”.
Artficial Intelligence | Startups
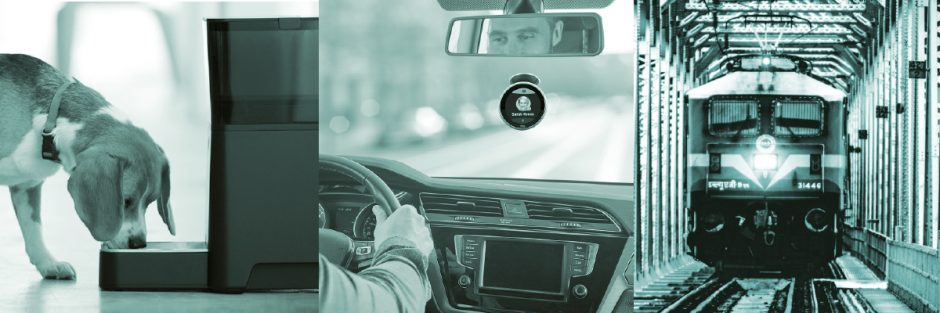
A look at the start-up scene reveals the diversity of the areas in which AI can be used. These young companies are developing products for industries as varied as healthcare, robotics, finance, education, sports, safety, and many more. We present a small selection of interesting start-ups here.
Connected Cars for Everyone
In the form of Chris, the start-up German Autolabs provides an assistant designed specifically for motorists, which easily and conveniently provides access to their smartphone via smart speech recognition and gesture control, including while driving. Chris can be integrated with any vehicle, regardless of its model and year of manufacture. The aim is to make connected car technology available to all through the combination of a flexible and scalable assistant software and hardware for retrofitting.
Make Your Own Voice Assistant
Snips is developing a new voice platform for hardware manufacturers. The service, based on Artificial Intelligence, is intended to allow developers to embed voice assistance services on any device. At the same time, a consumer version is due to be provided over the Internet, running on Raspberry Pi-powered devices. Privacy is at the forefront of this, with the system sending no data to the cloud and operating completely offline.
Realistic Simulation for Autonomous Driving Systems
Automotive Artificial Intelligence offers a virtual 3D platform which realistically imitates cars’ driving environment. It is intended to be used as a means of testing software for fully automated driving, helping to explore the systems’ limits. Self-learning agents provide the reality needed in the virtual platform. Aggressive drivers turn up just as often as overcautious ones, and there are arbitrary lane changes just as there are unforeseeable braking manoeuvres from other (simulated) vehicles that are part of the traffic.
Feeding Pets More Intelligently
With SmartShop Beta, Petnet provides a digital marketplace that guides dog and cat owners towards suitable food for their pets using Artificial Intelligence –
depending on their breed and specific needs. The start-up has itself also developed the Petnet SmartFeeder for the feeding pets. This allows the pets to be automatically supplied with individual portions. The system gives notifications for successful feeds and when food levels are low. An automatic repeat order can also be set up in the SmartShop.
Smart Water Bottle
Bellabeat has already successfully brought Healthtracker to market in the form of women’s jewellery. Building on this, the start-up has developed an intelligent water bottle with Spring. By way of sensors, its system can record how much water the user drinks, how active she is, how much she sleeps, or her stress sensitivity levels. An app, with the assistance of special AI algorithms, is used to analyse users’ individual hydration needs and give a recommendation for fluid intake.
Drone for the Danger Zone
Hivemind Nova is a quadrocopter for law enforcement, first responder and security applications. The drone learns from experience how to negotiate restricted areas or hazardous environments. Without a pilot remote-controlling, it autonomously explores dangerous buildings, tunnels, and other structures before people enter them. It transmits HD video and a map of the building layout to the user live. Hivemind Nova learns and continuously improves over time. The more it is used, the more capable it becomes.
Detecting Wear Ahead of Time
Konux combines smart sensors and analysis based on Artificial Intelligence. The solution is used on railways to monitor sets of points, for example. Field data, already pre-processed by sensor, is wirelessly transmitted to an analysis platform and combined with other data sources such as timetables, meteorological data, and maintenance logs. The data is then analysed using machinelearning algorithms to detect operational anomalies and critical wear in advance.
Greater Success with Job Posts
Textio is an advanced writing platform for creating highly effective job advertisements. By analysing the hiring outcomes of more than 10 million job listings per month, Textio predicts the impact of a job post and gives instructions in real time as to how the text could be improved. To do this, the company uses a highly sophisticated predictive engine and makes it usable for anyone – no training or IT integration needed.
AI-pioneer Minsky: Temporarily dead?
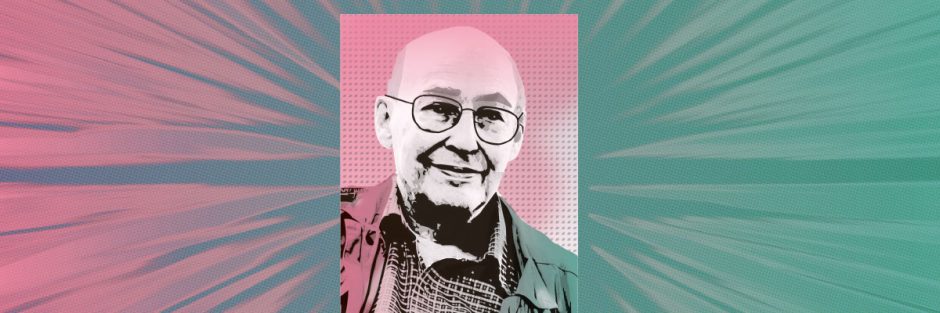
The brain functions like a machine, or so according to the theory of Marvin Minsky, one of the most important pioneers of artificial intelligence. In other words, it can be recreated – made immortal by backing up its consciousness onto a computer.
Could our entire life simply be a computer simulation, like “the Matrix” from the Hollywood blockbuster of the same name? According to Marvin Minsky, this is entirely conceivable: “It’s perfectly possible that we are the production of some very powerful complicated programs running on some big computer somewhere else. And there’s really no way to distinguish that from what we call reality.” Such thoughts were typical of the mathematician, cognition researcher, computer engineer and great pioneer of Artificial Intelligence. Minsky combined science and philosophy scarcely any other, questioned conventional views – but always with a strong sense of humour:
“No computer has ever been designed that is ever aware of what it’s doing; but most of the time, we aren’t either.”
Born in 1927 in New York, Minsky studied mathematics at Harvard University and received a PhD in mathematics from Princeton University. He was scarcely 20 years old when he began to take an interest in the topic of intelligence: “Genetics seemed to be pretty interesting because nobody knew yet how it worked,” recalled Minsky at the time in an article that appeared in the “New Yorker” in 1981. “But I wasn’t sure that it was profound. The problems of physics seemed profound and solvable. It might have been nice to do physics. But the problem of intelligence seemed hopelessly profound. I can’t remember considering anything else worth doing.”
Great intelligence is the sum of many non-intelligent parts
Back then, as a youthful scientist, he laid the foundation stone for a revolutionary theory, which he expanded on during his time at the Massachusetts Institute of Technology (MIT) and which finally led to him becoming a pioneer in Artificial Intelligence: Minsky held the view that the brain works like a machine and can therefore basically be replicated in a machine too. “The brain happens to be a meat machine,” according to one of his frequently quoted statements. “You can build a mind from many little parts, each mindless by itself.” Marvin Minsky was convinced that consciousness can be broken down into many small parts. His aim was to identify such components of the mind and understand them. Minsky’s view that the brain is built up from the interactions of many simple parts called “agents” is the basis of today’s neural networks.
Together with his Princeton colleague John McCarthy, he continued to develop the theory and gave the new scientific discipline a name at the Dartmouth Conference in 1956: Artificial Intelligence. Together McCarthy and Minsky founded the MIT Artificial Intelligence Laboratory some three years later – the world’s most important research centre for Artificial Intelligence ever since. Many of the ideas developed there were later seized on in Silicon Valley and translated into commercial applications.
Answerable for halting research
What is interesting is that the father of Artificial Intelligence was responsible for research into the area being halted for many years: Minsky had experimented himself with neural networks in the 1960s, but renounced them in his book “Perceptrons”. Together with his co-author Seymour Papert, he highlighted the limitations of these networks – and thus brought research into this area to a standstill for decades. Most of these limitations have since been overcome, and neural networks are a core technology for AI in the present day.
However, research into AI was by far not the only work area that occupied Marvin Minsky. His Artificial Intelligence Laboratory is regarded as the birthplace for the idea that digital information should be freely available – a theory from which open-source philosophy later emerged. The institute contributed to the development of the Internet, too. Minsky also had an interest in robotics, computer vision and microscopy – his inventions in this area are still used today.
Problems of mankind could be resolved
Minsky viewed current developments in AI quite critically, as he felt they were not focused enough on creating true intelligence. In contrast to the alarmist warnings of some experts that intelligent machines would take control in the not too-distant future, Minsky most recently advocated a more philosophical view of the future: machines that master real thinking could demonstrate ways to solve some of the most significant problems facing mankind. Death may also have been at the back of his mind in this respect: He predicted that people could make themselves immortal by transferring their consciousness from the brain onto chips. “We will be immortal in this sense,” according to Minsky. When a person grows old, they simply make a backup copy of their knowledge and experience on a computer. “I think, in 100 years, people will be able to do that.”
AI-Pioneer Minsky only temporarily dead
Marvin Minsky died in January 2016 at the age of 88. Although perhaps only temporarily: shortly before his death, he was one of the signatories of the Scientists’ Open Letter on Cryonics – the deep-freezing of human bodies at death for thawing at a future date when the technology exists to bring them back to life. He was also a member of the Scientific Advisory Board of cryonics company Alcor. It is therefore entirely possible that Minsky’s brain is waiting, shock-frozen to be brought back to life at some time in the future as a backup on a computer.
Faster to intelligent IoT products
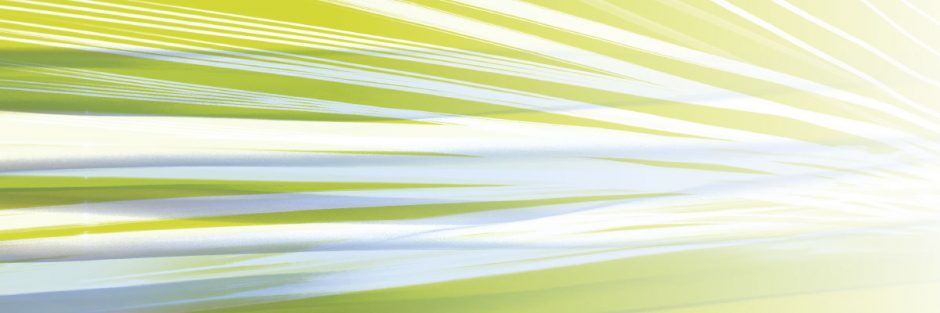
Developers working on products with integrated AI for the internet of things need time and major resources. This type of product typically requires up to 24 months until it is marketable. With a pre-industrialised software platform, Octonion now plans to reduce this time to only six months.
Smaller companies that want to realise products for the Internet of Things often lack the resources for electronics and software development. In addition, it takes lots of time to put together the building blocks required: connectivity, AI, sensor integration, etc. For example, the time to market for typical IoT projects is between 18 and 24 months – a very long time in the fast-paced world of the Internet.
A new solution from Octonion can help. The Swiss firm has developed a software platform for interconnecting objects and devices and equipping them with AI functions.
With this complete solution, IoT projects with integrated AI can be realised within six to eight months.
From the device to the cloud
Octonion provides a true end-to-end software solution, from an embedded device layer to cloud-based services. They include Gaia, a highly intelligent, autonomous software framework for decision-making that uses modern -machine-learning methods for pattern recognition. The system can be used for a wide range of applications in a variety of sectors. What’s more it guarantees that the data generated by the IoT device belongs to the customer only, who is also the sole project operator.
Reduce costs and development time
The result is a complete IoT system with Artificial Intelligence that provides a full solution from IoT devices or sensors and a gateway to the cloud. Since the individual platform levels are device-independent and compatible with all hosting solutions, customers can realise all their applications. Developers can select the functional module they require on each level. This enables them to adjust the platform to their individual requirements, providing the perfect conditions for developing and operating proprietary IoT solutions quickly and easily. With the Octonion platform, proprietary IoT solutions are marketable in only six months.
AI better than the doctor?
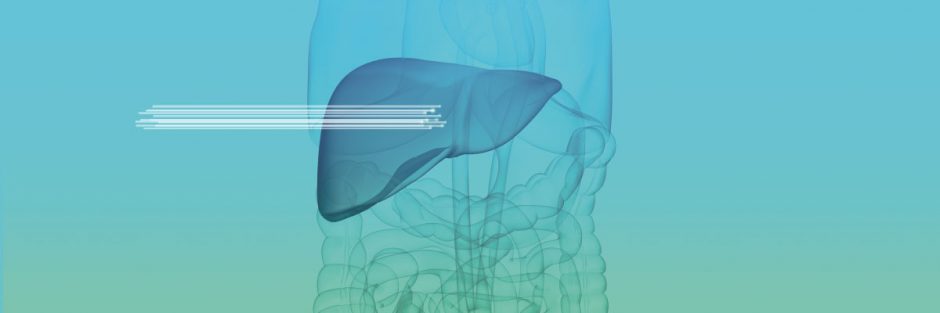
Cognitive computer assistants are helping clinicians to make diagnostic and therapeutic decisions. They evaluate medical data much faster, while delivering at least the same level of precision. It is hardly surprising, therefore, that, applications with Artificial Intelligence are being used more frequently.
Hospitals and doctors’ surgeries have to deal with huge volumes of data: X-ray images, test results, laboratory data, digital patient records, OR reports, and much more. To date, they have mostly been handled separately. But now the trend is towards bringing everything into a single unified software framework. This data integration is not only enabling faster processing of medical data and creating the basis for more efficient interworking between the various disciplines. It is also promising to deliver added value. New, self-learning computing algorithms will be able to detect hidden patterns in the data and provide clinicians with valuable assistance in their diagnostic and therapeutic decision-making.
Better diagnosis thanks to Artificial Intelligence: 30 times faster than a doctor with an error rate of 1%.
Source: PwC
Analysing tissue faster and more accurately
“Artificial Intelligence and robotics offer enormous benefits for our day-to-day work,” asserts Prof. Dr Michael Forsting, Director of the Diagnostic Radiology Clinic of the University Hospital in Essen. The clinic has used a self-learning algorithm to train a system in lung fibrosis. After just a few learning cycles, the computer was making better diagnoses than a doctor: “Artificial Intelligence is helping us to diagnose rare illnesses more effectively, for example. The reasons are that – unlike humans – computers do not forget what they have once learned, and they are better than the human eye at comparing patterns.”
Especially in the processing of image data, cognitive computer assistants are proving helpful in relieving clinicians of protracted, monotonous and recurring tasks, such as accurately tracing the outlines of an organ on a CT scan. The assistants are also capable of filtering information from medical image data that a clinician would struggle to identify on-screen.
Artificial Intelligence diagnosis – Better than the doctor
These systems are now even surpassing humans, as a study at the University of Nijmegen in the Netherlands demonstrates: the researchers assembled two groups to test the detection of cancerous tissue. One comprised 32 -developer teams using dedicated AI software solutions; the other comprised twelve pathologists. The AI developers were provided in advance with 270 CT scans, of which 110 indicated dangerous nodes and 160 showed healthy tissue. These were intended to aid them in training their systems. The result: the best AI system attained virtually 100 per cent detection accuracy and additionally colour-highlighted the critical locations. It was also much faster than a pathologist, who took 30 hours to detect the infected samples with corresponding precision. Most notably, the clinicians overlooked metastases less than 2 millimetres in size under time pressure. Only seven of the 32 AI systems were better than the pathologists, however.
The systems involved in the test are in fact not just research projects, but are already in use. In fibrosis research at the Charité hospital in Berlin, for example, where it is using the Cognitive Workbench from a company called ExB to automate the highly complex analysis of tissue samples for the early detection of pathological changes. The Cognitive Workbench is a proprietary, cloud-based platform which enables users to create and train their own AI-capable analyses of complex unstructured and structured data sources in text and image form. Ramin Assadollahi, CEO and Founder of ExB, states: “In addition to diagnosing hepatic fibrosis, we can bring our high-quality deep-learning processes to bear in the early detection of melanoma and colorectal cancers.”
Cost savings for the healthcare system
According to PwC, AI applications in breast cancer diagnoses mean that mammography results are analysed 30 times faster than by a clinician – with an error rate of just one per cent. There are prospects for huge progress, not only in diagnostics. In a pilot study, Artificial Intelligence was able to predict with greater than 70 per cent accuracy how a patient would respond to two conventional chemotherapy procedures. In view of the prevalence of breast cancer, the PwC survey reports that the use of AI could deliver huge cost savings for the healthcare system. It estimates that over the next 10 years, cumulative savings of EUR 74 billion might be made.
Digital assistants for patients
AI is also benefiting patients in very concrete ways to overcome a range of difficulties in their everyday lives, such as visual impairment, loss of hearing or motor diseases. The “Seeing AI” app, for example, helps the visually impaired to perceive their surroundings. The app recognises objects, people, text or even cash on a photo that the user takes on his or her smartphone. The AI-based algorithm identifies the content of the image and describes it in a sentence which is read out to the user. Other examples include smart devices such as the “Emma Watch”, which intelligently compensates for the tremors typical to Parkinson’s disease patients. Microsoft developer Haiyan Zhang developed the smart watch for graphic designer Emma Lawton, who herself suffers from Parkinson’s. More Parkinson’s patients will be provided with similar models in future.
Chips driving Artificial Intelligence
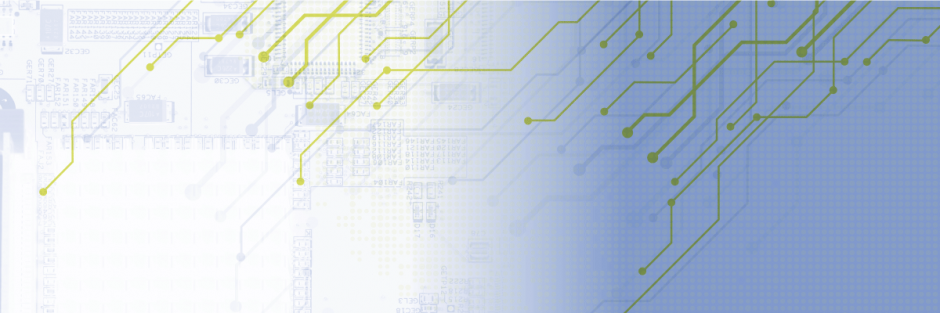
From the graphics processing unit through neuromorphic chips to the quantum computer – the development of Artificial Intelligence chips is supporting many new advances.
AI-supported applications must keep pace with rapidly growing data volumes and often have to respond simultaneously in real time. The classic CPUs that you will find in every computer quickly reach their limits in this area because they process tasks sequentially. Significant improvements in performance, particularly in the context of deep learning, would be possible if the individual processes could be executed in parallel.
Hardware for parallel computing processes
A few years, ago, the AI sector focused its attention on the graphics processing unit (GPU), a chip that had actually been developed for an entirely different purpose. It offers a massive parallel architecture, which can perform computing tasks in parallel using many smaller yet still efficient computer units. This is exactly what is required for deep learning. Manufacturers of graphics processing units are now building GPUs specifically for AI applications. A server with just one of these high-performance GPUs has a throughput 40 times greater than that of a dedicated CPU server.
However, even GPUs are now proving too slow for some AI companies. This in turn is having a significant impact on the semiconductor market. Traditional semiconductor manufacturers are now being joined by buyers and users of semiconductors – such as Microsoft, Amazon and even Google – who are themselves becoming semiconductor manufacturers (along with companies who want to produce chips to their own specifications). For example, Alphabet, the parent company behind Google, has developed its own Application-Specific Integrated Circuit (ASIC), which is specifically tailored to the requirements of machine learning. The second generation of this tensor processing unit (TPU) from Alphabet offers 180 teraflops of processing power, while the latest GPU from Nvidia offers 120 teraflops. Flops (Floating Point Operations Per Second) indicate how many simple mathematical calculations, such as addition or multiplication, a computer can perform per second.
Different performance requirements
Flops are not the only benchmark for the processing power of a chip. With AI processors, a distinction is made between performance in the training phase, which requires parallel computing processes, and performance in the application phase, which involves putting what has been learned into practice – known as inference. Here the focus is on deducing new knowledge from an existing database through inference. “In contrast to the massively parallel training component of AI that occurs in the data centre, inferencing is generally a sequential calculation that we believe will be mostly conducted on edge devices such as smartphones and Internet of Things, or IoT, products,” says Abhinav Davuluri, analyst at Morningstar, a leading provider of independent investment research. Unlike cloud computing, edge computing involves decentralised data processing at the “edge” of the network. AI technologies are playing an increasingly important role here, as intelligent edge devices such as robots or autonomous vehicles do not have to transfer data to the cloud before analysis. Instead, they can acquire the data directly on site – saving the time and energy required for transferring data to the data centre and back again.
Solutions for edge computing
For these edge computing applications, another new chip variant – Field-Programmable Gate Array (FPGA) – is currently establishing itself alongside CPUs, GPUs and ASICs. This is an integrated circuit, into which a logical circuit can be loaded after manufacturing. Unlike processors, FPGAs are truly parallel in nature thanks to their multiple programmable logic blocks, which mean that different processing operations are not assigned to the same resource. Each individual processing task is assigned to a dedicated area on a chip and can thus be performed autonomously. Although they do not quite match the processing power of a GPU in the training process, they rank higher than graphics processing units when it comes to inference. Above all, they consume less energy than GPUs, which is particularly important for applications on small, mobile devices. Tests have shown that FPGAs can detect more frames per second and watt than GPUs or CPUs, for example. “We think FPGAs offer the most promise for inference, as they can be upgraded while in the field and could provide low latencies if located at the edge alongside a CPU,” says Morningstar analyst Davuluri.
More start-ups are developing Artificial Intelligence chips
More and more company founders – and investors – are recognising the opportunities offered by AI chips. At least 45 start-ups are currently working on corresponding semiconductor solutions, while at least five of these have received more than USD 100 million from investors. According to market researchers at CB Insights, venture capitalists invested more than USD 1.5 billion in chip start-ups in 2017 – double the amount that was invested just two years ago. British firm Graphcore has developed the Intelligence Processing Unit (IPU), a new technology for accelerating machine learning and Artificial Intelligence (AI) applications. The AI platform of US company Mythic performs hybrid digital/analogue calculations in flash arrays. The inference phase can therefore take place directly within the memory, where the “knowledge” of the neural network is stored, offering benefits in terms of performance and accuracy. China is one of the most active countries when it comes to Artificial Intelligence chip start-ups. The value of Cambricon Technologies alone is currently estimated at USD 1 billion. The start-up has developed a neural network processor chip for smartphones, for instance.
New chip architectures for even better performance of Artificial Intelligence
Neuromorphic chips are emerging as the next phase in chip development. Their architecture mimics the way the human brain works in terms of learning and comprehension. A key feature of these chips is the removal of the separation between the processor unit and the data memory. Launched in 2017, neuromorphic test chips with over 100,000 neurons and 100 million plus synapses can unite training and inference on one chip. When in use, they should be able to learn autonomously at a rate that is a 1 million times better than the third generation of neural networks. At the same time, they are highly energy-efficient.
Quantum computers represent a quantum leap for AI systems in the truest sense of the word. The big players in the IT sector, such as Google, IBM and Microsoft, as well as countries, intelligence services and even car manufacturers are investing in this technology. These computers are based on the principles of quantum mechanics. A quantum computer can perform each calculation step for all states at the same time. This means that it delivers exceptional processing power for the parallel processing of commands and has the potential to compute at a much higher speed than conventional computers. Although the technology may still be in its infancy, the race for faster and more reliable quantum processors is already well underway.
Ethics and principles of AI
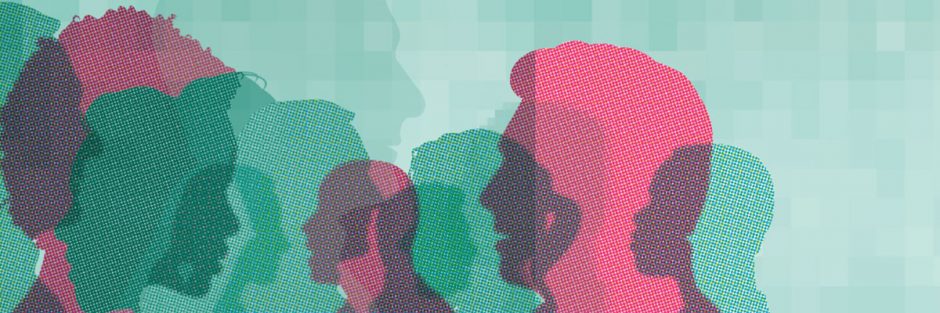
Artificial intelligence is only as good the data it is based on. Unless it takes all factors and all population groups into account, faulty and biased decisions may come as a result. But how about ethics and principles of Artificial Intelligence in recent applications?
The field of Artificial Intelligence is developing rapidly, and promises to help address some of the biggest challenges we face as a society,” says Kate Crawford, Co-Founder of the AI Now Institute: “But we urgently need more research into the real-world implications of the adoption of AI in our most sensitive social institutions. People are already being affected by these systems, be it while at school, looking for a job, reading news online, or interacting with the courts.” It is for this very reason that the AI Now Institute was launched in late 2017 at New York University. This is the first university research institute dedicated to the social impact of Artificial Intelligence. To this end, it wants to expand the scope of AI research to include experts from fields such as law, healthcare, occupational and social sciences. According to Meredith Whittaker, another Co-Founder of AI Now, “AI will require a much wider range of expertise than simply technical training. Just as you wouldn’t trust a judge to build a deep neural network, we should stop assuming that an engineering degree is sufficient to make complex decisions in domains like criminal justice. We need experts at the table from fields like law, healthcare, education, economics, and beyond.”
Safe and just AI requires a much broader spectrum of expertise than mere technical know-how.
AI Systems with Prejudices are a Reality
“We’re at a major inflection point in the development and implementation of AI systems,” Kate Crawford states. “If not managed properly, these systems could also have far-reaching social consequences that may be hard to foresee and difficult to reverse. We simply can’t afford to wait and see how AI will affect different populations.” With this in mind, the AI Now Institute is looking to develop methods to measure and understand the impacts of AI on society.
It is already apparent today that unsophisticated or biased AI systems are very real and have consequences – as shown, in one instance, by a team of journalists and technicians at Propublica, a non-profit newsdesk for investigative journalism. They tested an algorithm which is used by courts and law enforcement agencies in the United States to predict repeat offending among criminals. They found that it was measurably biased against African Americans. Such prejudice-laden decisions come about when the data that the AI is based on and works with is not neutral. If it includes social disparities, for instance, the evaluation is also skewed. If, for example, only data for men is used as the basis for an analysis process, women may be put at a disadvantage.
It is also dangerous if the AI systems have not been taught all the relevant criteria. For instance, the Medical Center at the University of Pittsburgh noted that a major risk factor for severe complications was missing from an AI system for initially assessing pneumonia patients. And there are many other relevant areas in which AI systems are currently in use without having been put through testing and evaluation for bias and inaccuracy.
Checks Needed
As a result of this, the AI Now Institute took to its 2017 research report to call for all important public institutions to immediately stop using “black-box” AI. “When we talk about the risks involved with AI, there is a tendency to focus on the distant future,” says Meredith Whittaker: “But these systems are already being rolled out in critical institutions. We’re truly worried that the examples uncovered so far are just the tip of the iceberg. It’s imperative that we stop using black-box algorithms in core institutions until we have methods for ensuring basic safety and fairness.”
Autonomous driving thanks to AI
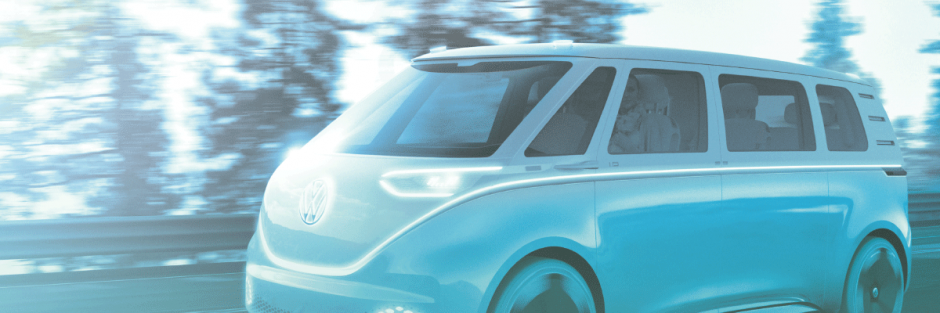
In just a few years, every new vehicle will be fitted with electronic driving assistants. They will process information from both inside the car and from its surrounding environment to control comfort and assistance systems.
We are teaching cars to negotiate road traffic autonomously,” reports Dr Volkmar Denner, Chairman of the Board of Bosch. “Automated driving makes the roads safer. Artificial Intelligence is the key. Cars are becoming smart,” he asserts. As part of those efforts, the company is currently developing an on-board vehicle computer featuring AI. It will enable self-driving cars to find their way around even complex environments, including traffic scenarios that are new to it.
Transferring knowledge by updates
The on-board AI computer knows what pedestrians and cyclists look like. In addition to this so-called object recognition, AI also helps self-driving vehicles to assess the situation around them. They know, for example, that an indicating car is more likely to be changing lane than one that is not indicating. This means self-driving cars with AI can detect and assess complex traffic scenarios, such as a vehicle ahead turning, and apply the information to adapt their own driving. The computer stores the knowledge gathered while driving in artificial neuronal networks. Experts then check the accuracy of the knowledge in the laboratory. Following further testing on the road, the artificially created knowledge structures can be downloaded to any number of other on-board AI computers by means of updates.
Assistants recognising speech, gestures and faces
Bosch is also intending to collaborate with US technology company Nvidia on the design of the central vehicle computer. Nvidia will supply Bosch with a chip holding the algorithms for the vehicle’s movement created through machine learning. As Nvidia founder Jensen Huang points out, on-board AI in cars will not only be used for automated driving: “In just a few years, every new vehicle will have AI assistants for speech, gesture and facial recognition, or augmented reality.” In fact, the chip manufacturer has also been working with Volkswagen on the development of an intelligent driving assistant for the electric microvan I.D.Buzz. It will process sensor data from both inside the car and from its surrounding environment to control comfort and assistance systems. These systems will be able to accumulate new capabilities in the course of further developments in autonomous driving. Thanks to deep learning, the car of the future will learn to assess situations precisely and analyse the behaviour of other road users.
3D recognition using 2D cameras
Key to automated driving is creating the most exact map possible of the surrounding environment. The latest camera systems are also using AI to do that. A project team at Audi Electronics Venture, for example, has developed a mono camera which uses AI to generate a high-precision three-dimensional model of the surroundings. The sensor is a standard, commercially available front-end -camera. It captures the area in front of the car to an angle of about 120 degrees, taking 15 frames per second at a 1.3 megapixel resolution. The images are then processed in a neuronal network. That is also where the so-called semantic segmentation takes place. In this process, each pixel is assigned one of 13 object classes. As a result, the system is able to recognise and distinguish other cars, trucks, buildings, road markings, people and traffic signs. The system also uses neuronal networks to gather distance information. This is visualised by so-called ISO lines – virtual delimiters which define a constant distance. This combination of semantic segmentation and depth perception creates a precise 3D model of the real environment. The neuronal network is pre-trained through so-called unsupervised learning, having been fed with large numbers of videos of road scenarios captured by a stereo camera. The network subsequently learned autonomously to understand the rules by which it generates 3D data from the mono camera’s images.
Mitsubishi Electric has also developed a camera system that uses AI. It will warn drivers of future mirrorless vehicles of potential hazards and help avoid accidents, especially when changing lane. The system uses a new computing model for visual recognition that copies human vision. It does not capture a detailed view of the scene as a whole, but instead focuses rapidly on specific areas of interest within the field of vision. The relatively simple visual recognition algorithms used by the AI conserve the system resources of the on-board computer. The system is nevertheless able to distinguish between object types such as pedestrians, cars and motorcycles. Compared to conventional camera-based systems, the technology will be able to significantly extend the maximum object recognition range from the current approximately 30 metres to 100 metres. It will also be able to improve the accuracy of object recognition from 14 percent to 81 per cent.
AI is becoming a competitive factor
As intelligent assistance systems are being implemented ever more frequently, AI is becoming a key competitive factor for car manufacturers. That is true with regard to the use of AI for autonomous driving as well as in the development of state-of-the-art mobility concepts based on AI. According to McKinsey, almost 70 per cent of customers are already willing to switch manufacturer today in order to gain better assisted and autonomous driving features. The advice from Dominik Wee, Partner at McKinsey’s Munich office, is: “Premium manufacturers, in particular, need to demonstrate to their highly demanding customers that they are technology leaders in AI-based applications as in other areas – for example in voice-based interaction with the vehicle, or in finding a parking space.”
(Picture Credit: Volkswagen AG)
Opinions on Artificial Intelligence
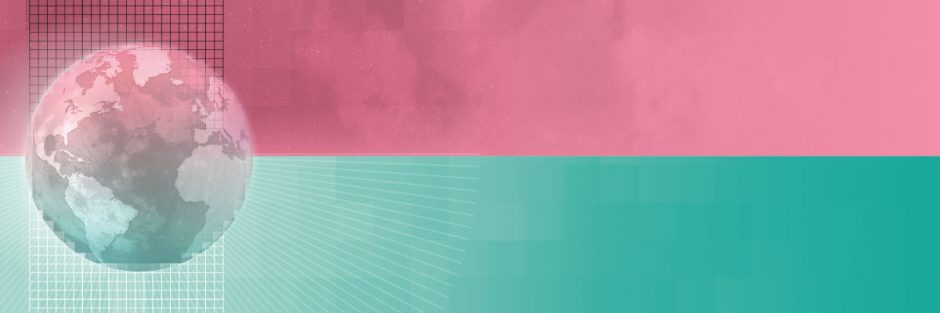
What will artificial intelligence mean for the human race and for human life? It is a question vehemently argued over by scientists and businesspeople. A small cross-section of opinion from a few pessimists, optimists and realists alike.
“AI is a fundamental existential risk for human civilisation, and I don’t think people fully appreciate that.”
Elon Musk, Entrepreneur and Investor
“The AI, big data is a threat to human beings. The AI and robots are going to kill a lot of jobs, because in the future, these will be done by machines.”
Jack Ma, CEO and Founder, Alibaba
“I fear that AI may replace humans altogether. If people design computer viruses, someone will design AI that improves and replicates itself. This will be a new form of life that outperforms humans.”
Stephen Hawking, Physicist
“One problem is that the term ‘killer robots’ makes people think of ‘Terminator’ which is still 50, 100 or more years away. But it is much simpler technologies, stupid AI and not smart AI that we need to worry about in the near future.”
Prof. Toby Walsh, University of New South Wales
“We live in a world that could become fraught with day-to-day hazards from the misuse of AI and we need to take ownership of the problems – because the risks are real.”
Dr Seán Ó hÉigeartaigh, Executive Director of Cambridge University’s Centre for the Study of Existential Risk
“It is time for an optimistic antidote to the doom and gloom we often hear regarding AI and the future of work. There is no passive forecast for the future of work – it will be what we make it, and that begins with imagination of what we want it to be.”
Martin Reeves, Director, BCG Henderson Institute
“AI is probably the most important thing humanity has ever worked on. I think of it as something more profound than electricity or fire. Artificial Intelligence will save us not destroy us.”
Sundar Pichai, CEO, Google
“Regulating AI can wait until we invent it. It’s not true that it will be too late by the time we create it. We have switches. They work.”
Reza Zadeh, CEO and Founder, Matroid
“If we want to close the demographic gap, we cannot leave anybody out. Robots, intelligent machines and software systems help people to work more productively.”
Dieter Spath, Co-Chair of Plattform Lernende Systeme, a digitalisation research venture launched by the German government
“AI promises to deliver benefits not only economically, but also in terms of business management: it enables employees to leave repetitive or hazardous tasks to computers and robots, and focus themselves on value-adding and interesting work.”
Harald Bauer, Senior Partner at McKinsey’s Frankfurt office
“Artificial Intelligence will change the way we live together, beyond a doubt. For our society, it is a case of welcoming the positive developments while preventing negative impacts.”
Hannes Schwaderer, President of Initiative D21, a network promoting the information society in Germany
“We must do everything we can to take advantage of AI’s potential for the digital society. It is not about an AI system coming to replace a police officer or a doctor. It is about intelligent systems doing work for them.”
Achim Berg, President of Bitkom, the German IT association
“AI is making advances in every aspect of our lives, such as health and well-being, transportation and education. However, there are also pressing concerns over the technology’s safety, trustworthiness, fairness and transparency that need to be addressed.”
Prof. Tony F. Chan, President, Hong Kong University of Science and Technology
Forms of Artificial Intelligence
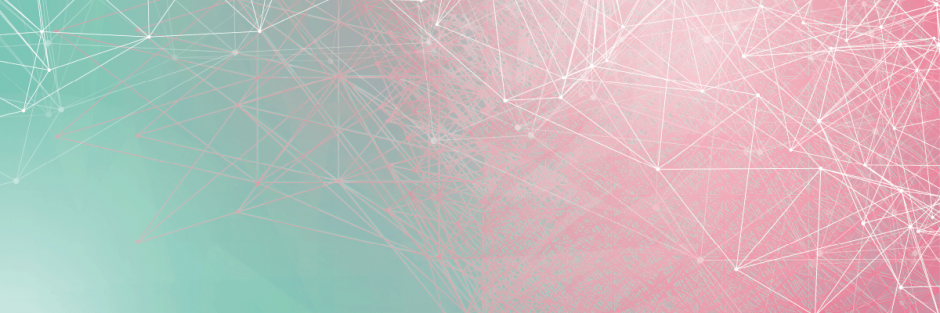
Despite the performance capabilities that many AI systems already have today, they are still classed as “weak”. Only when they are no longer designed to perform just a single specific task are they classed
as “strong AI”.
Artificial Intelligence is currently a real buzzword, even – or especially – beyond the high-tech industries. But all AI is not the same. Experts classify Artificial Intelligence in three different forms:
- Weak Artificial Intelligence
- Strong Artificial Intelligence
- Artificial Supertintelligence
First, there is weak AI (also known as narrow AI). This type of Artificial Intelligence is only able to perform a single, specific, clearly defined task. It employs mathematical and computer science methodology; its approach to problem-solving is not varied, and it gains no deeper understanding of the problem at hand. This means weak AI is quite capable of performing tasks better than a human, but it can’t be used to solve any problems other than the original defined task. Familiar examples of weak AI are Siri, some robots used in industrial manufacturing, and autonomous vehicles.
Same intellectual abilities as a human
When most people think of AI, they think of machines that are more intelligent than humans and can do anything a human can. That type of Artificial Intelligence is termed strong AI (also known as general AI). Machines with strong AI can attain the same intellectual abilities as a human or even surpass them. AI of this type is able to solve more than just one specific problem, act on its own initiative, plan, learn, and communicate in natural language. It has not yet been possible to develop strong AI to date, however. But scientists do consider it a realistic prospect within the next 20 to 40 years.
When machines become more intelligent than their creators
The third category of AI is Artificial Superintelligence (ASI). It will have been attained when machines surpass the intelligence of the most intelligent minds in human history. The fear is that a stage will then have been reached where humans are no longer the dominant species. Super-intelligent machines would be capable of building still better machines; AI would advance explosively, leaving human intelligence far behind. This scenario has become known as the “technological singularity”. Although it was first coined back in the 1960s, the term became particularly popular in 1998 through a book titled “The Singularity is Near” by Raymond Kurzweil. In his book, Kurzweil predicts that the technological singularity will be reached in the year 2045. That is when he estimates that the computing power of AI will have surpassed the intelligence of all of humanity by a factor of a billion. Different time-scales have repeatedly been mooted since Kurzweil’s book appeared, however. There is currently no sign of Artificial Superintelligence on the horizon. Yet we should not forget that computing power is advancing at a rapid rate; the performance of computer chips has doubled every 18 months in the past. So computers are advancing much more rapidly than human consciousness. Whereas humans have taken millennia to develop, computers have done so in less than 100 years. So, it seems only a matter of time before Artificial Superintelligence arrives…
The benefit of Artificial Intelligence
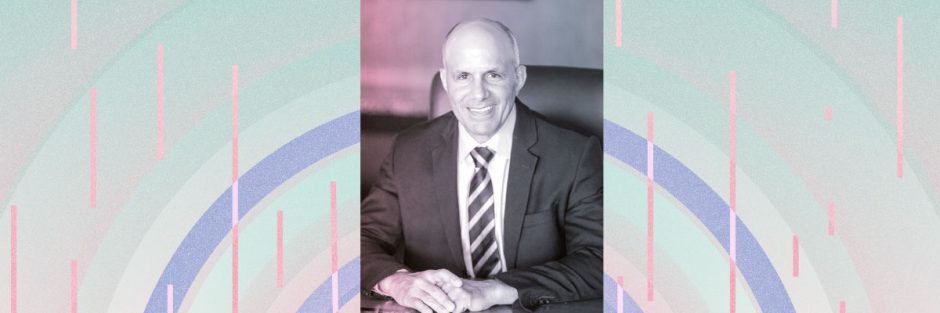
The technology landscape is changing ever more rapidly; development lead times for new products are getting shorter, as are the intervals at which innovations are coming onto the market. These are trends to which an electronics distributor especially needs to respond, in the view of William Amelio, CEO of Avnet, the parent company of EBV. So Amelio, Avnet’s CEO since the summer of 2016, has launched an extensive programme to transform the whole business. It incorporates some 450 individual projects aimed at enhancing relationships with customers, helping to meet their needs even more closely than before, and offering them even more services. “We are the first electronics distributor to offer genuine end-to-end solutions, carrying a product idea from the initial prototypes through to mass production. In order to achieve that, we had to make significant changes to our business,” says Amelio. His goal is to transform Avnet into an “agent of innovation”. Artificial Intelligence is a key component in those efforts – as a market segment, but above all for the company itself, as Amelio explains in the interview.
What influence do you think Artificial Intelligence (AI) will have in the future on technology and our lives in general?
William Amelio: Futurists are saying that AI will have a bigger impact on society than the industrial and digital evolutions. We’re starting to see more concrete examples of what that might be like as the technologies needed to power AI systems are now becoming more affordable and open-sourced. Artificial Intelligence is already enabling more personalised medicine and treatment plans in healthcare. The vehicles on our roads are increasingly autonomous. Facial, voice and fingerprint recognition are becoming more commonplace as well. Nonetheless, AI is providing input to us and we’re still making the ultimate decision. Over time, these applications will be equipped to make more decisions on our behalf, in theory helping us devote more time to higher-level thinking.
As Artificial Intelligence evolves to other applications over the next few years, it will begin to have exponential impact, especially in areas such as employment. Enabling farmers to maximise the efficiency of their fields or automating repetitive office management tasks will dramatically influence how we manage our work. In turn, AI will create new public policy challenges. Though opinions vary on the scale and timing of its impact, AI does have the capability and potential to help us solve many of the complex problems that plague our society.
What role will AI technology play specifically for Avnet as an electronics distributor?
W. A.: AI will help us optimise our operations, increase customer and employee satisfaction and unearth new market opportunities. Today, we’re exploring using database models for personalised pricing, automating payments and both managing and anticipating customer needs. We’re piloting a project to automate repetitive tasks that don’t add value to our bottom line or to our employees’ happiness. AI can also help us make predictions and deliver more personalised offerings and services to customers, suppliers and to our employee base.
We’ll also be able to speed up and automate processes by offloading some decisions to machines. In particular, I think supply chains will go through a complete metamorphosis resulting from a combination of emerging technologies, including AI. Much of AI’s early promise comes down to better decision-making. This is also the area where AI will begin to significantly impact corporate leadership and culture.
“To get there, we’ll need to shape a new generation of leaders who understand how to work with AI.”
What influence is AI technology having on your products?
W. A.: AI is certainly beginning to create demand for new technologies, which is opening the door for new market opportunities. For example, our new Avnet MiniZed Zync SoC platform improves the performance of sound capture for AI applications. It leverages technologies from Xilinx and Aaware to provide faster, safer far-field voice interfaces. Among our major suppliers, we’re seeing AI drive both hardware and software products, including both FPGA kits and custom chips to hardcode AI neural networks. Many companies are also designing AI-specific chips. This reflects a larger trend of moving some intelligence to the edge instead of housing it all in the cloud, which solves latency and cost issues with the magnitude of processing power that these applications require. Not only are venture capitalists backing start-ups in this area, but large technology powerhouses such as Intel, Google, Apple and Microsoft are getting in on custom chips, too. Many of them are already on our linecard.
Will AI bring any other changes beyond that for Avnet?
W. A.: I mentioned earlier that AI will significantly impact corporate culture and leadership. This is because it will change how we work, how we make decisions and how we structure our business models. To get there, we’ll need to shape a new generation of leaders who understand how to work with AI. This means introducing a new set of skills and evolving some of our existing ones to truly understand the new advantages that AI introduces.
For example, AI systems can help anticipate employee satisfaction and balance workloads accordingly. We can also gain insight from customer surveys more quickly and regularly because we won’t need to go through such laborious data mining. But are the corporate systems and talent needed to enable HR and customer service departments to operate this way available today? Probably not.
AI can do a lot for us, but first we need to learn how to work with it. The way we do business is going to look very different in 10 years, and each of us is going to need to embark on a personal journey of change and continuous learning along the way.
Avnet is now using AI itself – on its “Ask Avnet” platform. Can you tell us briefly what “Ask Avnet” is and how your customers benefit from it?
W. A.: Ask Avnet is designed to deliver a better customer experience by leveraging a combination of AI and human expertise to create an intelligent assistant. Our customers benefit because it can help them address a wide variety of questions without having to jump through customer service hoops. Ask Avnet can move customers through our various digital properties seamlessly, too. Customers can still enjoy the same experience on each site while Ask Avnet keeps them connected to the resources available within our broader ecosystem. We’re already seeing promising results, such as reduced errors. As Ask Avnet learns over time, it will help us deliver a more scalable, efficient and pleasant customer experience.
More importantly, Ask Avnet is designed to understand the context of our customers’ needs and tailor its responses accordingly. It makes personalisation possible, and this adds significant value that will only grow with time. Because it can contextually understand which stage of the product development journey our customers are in, Ask Avnet can proactively identify information that they might need but are not necessarily on the lookout for, such as product maturity stage or anticipated lead time. It continuously learns from new queries and experiences over time, continually delivering the latest insights as needs, technologies and markets evolve.
“AI does have the capability and potential to help us solve many of the complex problems that plague our society.”
“Ask Avnet” also utilises the know-how of the hackster.io and element14 platforms. How important are those 2016 acquisitions to the “Ask Avnet” objective of shortening time to market for your customers?
W. A.: Ask Avnet is another way for customers to access the wealth of information available through the Avnet ecosystem, of which these communities are one important piece. Ultimately, it extends the mission of our communities while introducing more proactivity and personalisation. When you’re in our communities, you’re on an exploratory journey. When you’re using Ask Avnet, you have an AI-powered guide that brings you the information you need.
By combining Ask Avnet with our online communities, we’re helping shorten our customers’ time to market by making the resources they need to solve their challenges more readily available, easy to access and relevant.
The beta version of “Ask Avnet” went online in July 2017. What has been your customers’ experience with it so far?
W. A.: The customer experience continues to improve because the intelligent assistant learns from every query. Customers are finding greater value in the tool, as both usage and customer satisfaction are increasing. It’s able to hold more detailed and longer conversations as the kinds of questions that Ask Avnet is able to address have expanded significantly. It’s also now able to probe further into queries.
For example, at launch, Ask Avnet would respond to a query with a list of recommendations. Today, Ask Avnet would respond with more relevant questions to help clarify your specs and narrow down options before providing recommendations. It can also include contextually relevant information, such as how-to projects from our communities, price and stock information or lead times. As it learns, Ask Avnet is providing more information and holding more high-quality conversations.
Will there be more projects with Avnet processes using AI in the future?
W. A.: Without a doubt. We’re currently focused on those that create the highest possible value for stakeholders, including both front-office and back-office projects. We’re looking at demand management, supply chain optimisation and are continuing work to enhance our customers’ experience with Ask Avnet and other projects. The technology is really applicable anywhere where there’s an opportunity to improve efficiency, reduce boredom and help our employees create more value.
How will AI influence our lives in the future?
W. A.: Just when you think innovation is waning, a new trend like AI takes hold. It’s clear to me that the economic and social value AI has to offer is just at the beginning of its “S-curve”. Whichever argument sways you, we all can agree that AI is fundamentally going to change the nature of how we live and work. This means that we need to explore new business models, hiring practices and skill sets. Start-ups, makers, tech giants and oldline companies are all in the game. Competition will drive new and innovative AI ideas and applications, and I’m excited to see the next chapter in this story.
From initial sketch to mass production
Avnet supports its customers through every phase of the product life cycle – from initial idea to design, from prototype to production. As one of the world’s largest distributors of electronic components and embedded solutions, the company offers a comprehensive portfolio of design and supply chain services in addition to electronic building blocks. Its acquisition of the online communities Hackster.io and Element14 in 2016 furthermore shows how Avnet is building bridges between the maker and manufacturer. Hackster.io is committed to helping fledgling companies develop hardware for IoT designs. The network engages with some 90 technology partners and includes close to 200,000 engineers, makers and hobbyists. Element14 is an engineering community with more than 430,000 members. By acquiring both platforms, the company is taking an important step towards achieving its goal of helping customers get their ideas first to market. In this respect, Avnet can call on a closely-knit global network of leading technology companies dating back almost a century.
Avnet has its headquarters in Phoenix, Arizona. The company was founded in 1921 by Charles Avnet, starting out as a small retail store in Manhattan specialising in the sale of components for radios. Today Avnet has a workforce of more than 15,000 employees and is represented in 125 countries in North America, Europe and Asia.
Pattern recognition trough AI
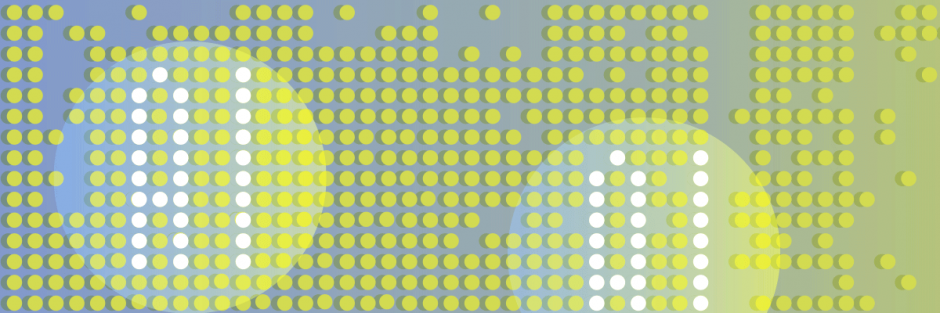
One of the greatest strengths of Artificial Intelligence systems is their ability to find rules or patterns in big data, pictures, sounds and much more.
Many functions of intelligent information systems are based on methods of pattern recognition: support for diagnoses in the area of medicine, voice recognition with assistance systems and translation tools, object detection in camera images and videos or also forecasting of stock prices. All of these applications involve identifying patterns – or rules – in large volumes of data. It is immaterial whether this data relates to information stored in a database or to pixels in an image or the operating data of a machine. Such identification of patterns was either not possible at all with classic computer systems or required lengthy calculation times of up to several days.
Classifying data in seconds
Developments in the area of neural networks and machine learning have led to the emergence of solutions today in which even complex input data can be matched and classified within minutes or even seconds with trained features. A distinction is made here between two fundamental methods: supervised and unsupervised classification.
With supervised classification of input data in pattern recognition, the system is “fed” training data, with the data with the correct result being labelled accordingly. The correct response must therefore be available during the training phase and the pattern recognition algorithm has to fill the gap between input and output. This form of supervised pattern recognition is used with machine vision for object detection or for facial recognition for example.
In the case of unsupervised learning, the training data is not labelled, which means that the possible results are not known. The pattern-recognition algorithm therefore cannot be trained by providing it with the results it is to arrive at. Algorithms are used more so, which explore the structure of the data and derive meaningful information from it. To stay with the example of machine vision: the techniques of unsupervised pattern recognition are used for object detection, among other things. Unsupervised methods are essentially also used for data mining, thus for detecting contents in large data volumes based on visibly emerging structures.
Finding structures in big data
A number of different methods are in turn used in this type of big data analysis. One such example is association pattern analysis. A set of training data is searched through in this case for combinations of individual facts or events, which occur significantly often or significantly rarely together in the data. Another example in this context is what is known as sequential pattern mining. A set of training data is searched through to identify time-ordered sequences that occur conspicuously often or rarely in succession in the data. The result of the different mining methods is a collection of patterns or rules, which can be applied to future data sets to discover whether one or more rules occur in these data sets. The rules can be integrated in operative software programs in order to develop early warning concepts, for example, or to predict when maintenance is due.
Opportunities of Artificial Intelligence
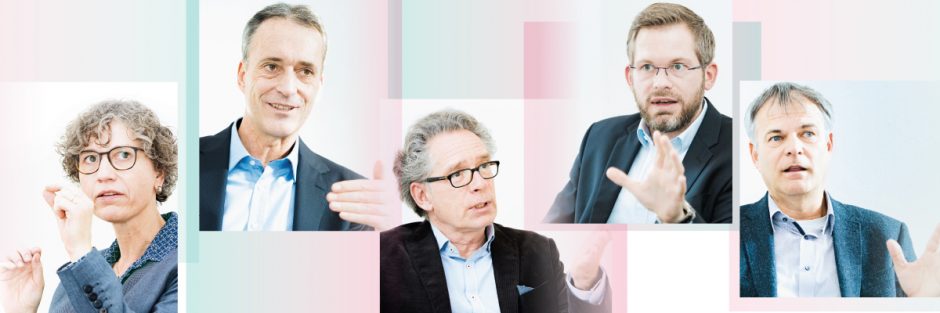
The participants in our expert discussion see a great need to inform people in particular about the opportunities and possibilities of Artificial Intelligence. Even if issues such as ethics and bias indeed pose a challenge – no one is worried about a super-intelligence that would replace human beings.
The image that people have of Artificial Intelligence is quite distorted. “On the one hand, the expectations placed on the capabilities of AI are huge; on the other hand, there is also the fear that super-smart Artificial Intelligence will take over and rule the world,” says Andrea Martin, Chief Technology Officer of IBM for the DACH region. Hollywood has especially shaped this image of a future ruled by machines, with blockbusters such as the “Terminator” series of films. However, statements by renowned scientists, such as Stephen Hawking, have also perturbed people: “When I hear that AI is going to kill us at some point, I just shake my head in dismay,” declares René Büst, Director of Technology Research at Arago. “Assertions such as that only serve to frighten people. And that makes it extremely difficult for us, as providers, to make clear just what AI is capable of and what not.” In this regard, the round-table participants also view the media as being obligated to not only report on horror scenarios in conjunction with Artificial Intelligence but also its opportunities and possibilities. “Unfortunately, though, my impression is that the press would rather focus on sensational doomsday theories because they simply attract more reader attention,” states Reinhard Karger, Spokesman for the German Research Centre for Artificial Intelligence (DFKI).
Yet, on the other hand, the providers of systems are also to blame for the distorted public image of AI: “With high marketing effort and expense, systems are being launched onto the market that are actually rather trivial,” says Thomas Staudinger, Vice President of Marketing at EBV. This creates expectations which many systems ultimately cannot fulfil.
“We should look at the opportunities and possibilities that Artificial Intelligence offers to us.”
Andrea Martin, Chief Technology Officer IBM DACH
More applications than assumed
A typical example is chatbots: many users expect one could conduct a conversation with the digital assistants as with a human. “We will probably not even be able to do this in the long term,” says René Büst. “We should instead try to start with smaller tasks, such as the automation of processes.” Andrea Martin also agrees: “Personalised interaction is only one aspect of Artificial Intelligence. In addition, we should see how AI can help us to gain new insights from enormous amounts of data and thereby assist us in making better decisions.”
The experts can list a whole range of applications where AI is already being used today, delivering real benefits to users: whether it’s predictive maintenance, the organisation of work, in medical research, or in many other areas where AI systems support people. In fact, many more AI solutions have already been deployed than most people realise. Oliver Gürtler, Senior Director Business Group Cloud & Enterprise, Microsoft Germany, makes this very clear: “As of today, 760,000 developers at our partners are already developing solutions that take advantage of AI. And this is only on our platform – there are, of course, other vendors.” This fits in with the figures which Andrea Martin cites: “In 2018, some 70 per cent of all developers will, in one way or another, be integrating Artificial Intelligence capabilities into their products.”
“The decisions made with AI must be transparent and reproducible.”
Oliver Gürtler, Senior Director Business Group Cloud + Enterprise, Microsoft Germany
Various technologies work together
This rapid progress is made possible through the interaction of various technologies, as René Büst explains: “With cloud computing, big data, analytics services and GPUs, the foundation was laid for today’s AI solutions over the past ten years.” Above all else, DFKI Spokesman Karger sees the possibility of massive parallel processing of graphics data, in order to compute neural networks, as having enabled a major breakthrough for AI: “Today, we finally have a supercomputer which we can, for example, integrate into a car in order to process sensor data in real time.” In addition to the possibility of providing computing power to an application via the processors, Thomas Staudinger sees a further building block: “Thanks to the developments in the field of semiconductor technology, the sensors have become so inexpensive that they can be integrated in applications on a wide scale.” Consequently, the data volumes required for AI solutions can be generated. “Data is the crude oil for Artificial Intelligence,” emphasises Oliver Gürtler. “In order to process it, for one thing, databases that can be accessed in milliseconds are required. For another, processing is done much more directly at the front end – on the devices. I also need connectivity to exchange data between devices and data centres.” The development will continue, as the round-table participants emphasised. Among other things, they cited mesh computing, in which end-user devices communicate directly with each other without an Internet connection, or quantum computers – with which initial testing has already been carried out.
“I see AI as a companion technology that helps us to more easily determine our lives.”
René Büst, Director of Technology Research, Arago
Secure AI systems
“Technology development has accelerated exponentially. A major challenge in this range of topics is transparency,” says Oliver Gürtler. If you don’t know why an AI application has made a certain decision, then the results could be easily manipulated without the user’s knowledge. This also means that the results must be reproducible. And, of course, that the data is protected to prevent tampering. “If there is no transparency, i.e. it is not apparent just how AI works at all, then the user has to accept the results without questioning.” The security of AI systems is based on many pillars, as Andrea Martin explains: “Security must be ensured in the hardware, in the software and in the connectivity.
And, then there is a further pillar – human beings. If we ignore people, then we have neglected the most important factor.” Reinhard Karger also views humans, or more specifically the lack of security awareness on the part of users, as being a major risk factor. “In all of the discussions regarding data protection and security, the focus is always on putting better locks on the doors – while the windows are wide open.”
“There is a plurality of assistant systems, but no singularity of AI.”
Reinhard Karger, Company Spokesman of the German Research Centre for Artificial Intelligence
The challenge of bias
Whereas data protection and data security are already familiar issues from many other areas, AI solutions pose an additional aspect: personal bias. “If facial recognition is developed by a team which, for example, consists of a group of white men, it could happen that the system returns incorrect results for people who do not have white skin colour,” explains Oliver Gürtler as an example. “This can be avoided if diversity is taken into account in the development teams. The industry is only finding out about this right now.” He stresses that guidelines are necessary for AI developers for this. “Microsoft, IBM, Google, Facebook, Amazon and a few other companies have founded the non-profit organisation ‘Partnership on AI’ to address this,” Andrea Martin mentions.
“There they jointly devise principles for best practices in the development of AI solutions. This is so that the things that we do can benefit society as well as business to the greatest extent possible, and not be in conflict with them.” Reinhard Karger points out just how difficult this can be: “Massive amounts of data are required to train neural networks. But how can we verify whether this data actually corresponds to the demands of today’s society? Should thousands of people be trained to individually check the data? What are the criteria? And, must this examination be repeated on a regular basis? It is very difficult to eliminate the bias of a system.”
“Especially with an aim towards productivity and efficiency gains, Artificial Intelligence can trigger very positive developments.”
Thomas Staudinger, Vice President Marketing, EBV
Rules for AI development
A similar challenge is the question of the ethics of decisions made by AI systems. What should an autonomously driven car do, for example, if it is in an emergency situation and must choose between the life of the driver, a child on the street, or an old man on the sidewalk? “In my view this is much more important than the discussion as to whether machines enslave us at some point,” says René Büst. However, Reinhard Karger is a bit annoyed by this issue: “The probability of such a scenario occurring is infinitesimally small. When humans get into such disastrous situations, they react reflexively and do not decide according to ethical principles, which do not even exist for such a difficult dilemma.” But here, he comes up against contradiction: “There is a lot of uncertainty in this context because there are so many unanswered questions,”
surmises Oliver Gürtler, for example. “These need to be answered before decisions made by an AI solution can be accepted – not only with regard to autonomous driving but also regarding applications such as those in the field of medicine.” Andrea Martin, too, considers the issue of ethics in conjunction with AI to be pertinent: “The question is, of course, raised by that segment of society which is not so intensely preoccupied with Artificial Intelligence. We still have a very long way to go, however; there are still many technical basics to be mastered before a car has to make such decisions. I therefore believe that we still have time to answer the question.” Nevertheless, just as with René Büst, she sees the need to form ethics commissions which are dedicated to resolving moral issues arising from AI use. “Not to frighten people, but instead to make AI socially acceptable,” Büst emphasizes.
Approaches to integration in companies
Consumers are one thing, says Thomas Staudinger: “But how can I, as a medium-sized company, integrate AI into my business processes? AI is not an end in itself.” Ultimately, it is a question of the strategy which acompany’s management must set forth, declares Oliver Gürtler, among others, and emphasises: “AI offers a great opportunity for an enterprise to differentiate themselves from the competition.” As a company, though, what can you really do with AI? How can you set up a new business model based on it? What processes can be improved with it? According to Staudinger, these are all questions which confront many decision-makers in companies today: “There is still great need for clarification. Best practice examples could help to make the benefits of AI solutions more understandable and the topic more tangible.”
Andrea Martin advises to switch the perspective: “The field of AI is so broad that it could crush companies. Instead of seeing it as a giant beast which needs to be slain, one should clearly understand that AI is a modular concept. You can pick out individual elements and create dedicated solutions.” René Büst recommends to first start by automating processes in a company’s IT area with the help of AI. “With the information you collect while doing this, you can then extend AI solutions to other business processes and, to the same extent, create an awareness in the company.” Thomas Staudinger, who has his focus on the end-customers of his customers, sees the responsibility at the decision-maker level: “It’s all about providing the end customer with better service based on AI. I can’t achieve this with IT; instead, this is a business decision.” To the contrary, Oliver Gürtler recommends just getting on with it: “In our experience, it is extremely productive if both young professionals and experienced staff creatively work together, then simply implement the solution. To begin with, these are all mini-projects which do not require five years of software development, but can instead be implemented as a very “lean” solution to supplement the existing IT. Once companies start to see the benefits of AI, then they will also learn to understand AI for themselves.” This would lead to the emergence of truly transformative projects, according to Gürtler. Reinhard Karger also thinks that much of the potential that lies in staff: “For small businesses in particular, it makes sense for them to ask their own employees – through the company-internal suggestion programme, for example. Because, above all else, imagination is needed to introduce AI aas opposed to technological expertise.”
Realising opportunities
All participants agree that one should look at the opportunities offered by Artificial Intelligence – in production, research, and logistics, but also in the back office with regard to taxes, insurance, and much, much more. “It’s a long way between the fictional super-intelligence of a Terminator and a simple assistant,” stresses Andrea Martin. The round-table participants view AI above all as a possibility to supplement human capabilities – not replace them. Consequently, Reinhard Karger also surmises optimistically: “Yes, Artificial Intelligence will change our lives – and that is terrific.”
AI in production
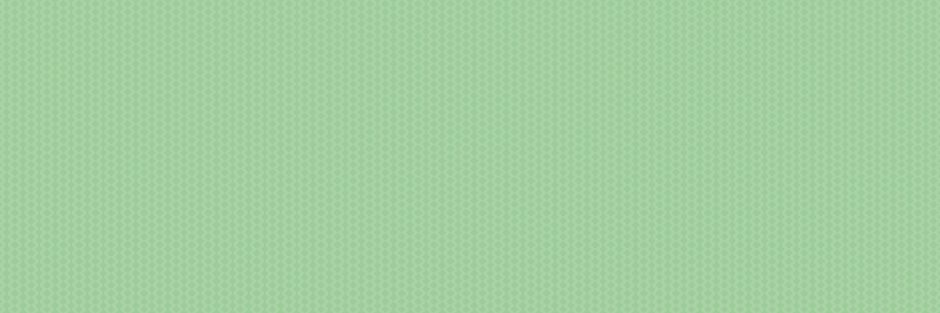
Artificial Intelligence boosts quality and productivity in manufacturing industry, and helps people in their work.
Artificial Intelligence can become a driver of growth for industry: according to a survey by management consultants McKinsey, GDP in Germany alone could rise by as much as 4 per cent, or EUR 160 billion, more than without AI by 2030 thanks to the early and concerted deployment of intelligent robots and self-learning computers. “AI promises to deliver benefits not only economically, but also in terms of business management: it enables employees to leave repetitive or hazardous tasks to computers and robots, and focus themselves on value-adding and interesting work,” says Harald Bauer, Senior Partner at McKinsey’s Frankfurt office.
AI and machine learning are exploiting opportunities in connection with Industry 4.0 in particular because enabling a production operation to organise itself autonomously and respond flexibly takes huge volumes of data. Computer systems autonomously identify structures, patterns and laws in the flood of data. This enables companies to derive new knowledge from empirical data. In this way, trends and anomalies can be detected – in real time, while the system is running.
Manufacturing industry offers many starting points where AI can boost competitive edge. At the moment, 70 % of all collected production data is not used – but AI can change that. (Source: obs / A.T. Kearney)
Proactive intervention before the machine breaks down
Predictive maintenance, particular offers genuine potential for rationalisation. Large numbers of sensors capture readings on the state of a machine or production line, such as vibration, voltage, temperature and pressure data, and transfer it to an analysis system. The data analysis enables predictions to be made. When are the systems likely to fail? When is the optimum time to carry out maintenance? This can reduce – or even rule out – the risk of breakdowns. McKinsey estimates that plant operators can improve their capacity utilisation by as much as 20 per cent by planning and executing their maintenance predictively.
Plant availability increases by up to 20 % while maintenance costs decrease by up to 10 %.
As one example, German start-up Sensosurf integrates force sensors directly into machine components that have no intrinsic intelligence of their own, such as flanged and pedestal bearings, linear guides and threaded rods. “We are working in areas where there has been little or no information available to date,” says Dr. Cord Winkelmann, CEO of Bremen-based company Sensosurf. The data obtained in this way is interpreted with the aid of custom machine-learning algorithms. As a result, specific irregularities can be detected and breakdowns prevented, for example.
Listening to make sure the machine is running smoothly
The intelligent Predisound system from Israeli company 3DSignals does not measure the deformation or vibration of a component, but instead records the acoustic sounds of a machine. Experienced machine operators and maintenance staff are able to determine from the sound of a machine whether it is running smoothly. In the ideal case, they can even predict impending breakdowns. Such detection measures are of course not entirely reliable – and they tie up personnel. Predisound aims to solve both those issues. The system consists of large numbers of ultrasonic sensors installed in the machines being monitored. The sensors record the full sound spectrum during the machine’s operation, and transmit the data to a centralised software program based on a neuronal network. By applying so-called deep learning, the software gradually recognises ever more precisely which variations in the sound pattern might be critical. This means that anomalies which would be indiscernible to a human can be detected. Based on predictive analytics algorithms, the probability of failure and time to failure of individual machine components can be predicted. The maintenance engineer is automatically notified before any damage occurs that might cause a machine to shut down. As a consequence, fixed inspection intervals are no longer necessary.
In certain operations, productivity increases by up to 20 % due to the AI-based interaction of humans and robots. (Source: McKinsey)
More effective quality control
Another key area of application for AI alongside machine maintenance is industrial image processing. Automatic pattern recognition by means of cameras and sensors enables faults and their causes to be detected more quickly. That is a great aid to quality control. Bosch’s APAS inspector is one example: it uses learning image processing to automatically detect whether or not the material surface of a production component conforms to specifications. The operator teaches the machine once what non-conformity it can tolerate, and when a component has to be taken offline. Artificial Intelligence then enables the machine to autonomously apply the patterns it has learned to all subsequent quality inspections.
Robots learning independently thanks to Artificial Intelligence
Thanks to AI, industrial robots are also increasingly becoming partners to factory staff, working with them hand-in-hand. One example of a collaborative robot of this kind is the Panda from Franka Emika. It is a lightweight robot arm capable of highly delicate movements. The medium-term aim of developer Sami Haddadin is to turn Panda into a learning robot that no longer has to be programmed. The human controller specifies a task to perform, and Panda tries out for itself the best way to perform it. The key feature is that once Panda has found the most efficient method, it relays the information to other robots via the cloud. This means the production company does not have to invest in costly and complex programming.
“We are just at the beginning of an exciting development,” asserts Matthias Breunig, a Partner at McKinsey’s Hamburg office. “Key to the beneficial application of AI is an open debate as to how and where humans and machines can work together advantageously.”
The human face of AI chatbots
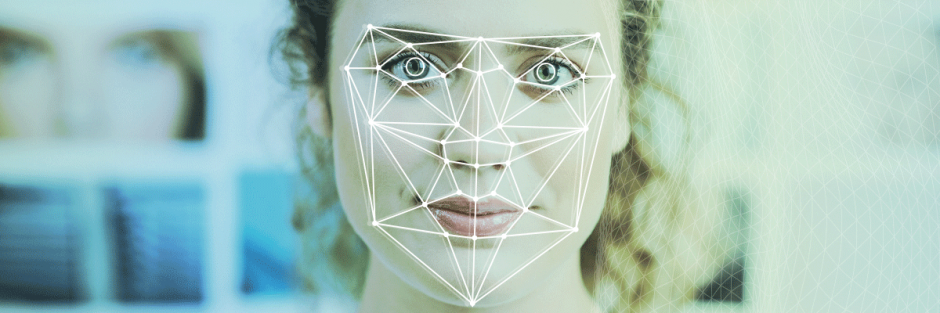
Software systems that communicate with people via text or speech are becoming increasingly sophisticated: they recognise the other party’s mood and respond as an avatar with suitable gestures and facial expressions.
For British electrical retailer Dixons Carphone, the shopping experience for nine out of ten customers begins online. Two thirds of customers access the virtual retail shop via mobile technology, such as smartphone, in order to check product information and compare prices. All are greeted by Cami, the company’s AI chatbot. Cami is designed to learn from the chats so that it can anticipate the needs of customers, match these needs with current prices and stocks and can thus answer all questions quickly and precisely. The e-commerce retailer is thus recording significant growth in sales and creating scope for its human sales employees, who can now offer valuable customer services in the time they have saved.
Communication is becoming increasingly natural thanks to Artificial Intelligence
Chatbots – or software programs that can communicate with people via text or speech – are now enabling increasingly more natural communication with users: they use Artificial Intelligence, especially natural-language -processing (NLP) technologies, to understand and generate speech. Natural-language understanding interprets what the user is saying and assigns it to a pattern stored in the computer. Natural-language generation creates a natural voice response that can be understood by the user.
If a chatbot is integrated fully automatically, it handles the interaction with the customer by itself. During the conversation, the bot selects the responses it gives to the customer or can decide to forward the dialogue to a human agent if it does not understand the question. A semi-automatic chatbot, in contrast, does not act fully autonomously, rather suggests answers to a human agent. The agent can then select the most suitable response from those offered, revise the response or also take over the dialogue from this point. The advantage of this is that the chatbot can continue to learn from the interaction while already supporting the agent to respond faster and more purposefully. At the same time, there is less of a risk that valuable customer contacts will be lost as a result of errors by the bot. “The evolution of chatbots is only just beginning,” says Wolfgang Reinhardt, CEO of optimise-it, one of the leading providers of live chat and messaging services in Europe. “In the coming months and years, they will become even more intelligent and sophisticated. What is important in this respect is that the bots are incorporated and networked into the communication structure of the company so as to offer the customer the best possible user and service experience.”
AI chatbots in a new guise
The chatbots are to get a new look too for this purpose, too as avatars, they also emulate humans graphically. Cologne-based company Charamel and the German Research Centre for Artificial Intelligence (DFKI) have already been working for some time in the area of virtual avatars. Charamel’s VuppetMaster avatar platform allows users to integrate interactive avatars into web applications and other platforms without having to install additional software. The aim is to develop a next generation of multimodal voice-response systems that can make more extensive use of facial expressions, gestures and body language on the output side in order to enable a natural conversation flow. Prof. Wolfgang Wahlster, Chairman of the Executive Board of the DFKI: “That will make chatbots credible virtual characters with their own personalities. This new form of digital communication will make customer dialogues and recommendation, advice and tutoring systems in the Internet of Services even more efficient and appealing. These personalised virtual service providers will make it even easier for people to use the world of smart services, turning it into a very personal experience.”
Face-to-face
“At the end of the day, as human beings, the most emotionally engaged conversations we have are face-to-face conversations,” reckons Greg Cross, Chief Business -Officer at Soul Machines. “When we communicate face-to-face, we open up an entire series of new non-verbal communications channels.” Many bots are already very good at understanding what someone is saying. It will be more important in future, however, to also be able to evaluate how someone says something. The more than 20 facial expressions a -person uses help in this respect. For example, winking after a sentence indicates that the statement should not be taken too seriously. Recognising this is something that machines or chatbots should now also be able to do. This already works impressively as the life-like avatar from Soul Machines demonstrates. Its computer engine uses neural networks to mimic the human nervous system. When the user starts the system, a camera begins to read and interpret their facial expressions. At the same time, a microphone records the request. The request is interpreted based on the Artificial-Intelligence solution Watson from IBM and a suitable response is given. The Soul Machines engine recognises the emotions in parallel based on the facial expression and voice tone so that it can better understand how someone is interacting with the system.
Because people are interacting with more and more machines in their everyday lives, giving AI a human face is becoming increasingly important for Greg Cross: “We see the human face as being an absolutely critical part of the human-machine interaction in the future.”
Drones controlled by AI
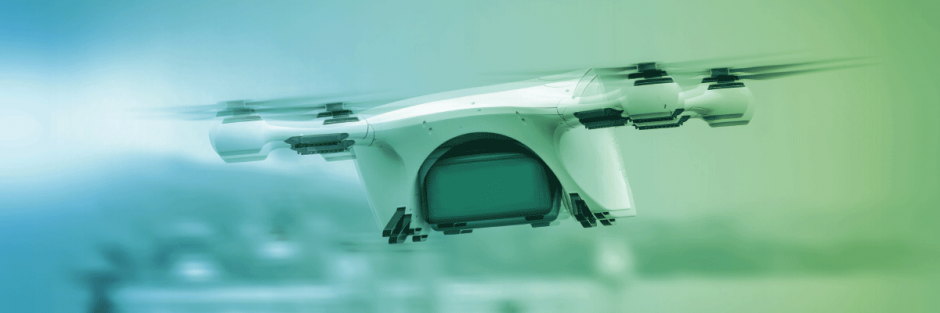
Drones controlled by Artificial Intelligence can already deliver similar performance today to those controlled by humans. Even in an urban setting they are capable of navigating safely.
Congested streets, rising emission levels and the lack of parking all combine to make urban logistics an increasingly greater challenge. Powered by e-commerce, the package market is growing by seven to ten per cent annually in mature markets such as the United States or -Germany. This will see the volume double in Germany by 2025, with around five billion packages mailed each year. “While -deliveries to consumers have previously made up about 40 per cent, more than half of all packages are now delivered to private households. Timely delivery in ever greater -demand,” says Jürgen Schröder, a McKinsey -Senior Partner and expert in logistics and postal services. “New technologies like autonomous driving and drone delivery still need to be developed further. They present opportunities to reduce costs and simplify delivery. We expect that by 2025, it will be possible to deliver around 80 per cent of packages by automated means.”
Package-carrying drones, as Amazon put forward for the first time in 2013, were initially laughed off as a crazy idea. Today, a large number of companies are experimenting with delivery by drone. One example is Mercedes-Benz with its Vans & Drones concept, in which the package is not directly delivered to the customer via a drone, but in a commercial vehicle. In the summer of 2017, the company carried out autonomous drone missions for the first time in an urban environment in Zurich. In the course of the pilot project, -customers could order selected products on Swiss online marketplace siroop. These were a -maximum of two -kilograms in weight and suitable for transport by drone. The range of products included coffee and -electronics. The -customers received their goods the same day. The retailer loaded the drones immediately after receiving the -order on its own premises. After this, they flew to one of two Mercedes vans used in the project, which featured an -integrated drone landing platform. The vans stopped at one of four -pre–determined “rendezvous points” in the Zurich metropolitan area. At these points, the mail carriers received the products and delivered them to the customers, while the drone returned to the retailer. Overall, some 100 flights were -successfully completed without any incidents across the urban area. “We believe that drone-based logistics networks will fundamentally change the way we access products on a daily basis,” says Andreas Raptopoulos, Founder and CEO of Matternet, the manufacturer of the drones used in the test.
Reliably dodging obstacles thanks to Artificial Intelligence
An essential element of such applications are drones that can fly safely between buildings or in a dense street network, where cyclists and pedestrians can suddenly cross their path. Researchers at the University of Zurich and the NCCR Robotics research centre developed an intelligent solution for this purpose. Instead of relying on sophisticated -sensor systems, the drone developed by the Swiss -researchers uses a standard smartphone camera and a very -powerful AI -algorithm called DroNet. “DroNet recognises static and dynamic obstacles and can slow down to avoid crashing into them. With this algorithm, we have taken a step forward towards integrating autonomously navigating drones into our everyday life,” explains Davide Scaramuzza, Professor for Robotics and Perception at the University of Zurich. For each input image, the algorithm generates two outputs: one for navigation to fly around obstacles and one for the likelihood of collisions to detect dangerous situations and make it possible to respond. In order to gain enough data to train the algorithm, information was collected from cars and bicycles that were travelling in urban environments in accordance with the traffic rules. By imitating them, the drone -automatically learned to respect the safety rules, for example “How do we follow the street without crossing into the oncoming lane” or “How do we stop when obstacles like pedestrians, construction works or other vehicles block the way?”. Having been trained in this way, the drone is not only capable of navigating roads, but also of finding its way around in completely different environments than those it was ever trained for – such as multi-storey car parks or office corridors.
Drones controlled by Artificial Intelligence are winning the race
Just how sophisticated drones controlled by Artificial Intelli-gence are today was demonstrated in a race organised by NASA’s Jet Propulsion Laboratory (JPL), when world-class drone pilot Ken Loo took on Artificial Intelligence in a timed trial. “We pitted our algorithms against a human, who flies a lot more by feel,” said Rob Reid of JPL, the project’s task manager. Compared to Loo, the drones flew more cautiously but consistently. The drones needed around 3 seconds longer for the course, but kept their lap times constant at a speed of up to 64 kilometres per hour, while the human pilot varied greatly and was already exhausted after a few laps.
(Picture Credit: Daimler AG)
Neuronal networks simulating the brain
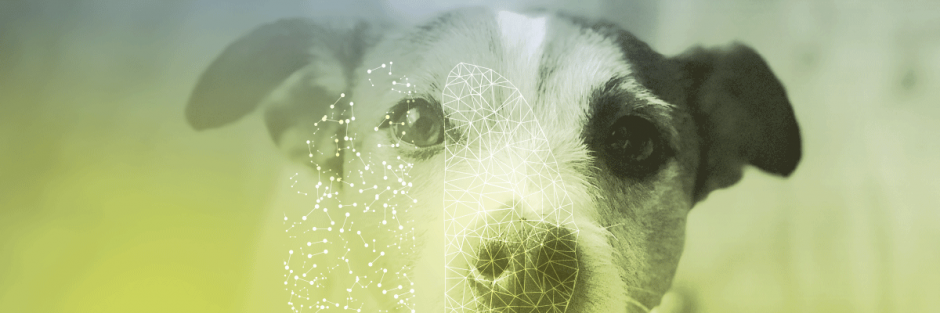
Machines are being made more intelligent based on a variety of data analysis methods. The focus of these efforts is shifting increasingly from mere performance capability towards creating the kind of flexibility that the human brain achieves. Artificial neuronal networks are playing a big role.
All forms of Artificial Intelligence are not the same – there are different approaches to how the systems map their knowledge. A distinction is made primarily between two methods: neuronal networks and symbolic AI.
Knowledge represented by symbols
Conventional AI is mainly about logical analysis and planning of tasks. Symbolic, or rule-based, AI is the original method developed back in the 1950s. It attempts to simulate human intelligence by processing abstract symbols and with the aid of formal logic. This means that facts, events or actions are represented by concrete and unambiguous symbols. Based on these symbols, mathematical operations can be defined, such as the programming paradigm “if X, then Y, otherwise Z”. The knowledge – that is to say, the sum of all symbols – is stored in large databases against which the inputs are cross-checked. The databases must be “fed” in advance by humans. Classic applications of -symbolic AI include, for example, text processing and voice recognition. Probably the most famous example of -symbolic AI is DeepBlue, IBM’s chess computer which beat then world champion Garry Kasparov using symbolic AI in 1997.
As computer performance increases steadily, symbolic AI is able to solve ever more complex problems. It works on the basis of fixed rules, however. For a machine to operate beyond tightly constrained bounds, it needs much more flexible AI capable of handling uncertainty and processing new experiences.
Advancing knowledge about neurons autonomously
That flexibility is offered by artificial neuronal networks, which are currently the focus of research activity. They simulate the functionality of the human brain. Like in nature, artificial neuronal networks are made up of nodes, known as neurons, or also units. They receive information from their environment or from other neurons and relay it in modified form to other units or back to the environment (as output). There are three different kinds of unit:
Input units receive various kinds of information from the outside world. This may be measurement data or image information, for example. The data, such as a photo of an animal, is analysed across multiple layers by hidden units. At the end of the process, output units present the result to the outside world: “The photo shows a dog.” The analysis is based on the edge by which the individual neurons are interconnected. The strength of the connection between two neurons is expressed by a weight. The greater the weight, the more one unit influences another. Thus the knowledge of a neuronal network is stored in its weights. Learning normally occurs by a change in weight; how and when a weight changes is defined in learning rules. So before a neuronal network can be used in -practice, it must first be taught those learning rules. Then neuronal networks are able to apply their learning algorithm to learn independently and grow autonomously. That is what makes neuronal AI a highly dynamic, adaptable system capable of mastering challenges at which symbolic AI fails.
Cognitive processes as the basis of a new AI
Another new form of Artificial Intelligence has been developed by computer scientists at the University of -Tübingen: their “Brain Control” computer program simulates a 2D world and virtual figures – or agents – that act, cooperate and learn autonomously within it. The aim of the simulation is to translate state-of-the-art cognitive science theories into a model and research new variants of AI. Brain Control has not made use of neuronal networks to date, but nor does it adhere to the conventional AI paradigm. The core theoretical idea underlying the program originates from a cognitive psychology theory, according to which cognitive processes are essentially predictable, and based on so-called events. According to the theory, events – such as a movement to grip a pen, and sequences of events such as packing up at the end of the working day – form the building blocks of cognition, by which interactions, and sequences of interactions, with the world are selected and controlled in a goal-oriented way. This hypothesis is mirrored by Brain Control: the figures plan and decide by simulating events and their sequencing, and are thus able to carry out quite complex sequences of actions. In this way, the virtual figures can even act collaboratively. First, one figure places another figure on a platform so that the second figure can clear the way, then both of them are able to advance. The modelling of cognitive systems such as in Brain Control is still an ambitious undertaking. But its aim is to deliver improved AI over the long term.
Autonomous trains on long-distance

Our world’s growing metropolises require a new kind of transport network. Autonomous trains and completely new train concepts will revolutionise passenger and freight traffic between cities.
It’s 6 pm in Berlin. It would be nice to go and try out that new restaurant in Hamburg. So you order an autonomous taxi from Uber via your smartphone. It arrives punctually at your office, picks up your wife from her workplace and then heads over to the central station Hyperloop portal. The autonomous taxi has already contacted the vacuum conveyor, so it can drive directly into the waiting Hyperloop pod. The pod accelerates gradually until it reaches airline speed, and you get to Hamburg in just 20 minutes. The Uber taxi drives out of the Hyperloop portal and straight to the restaurant … This is the vision of Doug Chey, Senior Vice President Systems Development at Hyperloop One. “It’s direct, autonomous, ultrafast intercity travel and the doors only open twice, once to let you in and once to let you out,” he writes in his Hyperloop One blog. He does admit, however, that this is the long-term vision. “We obviously have a ton of engineering work to do.” Elon Musk, who is already successfully building electric cars with his company Tesla and launching spacecraft with SpaceX, is the original visionary behind these trains in vacuum tubes. Hyperloop One is one of the companies working on implementing the idea and has already carried out some initial driving tests with the vehicles – but currently only at a speed of just over 100 kilometres per hour.
Driverless doesn’t equal autonomous
For now, “traditional” trains will continue to connect metropolises. But here, too, there is a trend towards more and more automation. In the future, as well as the doors closing automatically, the entire train will run automatically.
Driverless trains were introduced a number of years ago and can be found in cities including Nuremberg, Singapore and Paris. However, they are only in use in local transport systems. What’s more, they are not truly autonomous, because the intelligence that runs them is in the infrastructure; only a little of it – if any – is in the railway vehicle itself. None of the driverless trains we have seen thus far are fitted with any sensors to monitor the route in front of them. In addition, the trains are controlled remotely via a computer system that is installed in a control room and do not make any decisions for themselves.
However, this will change in the not too distant future, and it is a change that will bring significant benefits. Autonomous trains can run more frequently and achieve higher speeds, enabling managers to increase the number of trains in operation on a route instead of having to go to the significant expense of building new tracks.
The French rail operator SNCF, for example, intends to run its TGV high-speed train autonomously from 2023 onwards. Equipped with its own sensors, the train should be able to detect obstructions in its path and brake automatically. Testing is due to start in 2019. There will still be a train driver on board of autonomous trains, although he or she would only intervene in the event of an emergency. SNCF expects to be able to increase the speed and frequency of rail connections with an autonomous TGV and operate up to 25 per cent more high-speed trains on the route between Paris and Lyon.
Deutsche Bahn has also announced that it will be introducing autonomous trains on a small number of selected routes by 2023 at the latest. The company started a pilot project for its autonomous trains in the Ore Mountains in 2017, running a converted diesel train in an “automated” manner on a 25-kilometres test route. The vehicle has to learn to detect obstacles and optical signals in its path. “The train will have sufficient intelligence to work with the existing infrastructure,” according to project coordinator Michael T. Hoffmann. Sensor systems like those fitted to driverless cars (cameras, radar and lidar, for example) are used for the optical measurement of distance and speed. However, the vehicle does not make its own decisions, as “a control room remains in control”, to quote Hoffmann.
Intelligence for single wagons in freight transport
The gift of autonomy will be bestowed not only to complete trains. The German Aerospace Centre, or DLR for short, recently presented NGT Cargo, a concept for the freight trains of the future which gives every individual wagon the capability to be autonomous. “Freight transport is currently dominated by block trains that are not shunted and that use a large number of wagons to carry large, standard volumes of freight from point A to point B,” explains DLR researcher Dr Joachim Winter, who is leading the Next Generation Train (NGT) project. Coupling these trains, however, takes up a great deal of time and significant resources. The automatically driven NGT Cargo trains will be made up of single wagons and powerful end cars, automatically coupled together as required. The intelligent freight wagons in the NGT Cargo concept have a separate drive based on electric motors and a battery that stores energy recovered during braking. This makes it possible for the single wagons to shunt autonomously and, thanks to their sensors, they can even travel the final kilometres to the respective customer autonomously.
The challenges of powering Artificial Intelligence
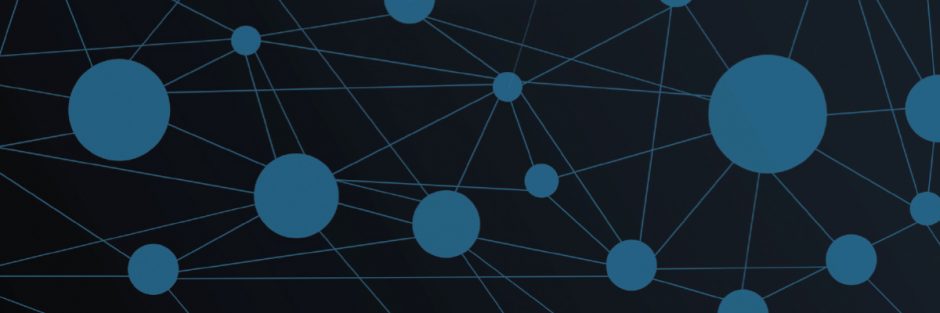
Making a smart, connected world possible depends on energy-efficient data centres.
The invention of computers changed the world due to their ability to retain and share information, but up until recently, they lacked the capability to emulate a human brain and autonomously learn in order to perform tasks or make decisions.
To come close to the processing power of a human brain, an AI system must perform around 40 thousand trillion operations per second (or 40 PetaFLOPS). A typical server farm with this level of AI computing power would consume nearly 6 MW of power, whereas the human brain by comparison requires the calorific equivalent of only 20 W of power to perform the same tasks. Some of AI’s most advanced learning systems are currently consuming power at up to 15 MW – levels that would power a small European town of around 1500 homes for an entire day.
AI’s neural networks learn through exposure to differentiation, similar to human learning. Typically, thousands of images are processed through Graphics Processing Units (GPUs) set up in parallel in order for the network to compare and learn as quickly as possible.
AI computing is also dependent on so-called edge devices, including cameras, sensors, data collectors and actuators, to receive input information and output movement or actions in the physical world. Consumer and manufacturing trends such as the Internet of Things (IoT) have also led to the proliferation of AI-enabled devices in homes and factories, thereby also requiring increased data and energy consumption.
Delivering and managing megawatts of power is constantly underscored by pressure from rising energy prices. Additionally, every watt of energy dissipated in the data centres requires more cooling, increasing energy costs further.
Miniaturisation is central to improving processing power, but smaller sizes with increased power density reduce the surface area available for dissipating heat. Thermal management is therefore one of the most significant challenges in designing power for this new generation of AI supercomputers.
Reducing CO2 emissions
Estimates predict that there will be over 50 billion cloud-connected sensors and IoT devices by 2020. The combined effect these devices and the data centres that power Artificial Intelligence will have on global power consumption and global warming indicates the need for collective action to make power supplies for server racks, edge devices, and IoT devices much more energy-efficient.
In addition to investments in renewable energy production and attempts to move away from the use of petrol and diesel vehicles, European countries will need to place significant focus on energy efficiency in their efforts to cut carbon emissions. The European Commission implemented the Code of Conduct for Energy Efficiency in Data Centres in 2008 as a voluntary initiative to help all stakeholders improve energy efficiency, but data centres are still on course to consume as much as 104 TWh in Europe alone by 2020, almost doubled from 56 TWh in 2007.
According to a 2017 study on data centre energy consumption, the Information and Communication Technology (ICT) sector generates up to 2 per cent of the world’s total carbon dioxide emissions – a percentage on par with global emissions from the aviation sector. Data centres make up 14 per cent of this ICT footprint.
However, another report states that ICT-enabled solutions such as energy-efficient technologies could reduce the EU’s total carbon emissions by over 1.5 gigatonnes (Gt) of CO²e (carbon dioxide equivalent) by 2030. This would be a vast saving, almost equivalent to 37 per cent of the EU’s total carbon emissions in 2012.
Analogue vs. digital controllers
AI will no doubt have a significant impact on human society in the future. However, the repetitive algorithms of AI require a significant change to computing architectures and the processors themselves. As a result, powering these new AI systems will remain a persistent challenge.
Clearly, power solution sophistication must increase and, as a result, power management products have now emerged with advanced digital control techniques, replacing legacy analogue-based solutions.
Digital control has been shown to increase overall system flexibility and adaptability when designing high-end power solutions. A digital approach allows controllers to be customised without costly and time-consuming silicon spins and simplifies designing and building the scalable power solutions required for AI. Even with all of the included functionality and precision delivery of power, digital solutions are now price-competitive with the analogue solutions they will replace.
Making the power solutions for AI applications of the future as efficient as possible is a relatively easy and attainable way in which the ICT sector can contribute to reducing global carbon emissions.
Clayton Cornell, Technical Editor, Infineon Technologies AG
Better speech recognition thanks to Deep Learning
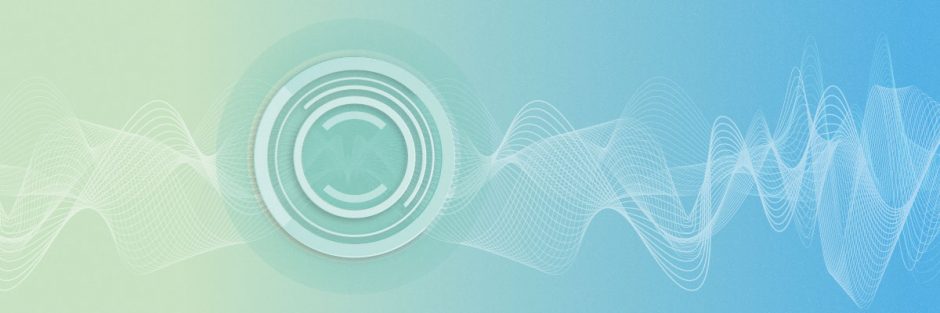
Digital assistants are becoming increasingly sophisticated at recognising speech thanks to deep-learning methods. And owing to their AI ability, they are even capable of predicting what their users want.
“Tea, Earl Grey, hot” – every Star Trek fan is familiar with the words Captain Picard uses to order his favourite drink from the replicator. Use of speech to control computers and spaceships is an unwavering element of most science-fiction films. Attempts have been made for many years to control machines through speech: the first speech-recognition software for computers, generally speaking, was presented to the public by IBM in 1984. Some ten years later, it was developed for the PC and thus for the mass market. Meanwhile, Microsoft used speech recognition in an operating system for the first time in 2007 with Windows Vista.
Apple was responsible for the breakthrough on the mass market in 2011, when it launched its speech-recognition-software assistant Siri for the iPhone 4s. Siri now shares the market with a number of similar solutions: Amazon’s Alexa, Cortana from Microsoft or Google’s Assistant. Common to all systems is that the speech input is not processed locally on the mobile device, rather on servers at the company: the voice message is sent to a data centre and converted there from spoken to written language. This allows the actual assistant system to recognise commands and questions and respond accordingly. An answer is generated and sent back locally to the mobile device – sometimes as a data record and sometimes as a finished sound file. With fast mobile Internet connections needed for this purpose, speech recognition is therefore benefiting from the current trend towards cloud computing and faster mobile Internet connections.
The error rate of speech-recognition systems has decreased significantly from 27% in 1997 to only about 6% in 2016!
Enhanced-quality speech recognition thanks to Deep Learning and Artificial Intelligence
Speech-recognition systems have benefited primarily in recent times from Artificial Intelligence. Self-learning algorithms ensure that machine understanding of speech is improving all the time: the error rate with computer-based speech recognition fell according to a study by McKinsey in 2017 from 27 per cent in 1997 to 6 per cent in 2016. Thanks to deep learning, the systems are getting increasingly better at recognising and learning the speaking patterns, dialects and accents of users.
Nuance – whose voice technology is incidentally behind Apple’s Siri – was also able to increase the precision of its Dragon speech-recognition solution, which it launched in 2017, by up to 10 per cent in comparison with the predecessor version. The software consistently uses deep learning and neural networks in this regard: on one hand at the speech model level, where the frequency of words and their typical combinations are recorded. And, on the other hand, also at the level of the acoustic model, where the phonemes or smallest spoken units of speech are modelled. “Deep-learning methods normally require access to a comprehensive range of data and complex hardware in the data centre in order to train the neural networks,” explains Nils Lenke, Senior Director Corporate Research at Nuance Communications. “At Nuance, however, we managed to bring this training directly to the Mac. Dragon uses the specific speech data of the user and is therefore continuously learning. This allows us to increase the precision significantly.”
Predictive assistants
AI not only improves speech recognition, however, but also the quality of the services offered by digital assistants such as Alexa, Siri and others. The reason for this is that the systems can deal with topics predictively based on their learning ability and make recommendations. Microsoft’s Cortana uses a notebook – like a human assistant – for this purpose, in which it notes down the interests and preferences of the user, frequently visited locations or rest periods when the user prefers not to be disturbed. For example, if the user asks about weather and traffic conditions every day before leaving for work, the system can offer the information independently after several iterations without the user needing to ask actively.
Voice control of IoT devices
The digital assistants become especially exciting when they are networked with the Internet of Things, since they can be used to control a whole host of different electronic equipment. Digital assistants will already be supported by more than 5 billion devices in the consumer sector in 2018, according to IHS Markit market researchers, with a further 3 billion devices to be added by 2021. Even today, for example, the smart home can be operated by voice commands using digital assistants.
In the US, Ford has also been integrating the Alexa voice assistant into its vehicles since the start of 2017 – thus incorporating the Amazon App into the car for the first time. Drivers can therefore enjoy audio books at the wheel, shop in the Amazon universe, search for local destinations, transfer these directly to the navigation system, and much more. “Ford and Amazon share the vision that everyone should be able to access and operate their favourite mobile devices and services using their own voice,” explains Don Butler, Executive Director of Ford Connected Vehicle and Services. “Soon our customers will be able to start their cars from home and operate their connected homes when on the go – we are thus making their lives easier step by step.”
And something else that is sure to please Star Trek fans: thanks to Alexa, you can now also order your hot drink with a voice command. Coffee supplier Tchibo has launched a capsule machine onto the market, for example, which can be connected to Alexa via WLAN. As a result you can order your morning coffee from the comfort of your bed: “Coffee, pronto!”
Autonomous ships set sail
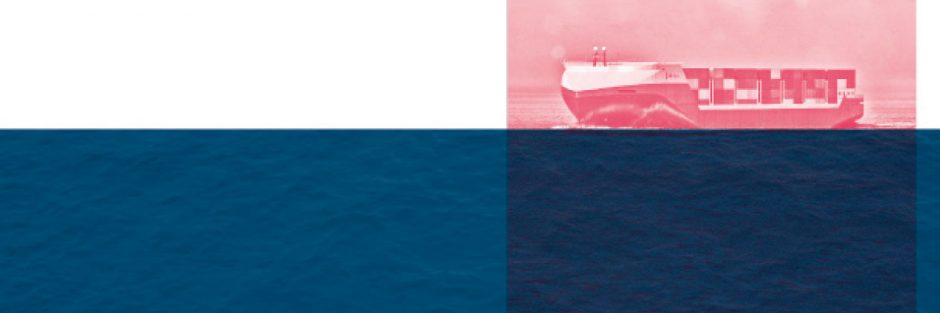
A lack of qualified staff, higher efficiency and better security are grounds for extending automation to seafaring applications. The first ships with autonomous functions are set to be launched in 2018.
It is not a matter of whether autonomous ships will exist, but when. Oskar Levander, the Vice President of Innovation – Marine at Rolls-Royce, is convinced: “The technologies needed to make remote and autonomous ships a reality exist. We will see a remote-controlled ship in commercial use by the end of the decade.” The British company has been active in this area, including starting the Advanced Autonomous Waterborne Applications Initiative (AAWA). Several Finnish universities, companies from the shipping industry and classification society DNV GL participated in this project, which ended in July 2017. The aim of the initiative was to develop the technologies for remote-controlled and autonomous ships.
Autonomy Mixed with Remote Control
There are similar projects around the world, which generally do not envisage completely autonomous operation. Human beings are intended to resume control by no later than the time of docking and undocking at the port. However, they will no longer be sitting on the ship’s bridge, but inside a control centre on land. In the future, they are also expected to monitor the ships in autonomous operation from this vantage point, for instance as the vessels navigate the open sea.
“The advantages of unmanned ships are manifold, but primarily centre on the safeguarding of life and reduction in the cost of production and operations,” explains Brett A. Phaneuf, Managing Director of the British firm Automated Ships. According to a study by Munich-based Allianz Insurance, between 75 and 96 per cent of accidents at sea can be attributed to errors made by the crew, often as a result of fatigue. Remote-controlled or autonomous vessels would significantly reduce this risk. A further benefit is that the ships can be designed with higher loading capacity and lower wind resistance. After all, in the absence of a crew, there is no need for bridges, quarters or systems such as heating, ventilation and wastewater management. As a result, the ships become lighter and more streamlined, fuel consumption is reduced, construction and operating costs decrease, and there is more space available for cargo. Last but not least, autonomous ships will make sailors’ work more attractive as a career. Seamen will no longer be at sea for weeks on end and instead will work on land in the control station, able to return home every night.
The Technology is Already Here
Most of the technology necessary for an autonomous vessel exists today; many features are automated on a modern ship’s bridge. The autopilot steers a set course with the help of GPS, while a cruise control system manages the speed. Radars and ship detection systems scan the environment and automatically sound the alarm in the event of danger. In addition, autonomous ships are to be equipped with more sensors. Types of sensors previously unused in a maritime context are integrated along with the normal radar in the Autosea project, for example, to provide the means to also detect small objects such as driftwood or small boats. These sensor types include lidar, infrared or 3D cameras. The Norwegian University of Science and Technology is directing the project, whose industry partners include the two Norwegian companies Kongsberg Maritime and Maritime Robotics.
Globally Connected via Satellite
A software program evaluates the data from all the sensors and determines, for example, whether and how the ship should change course to avoid collisions. People will be able to monitor what is happening via satellite and intervene if necessary. This requires a constant real-time connection with high data transfer rates. To this end, British company Inmarsat – also a partner of the AAWA project – has now deployed four Global Xpress satellites. By means of the Ka band, these can create a high-speed broadband connection that is available worldwide. This method is not only used to transfer the operating data to the control station; autonomous ships can also use it to access weather-forecast information or intelligence from other vessels for decision-making purposes, making them part of the Internet of Things.
Autonomous ships: Stages of development
2020
Reduced crew, certain functions will be executed with remote control
2025
Remote-controlled, unmanned coastal freighters
2035
Autonomous, ocean-going ships
First Ships from 2018
The plans for unmanned, ocean-going vessels have become increasingly specific. For one, the Hrönn is under construction, a joint project by Automated Ships and Kongsberg Maritime, which is intended to launch in 2018. The Hrönn is designed as a light-duty supply ship for offshore wind turbines or fish farms. The vessel will initially be remotely controlled. However, its control algorithms are due to be developed in parallel during use, later enabling it to be operated fully automatically and even autonomously. Kongsberg is also involved in another project, the Yara Birkeland, which will be the world’s first electrically powered and autonomous container ship. The ship is to first set sail – initially still with crew – in 2018, then with remote control as of 2019, and is expected to be autonomous from 2020 onwards.
“Autonomous shipping is the future of the maritime industry. As disruptive as the smartphone, the smart ship will revolutionise the landscape of ship design and operations,” Mikael Makinen, Rolls-Royce, President – Marine, states with confidence.
(Picture credit: Rolls Royce Plc)
Deep Learning in Artificial Intelligence
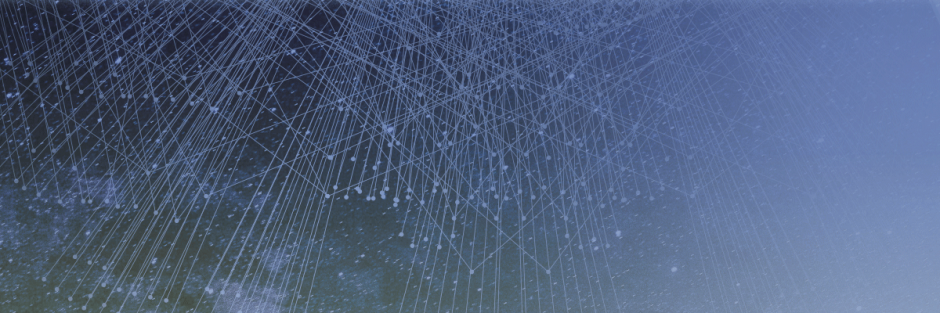
Machine learning especially deep learning are the core competences of Artificial Intelligence. Self-learning programs are being used today in increasingly more products and solutions. Machine-learning algorithms can be found in speech recognition applications on smartphones as well as in spam filters in anti-virus programs. Personalised online advertising also only works as well as it does because of learning systems. A whole range of different concepts, methods and theoretical approaches is involved in this context. Yet all have one goal in common: the computer or the machine should acquire empirical knowledge independently and, based on this find solutions autonomously for new and unknown problems. This makes machine learning one of the core fields of Artificial Intelligence, without which other core competences of smarter systems, such as pattern recognition or natural-language processing, would scarcely be conceivable. The technology is actually not especially new, with AI pioneer Marvin Minsky already developing an initial learning machine in the 1950s. However, the breakthrough and practical application of the relevant methods really only came about owing to rapid development in recent years in the area of semiconductor technology. Except that with the processor technology now available, it was possible to process large volumes of data at high speed in parallel.
Many experts regard this area as currently having the greatest potential within AI.
Deep learning currently dominates learning methods
Deep learning is a method of machine learning: many experts regard this area as currently having the greatest potential within AI. Deep learning uses complex neural networks to learn autonomously how something can be classified. The system records large volumes of known information – for example pictures or sounds – in a database and compares it with unknown data.
The procedure eliminates many work steps involved in classic machine learning. That’s because the training effort is significantly less: the “trainer” simply has to present the neural network with data such as pictures – the system discovers for itself how the objects shown in the pictures are to be classified. The human has to unambiguously indicate whether the object whose recognition is to be learned can be seen in the picture (therefore, for example, whether or not a pedestrian is shown in the picture). The deep-learning program uses the information from the training data in order to define typical features of a pedestrian and generate a prediction model from this. The system works down deeper into the neural network level by level – hence the name deep learning. The nodes at the first level, for example, only register the brightness values of the image pixels. The next level recognises that some of the pixels form lines. The third differentiates between horizontal and vertical lines. This iterative process continues until the system recognises legs, arms and faces and has learned how a person in the picture should be classified.
This learning process requires significant computing power, however, and therefore places increased demands on the processor technology. Researchers and manufacturers are consequently working intensively on developing special AI chips that can perform even more computing processes faster.
Simply a case of sharing acquired knowledge
At the same time, thought is being given as to how the knowledge that a system has elaborately acquired can be made available to other systems, too. This would then mean, for example, that not every autonomous vehicle would have to learn for itself what a pedestrian looks like, rather it could draw on the experience of vehicles that have been on the road for longer. The Khronos Group, an open consortium of leading hardware and software companies presented an exchange format for neural networks at the end of 2017. The Neural Network Exchange Format 1.0 allows scientists and engineers to transfer existing trained networks from the training platform to a host of other systems – in other words, in the same way as with PDF format in text processing.
Autonomous farm vehicles
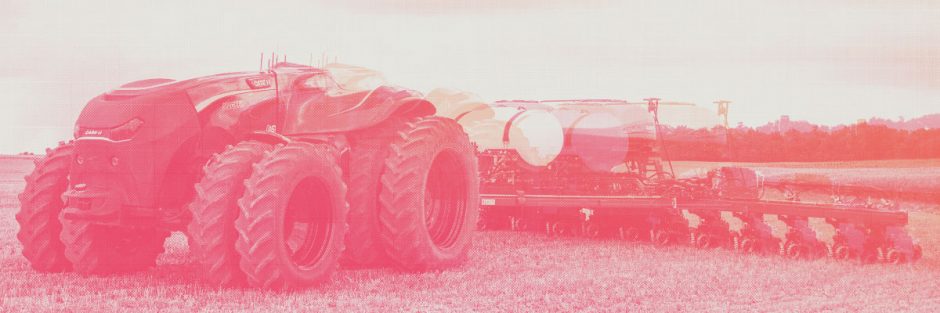
Autonomous farm vehicles will change the landscape of farming, allowing arable land to be used more efficiently while protecting the environment.
Agriculture is facing major challenges. The world’s available agricultural land will need to be used more efficiently to continue to feed its rapidly growing population in the future. Yet farms in many parts of the world today are lacking labour.
Autonomous farm vehicles: In Use 24/7
The major tractor manufacturers have therefore been working for several years on autonomous farm vehicles. For example, Case IH presented its Autonomous Concept Vehicle (ACV) to the public for the first time in 2016. “The ACV retains much of the conventional technology of a modern tractor, and uses ultra-accurate RTK GPS to provide parallel steering capability with a variation of less than 2.5 cm, which many farmers are already using to ensure missed or overlapped land between passes spans no more than this width,” Case IH’s Dan Stuart states. In addition, the ACV is equipped with radar, lidar, proximity sensors, as well as safety and wireless systems, meaning that it can be monitored and controlled from a PC or tablet. Consequently, having entered the field, the tractor can work completely independently without a driver. The ACV is still a concept. However, a test programme working with farmers in real-life conditions has already been launched.
To the Heart of Agriculture
However, in recent times, there has been a growing number of voices responding negatively to the use of large, heavy machinery on farms. Dr Jens-Karl Wegener, Head of the Institute for Application Techniques at Germany’s Federal Research Centre for Cultivated Plants, the Julius Kühn Institute, points out that “agriculture, the way it currently operates, is coming under social criticism. In the face of nitrate pollution, loss of species and soil compaction, we have to critically question how much longer it will be good for.” The use of ever larger machines on ever larger areas of land seems to be part of the problem rather than a solution. Wegener takes a different approach based on the needs of the individual plant.
“This sort of precision farming, geared to the needs of the individual plant, would naturally also affect how the land will look in the future,” project team member Lisa-Marie Urso says. Spot farming is the term she and her colleagues use for the new cultivation system, which takes account of small-scale differences in the landscape. “The benefit of spot farming would be the ability to achieve multiple crop rotations at a time, not only one, as has been the case to date,” Urso continues. Depending on the ground conditions, various crops (rape, wheat and beets) could be sown, taking into account properties of the land such as dips containing pooled water, dry hillocks or other small structures. “That would certainly increase the diversity of species in the fields, as well as saving on fertiliser and pesticides based on single-crop treatment,” Wegener comments.
Increasing intelligence thanks to machine learning
The fact that this is not merely a vision was demonstrated by start-up company Deepfield Robotics back in 2015 with its “Bonirob”, a robot the size of a small car which uses video and lidar positioning as well as satellites to navigate around the field with centimetre-precise accuracy. It is able to distinguish crops from weeds based on their leaf shapes, and removes weeds mechanically using a ramrod rather than with toxic herbicides. Any unwanted weeds are simply rammed into the ground at high speed.
In view of the diverse flora, the Bonirob’s automatic image recognition is key. Professor Dr Amos Albert, Head of Deepfield Robotics, describes the challenge: “The leaves of carrots and camellias are very similar in their early stages, for example.” So the Bonirob has to be taught how to learn and identify leaf shapes. Albert and his team apply machine learning for the purpose. The system captures large numbers of images, in which the Bosch researchers mark the weeds. “In this way, the Bonirob gradually learns to distinguish productive crops from weeds ever more efficiently based on parameters such as leaf colour, shape and size,” Albert explains. But the Bonirob is not yet available on the market as a production vehicle.
Tackling individual weeds in a targeted way
Swiss company Ecorobotix has gone one step further: it has already built an initial production run of its “Jät” robot. The test-phase machines are currently proving their worth on fields in Switzerland, France and Belgium. They are scheduled for official market launch in 2018. The solar-powered robot weighs just 130 kilograms. It works for as long as 12 hours a day with no human operator, and is controlled and configured entirely using a smartphone app. The robot orientates and positions itself using its RTK GPS, its camera and sensors. Its imaging system enables it to align itself to the rows of crops and to detect whether and where there are weeds within or between the rows. It adapts its speed to the density of the weeds as it does so. Two robotic arms target a micro-dose spray of herbicide specifically onto the detected weed.
(Picture credit: Case IH; iStockphoto: Barcin, gusach, juliedeshaies)
Artificial Intelligence is moving into smartphones and wearables
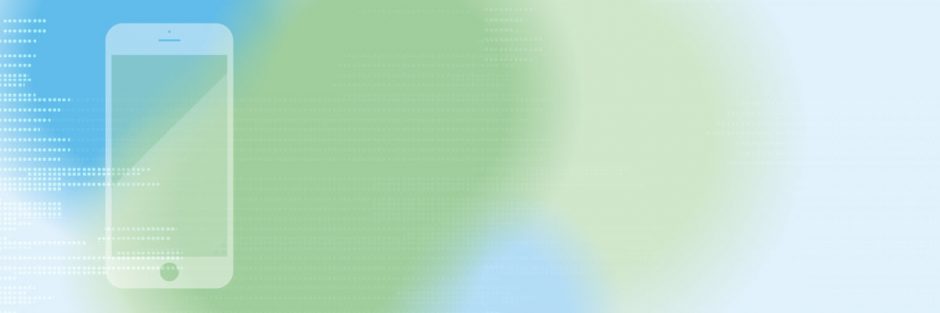
Thanks to new developments in chip technology, even small wearables such as fitness bracelets have AI on board. The latest top-of-the-range smartphones are already learning to understand their users better through neuronal networks, and are delivering significantly higher performance.
Mobile devices such as smartphones and wearables are becoming ever more important in people‘s everyday lives. “Smartphones have fundamentally changed our lives over the last 10 years. They have become the universal tool for accessing communications, content and services,” says Martin Börner, Deputy President of the industry association Bitkom.
Mobile devices are gaining AI capabilities
Now mobile devices are coming onto the market with Artificial Intelligence capable of analysing the recorded data even better, and providing users with more closely targeted recommendations to enhance their health or fitness. The trend is towards edge computing. In this, the data remains in the device, and is not – or is only in part – uploaded to the cloud for analysis. That provides a number of benefits: firstly, it reduces the load on cloud computing systems and transfer media. Secondly, latency is reduced; users receive their analysis results faster. And thirdly – a key factor in medical applications especially – personal data is kept secure on the mobile device. “AI used to rely on powerful cloud computing capabilities for data analysis and algorithms, but with the advancement of chips and the development of edge computing platforms, field devices and gateways have been entitled basic AI abilities, which allow them to assist in the initial data screening and analysis, immediate response to requirements, etc.,” states Jimmy Liu, an analyst with Trendforce.
More efficiency, performance and speed
For Huawei, too, this on-device AI is a response to existing AI issues such as latency, stability and data protection. In late 2017, the company launched two smartphone models – the Mate 10 and Mate 10 Pro – that it claims are the first in the world to feature an artificially intelligent chipset with a dedicated Neural Processing Unit (NPU). This enables the phones to learn the habits of their users. The mobile AI computing platform identifies the phone‘s most efficient operating mode, optimises its performance, and generally delivers improved efficiency and performance at faster speeds. But the main way in which Huawei is utilising AI is in real-time scene and object recognition, enabling users to shoot perfect photos.
Facial recognition on a smartphone
Apple has also fitted out its new iPhone X with a special chip for on-device AI. The neural architecture of the A11 Bionic Chip features a dual-core design and executes up to 600 billion operations per second for real-time processing. The A11 neural architecture was designed for special machine learning algorithms, and enables Face ID, Animoji and other functions. This makes it possible, for example, to unlock the phone by facial recognition. The feature, named Face ID, projects more than 30,000 invisible infrared dots onto the user‘s face. The infrared image and the dot pattern are pushed through neuronal networks in order to create a mathematical model of the user‘s face before the data is sent to the Secure Enclave to confirm a match, while machine learning is applied to track physical changes in the person‘s appearance over time. All the stored facial data is protected by the Secure Enclave to an ultra-high security level. Also, the entire processing is carried out on the device and not on the cloud in order to preserve users‘ privacy. Face ID only unlocks the iPhone X when the user looks at it, with highly-trained neuronal networks preventing any manipulation using photographs or masks.
The Right Camera Mode Every Time
“The smartphone market has evolved significantly over the past decade,” stresses Hwang Jeong-Hwan, the President of LG Mobile Communications Company: “LG customers expect our phones to excel in four core technologies – audio, battery, camera and display.” As a result, LG has also started to develop specialised and intuitive AI-based solutions for the features most commonly used on smartphones. The first result is the LG V30S ThinQ smartphone with integrat-ed Artificial Intelligence. The device’s AI camera analyses subjects in the picture and recommends the ideal shooting mode – depending, for instance, on whether it is a portrait, food, a pet, or a landscape. Each mode helps to improve the subject’s special characteristics, taking account of factors like the viewing angle, colour, reflections, lighting, and degree of saturation. The Voice AI allows users to run applications and customise settings by simply using voice commands. Combined with Google Assistant, searching via menu options becomes superfluous and certain functions can be selected directly. But LG wants to go further than simply equipping new smartphone models with AI. Depending on the hardware and other factors, LG is due to give some smartphones important AI functions via over-the-air updates in the future.
Every third wearable with AI
It is expected that AI wearables will give the stagnant wearables sector a much-needed boost. One in three wearables in 2017 operated with AI, according to market analysts at Counterpoint. According to Research Associate Parv Sharma: “Wearables haven’t seen the expected momentum so far because they have struggled on the lines of a stronger human computer interaction. However, the integration of Artificial Intelligence into the wearables will change how we interact with or use wearables. AI will not only enhance the user experience to drive higher usage of wearables, but will also make wearables smarter and intelligent to help us achieve more.” The analysts expect particularly high growth in the hearables -category – with devices such as the Apple Airpod or innovative products from less well-known brands like the Dash made by Bragi.
Wearables getting to know their users
Other wearables use AI, too. Machine learning offers far greater predictive potential in monitoring vital health signs. A company called Supa, for example, has developed clothing with integrated sensors. They capture a wide range of biometric data in the background, and provide personalised information on the user’s environment. AI enables Supa clothing to continually learn more about the user and so, for example, better understand their behaviour when exercising. Supa Founder and CEO Sabine Seymour claims that in 20 or 30 years, wearables of such a kind will be able to explain why the user has contracted cancer, for example – whether as a result of a genetic defect, due to environmental causes, or because of nutritional habits.
PIQ likewise combines its sports assistant Gaia with AI. It intelligently captures and analyses movements using specific motion-capture algorithms. Thanks to AI, Gaia detects its users’ movements ever more accurately, enabling it to provide personalised advice in order to optimise their training.
More Safety on the Bus
Intelligent wearable devices not only come in handy during sport and exercise, but also in more serious applications. For instance, NEC and Odakyu City Bus are partnering to test a wearable that collects biological information from drivers. The aim is to improve safety in the operation of the bus. In the pilot project, a wristband measures vital signs such as the pulse, temperature, moisture and body movements while the bus is being driven. The data is then sent off for analysis via a smartphone to an IoT platform, which is based on NEC’s latest Artificial Intelligence technologies. This is intended to visualise, monitor, and evaluate a wide range of health factors – for example, the driver’s levels of fatigue or changes in their physical condition which they may not be able to detect on their own.
Ayata Intelligence has developed an exciting solution: its Vishruti wearable smart eyewear helps people with visual impairments to find their way around their environment. To do so, it is fitted with a camera and a special, energy-efficient chip providing image recognition and deep-learning processes. This enables it to recognise objects and people’s faces. The system features a voice guidance feature, telling the user when a car is aproaching, for example, where a door is, or the name of the person in front of them.
Developments of this kind deliver the prospect that, in the years ahead, smartphones and wearables will continue to have an increasing influence on our lives, becoming guides and advisors in many different ways.
Developing autonomous vehicles
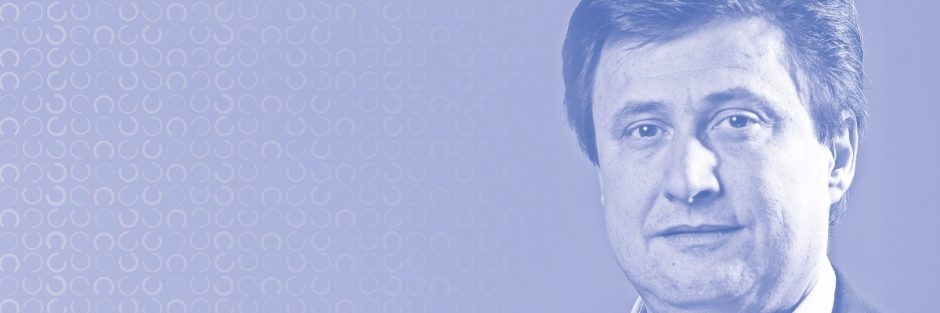
EBV Elektronik is Europe’s largest distributor of semiconductors and supplies all the electronics components that are necessary for developing autonomous vehicles. But its abilities go far beyond that, according to Frank-Steffen Russ, Vertical Segment Manager Automotive Europe at EBV. Through its network of partners, it has what it takes to cut down on development times and launch products on the market more quickly. At the same time, the experience that EBV’s experts are gaining in other applications is providing inspiration for new autonomous vehicle solutions.
The Quintessence: Hand on heart, would you travel in the kind of autonomous vehicle that exists today?
Frank-Steffen Russ: I would, and I’ve actually done it! Admittedly, it was a test vehicle at autonomy level 3 or 4, so it was some way off being a genuine robotic vehicle. But it’s important that we recognise the limitations of the technology these vehicles are using – they may be referred to as autonomous, but many of them are still undergoing trials and need more interaction with drivers in hazardous situations, for example. As users, we need to be aware of that fact.
Where do autonomous vehicles fit into EBV?
F.-S. R.: Autonomous systems are important parts of our Industrial, High-Rel and Automotive segments. Autonomous driving, flying and working all involve a range of disciplines, requiring a certain amount of thinking outside the box – and that’s exactly what EBV can deliver. We operate in all kinds of different applications: actuators, environment sensors, sensor fusion and connectivity, as well as cross-discipline areas such as on-board electrical system structures, security, and data management. That enables us to contribute our skills in significant ways across different segments. To take a few examples, our RF & Wireless segment provides products that support radar technologies for environment sensors, we create LightSpeed solutions for lidar applications, and we deliver high-end, camera-based technologies and solutions for sensor fusion as well as artificial intelligence. In the field of connectivity, our RF & Wireless and Security & Identification technology segments allow us to cover two major areas in vehicle networking. Together with the industry-specific expertise we have gained from working in these various market segments, we have what it takes to play a significant role in the evolution of autonomous vehicles.
Where do semiconductors come into autonomous driving?
F.-S. R.: Today’s semiconductor technology is advanced enough to support the systems required for autonomous driving. These systems can be used to establish both wired and wireless versions of high-speed data networks – which in turn form the groundwork for networked vehicles. State-of-the-art data centres are proving a boon for transport infrastructure planning. Thanks to high-performance processors, it is possible to simulate and optimise even exceptionally complex traffic situations. Vehicles themselves are also benefiting from increasingly integrated computer technology – in particular, multi-core systems that are available at an affordable price and with the same standards of quality found in automotive applications. By using cutting-edge semiconductors, driver assistance systems are already playing a major role in reliably preventing accidents and critical traffic situations.
Specifically, what kind of electronics components does EBV provide for creating autonomous vehicles?
F.-S. R.: Put simply, we offer a combination of semiconductors and our expertise. Autonomous systems need to deliver functions that people can rely on. And that means using components which meet the very highest standards of durability, fault tolerance and reliability – from simple diodes to sensors and all the way through to complex multi-core µC systems.
What else can EBV offer in order to help companies make their visions of autonomous vehicles a reality?
F.-S. R.: The structure itself is the cornerstone of our sales concept. As well as an ability to advise on technology, it is becoming increasingly important to be an expert in systems so that you can actually bring complex structures like autonomous systems to life. In this area, we help companies identify who the right system partners for them might be. These partners are able to provide hardware, software, design support, production services and much more in order to bring a product idea to fruition. As well as this, we work in partnership with semiconductor manufacturers so that we can support our customers with the right tools and reference platforms. This allows our customers to benefit from significant reductions in their development times – or take advantage of the latest technology for their product concept in cases where they are moving into a different field.
Networking is a huge talking point in areas like wearables. Are there any technologies this field is using that you think could be adopted in autonomous vehicles too?
F.-S. R.: Absolutely. One example comes from my own experience with hearing aids. Hearables are already very advanced, but the fact that vehicles are becoming increasingly quiet – especially electric cars – is posing a real challenge for them. So why not incorporate hearables into V2X communication and have intelligent warnings from vehicles relayed directly into the ears of pedestrians?
Something else to consider is the fact that the environment sensors in autonomous vehicles collect huge amounts of data that could be used in other applications – like weather, to take a basic example. In this case, a vehicle could be a sensor for precipitation that is about to occur in the local area – and that information could then be used in agricultural applications or for planning sports events, for instance. Networked vehicles will not only receive data about traffic flow – they’ll also be able to pass data from their surrounding environments directly onto road users in the vicinity, or even onto the Internet via a Cloud server, allowing the information to be relayed to a whole host of users.
As networking and autonomy are gaining a higher profile, so too is cybersecurity. What does EBV offer in this area?
F.-S. R.: Our segmented strategy means we have the potential to deliver best practice in every area. We provide support for cybersecurity through our Security & Identification technology segment. This allows us to ensure that our customers are always kept informed about the latest developments in technology as well as methods that are being applied.
What about communications equipment? Smart buildings have given rise to technology such as Li-Fi, a kind of wireless network based on lighting. Can you envisage using applications like this too?
F.-S. R.: If a vehicle has a wireless network – such as a WLAN – then the surrounding area undoubtedly has a similar connection. Let’s take the example of Dedicated Short-Range Communications, or DSRC, a system that exchanges data in real time: Li-Fi could definitely offer an alternative to this. In fact, LED daytime running lights, headlights and rear lights could already be used for this purpose. That would enable communication with traffic lights or traffic management systems without wireless interference in the viewing range.
How do you think autonomous vehicles will change mobility as we know it?
F.-S. R.: Autonomous vehicles will be essential if we want to achieve the WHO and EU aims of creating a safer traffic environment by 2050. I also believe that the period between now and then is when we will see changes occur. In ten to 15 years’ time, I think technology is bound to have reached level 5 of autonomous driving. However, it will be another few years after that before the technology moves from its initial premium segment to a level at which it is available to all.
Autonomous agricultural machinery will allow us to minimise soil compaction by harnessing concepts such as swarm farming – and that will mean significant changes for crop cultivation.
Where transport is concerned, autonomous heavy goods vehicles could propel truckers’ job descriptions from simply driving to mobile shipping, dispatching and monitoring of freight. The driver’s seat will become a mobile office.
Both aviation and shipping are already benefiting from the ability to manage traffic volumes more easily. Autonomous processes have been introduced in these areas, but volumes are only going to increase – and as that happens, we will need to move even further in the direction of full automation.
Autonomous driving | Start-Ups
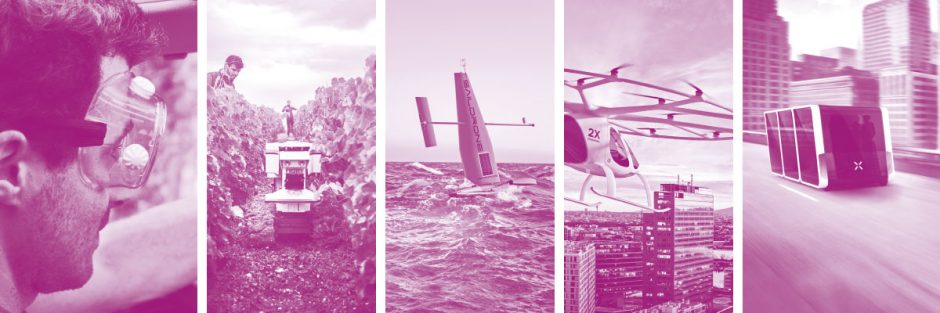
The possibilities offered by autonomous driving inspire the imagination. Lots of start-up businesses are working to develop innovative solutions from their ideas for application in a wide variety of fields. We present a small selection of interesting start-ups.
Hack your car yourself
US company Comma.ai has launched Panda, a type of dongle that can be connected to a car’s OBD-2 port. It enables all the data generated on board the vehicle to be read out. Together with the software tool Cabana, users are able to “hack” their own car and modify its systems. This might allow semi-autonomous functions such as automatic cruise control or brake assist to be programmed, provided the vehicle has the relevant sensors.
The device was developed by George Hotz – famous on the hacker scene for having been the first person to hack the iPhone, aged just 17, and subsequently doing the same to the Playstation 3 at the age of 20. Hotz originally wanted to market a complete “self-driving kit” for less than 1,000 dollars. But he gave the project up after the US Highway Traffic Safety Administration demanded testing and certification, which would have been too costly to undertake.
Hotz’s vision is to develop an open operating system for self-driving cars which, like Android, is capable of running on a wide range of devices, or in this case car models. He has developed a set of software solutions for the purpose: the “chffr” Cloud app is a dashcam app which can be used to record journeys. When linked to Panda, the app can also capture all the on-board sensor data and upload it to the Cloud. Comma.ai intends on using the data to enhance future autonomous driving functions. The benefits of that will also be felt by Openpilot, an open-source software program developed by Comma.ai, using which self-driving functions can be integrated into a car via the Panda dongle.
Comma.ai claims to have already collected data from over 1 million miles of driving, and to have the third-largest network of data suppliers after Tesla and Waymo. Hotz’s aim is to enable autonomous driving at level 3, based on selling low-cost hardware together with a monthly subscription to the Comma network. As the number of users increases, delivering data from ever more roads, the aim is eventually for the full-package solution to even enable level 4 or 5.
Help for wine-growers
French company Naïo Technologies specialises in robots for agriculture and viniculture. One of them is Bob, an autonomous robot running on chain tracks which helps wine-growers with tough tasks such as weeding and hoeing. Bob runs autonomously along the rows of vines, weeding both between the rows and among the vines, and moving from row to row with no human intervention.
Efficient collection of marine data
California-based Saildrone is developing and manufacturing a fleet of autonomous wind and solar powered water-borne vehicles. Their aim is to collect marine data cost-effectively on a large scale. This will be used to gain new findings for weather forecasting, managing the global carbon cycle, the fisheries industry, and climate change research. The Saildrones navigate autonomously to their assigned destination, hold position there, or run specific search patterns.
Flying Taxi
Volocopter is looking to fulfil every human’s dream of flying. The German company develops vertical take-off, fully electric multicopters for passenger transportation and to serve as heavy-duty drones. The technical platform enables the pilot to fly by remote control and fully autonomously. High redundancy of all critical components assures the high levels of safety of the flying taxis. The first Volocopter is scheduled to be licensed and launched onto the market in 2018.
Taxi, car and Bus in one
With Italian design and American know-how, Next is developing a smart road transport system based on a swarm of modular self-driving vehicles. Each of the electrically powered modules can connect and disconnect to and from other modules. The idea is that passengers will be able to order a vehicle using an app. If multiple modules are running on the same stretch of road, they will link together to create a vehicle chain. This will save energy and space on the roads.
(picture credit: Comma.ai; Next Future Mobility; Tien Tran/Naio Technologies; Volocopter; Saildrone)
Autonomous construction machines
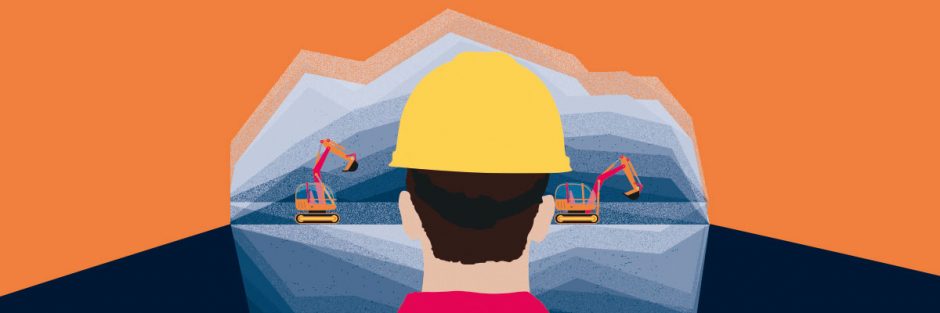
Autonomous construction machines like driverless dumper trucks have already proven their readiness for practical application at ore mines around the world. Now work is underway to produce autonomous excavators, though the technology is still in its infancy.
In the late summer of 2016, a project in the Swedish town of Eskilstuna provided a glimpse of the construction site of the future: at the site, Volvo Construction Equipment (CE) demonstrated how an autonomous wheel loader and a driverless dumper truck worked together. The wheel loader handled around 70 per cent of the volume normally loaded by a human-controlled machine. That’s a lot less – though the autonomous construction machines are able to operate round the clock. “The machines are able to perform the same task on a predetermined route time and time again, over protracted periods of time. But the technology is still in its infancy. We are working to devise solutions capable of delivering the safety and performance the market demands,” explains Jenny Elfsberg, Volvo CE’s Director of Emerging Technologies. “We still have a long way to go. So we don’t yet have any plans for implementation on an industrial scale,” she adds. The machine prototypes do not yet communicate with each other, for example. Yet that is vital in terms of avoiding collisions and simplifying efficient material flow. Nevertheless, Elfsberg is certain: “Autonomous machines will improve safety in a hazardous working environment, and eliminate the risk of accidents caused by human error. They will also perform repetitive tasks more efficiently and precisely than a human operator.”
24/7 operation: Drones supply topographical information, autonomous machines then move the required volume of earth precisely and highly efficiently on this basis.
Autonomous construction machines working together
Japanese manufacturer Komatsu is also working on autonomous construction machines. Its “Smart Construction” concept is already well advanced. The excavators and wheel loaders are only partially autonomous so far, however: the excavator operator now merely controls the boom, for example, while the bucket operates automatically. Its height and position are adjusted with the aid of cameras on the excavator and GPS sensors. The system “knows” how much earth has to be moved where. The necessary data is provided by thousands of aerial photographs captured by drones from US manufacturer Skycatch. The Skycatch software uses the images to compute a three-dimensional topographical model, accurate to within three centimetres. Based on several million measuring points, the volume of earth to be moved can then be calculated very much more precisely than using conventional manual methods.
Fully automated iron ore transportation
It will be a while before fully autonomous excavators are available. But fully autonomous trucks are already operating today: Australian mining company Rio Tinto, for example, introduced so-called Autonomous Haulage Systems (AHS) at its iron ore mines in the Pilbara region as far back as 2008. Today the company is the world’s largest owner-operator of autonomous trucks, running 71 autonomous dumper trucks. Actually, the word “truck” does not quite cover it: the Komatsu driverless dumper trucks transporting iron ore at the Yandicoogina, Hope Downs 4 and Nammuldi mines are the height of a three-storey building.
Higher productivity, less risk for staff
The dumper trucks are equipped with powerful computers which control the standard driving functions: starting the engine, accelerating and braking. The navigation system is GPS-based, and the trucks are fitted with distance sensors and collision-avoidance systems in order to identify and avoid any hazards. The vehicles are additionally monitored remotely from an operations centre in Perth, 1,500 kilometres away. Rio Tinto’s Mining Operations Manager at Yandicoogina, Josh Bennett, explains: “What we have done is map out our entire mine and put that into a system, and the system then works out how to manoeuvre the trucks through the mine.” The trucks are programmed to transport their loads as efficiently as possible. And they are a success: since 2008, the autonomous fleet has cut loading and transportation costs at the mines by 13 per cent. “Autonomous trucks reduce employee exposure to hazards and risks associated with operating heavy equipment, such as fatigue-related incidents, sprains and other soft-tissue injuries, and exposure to noise and dust,” says Yandicoogina Mining Operations Manager Josh Bennett.
The future of autonomous driving
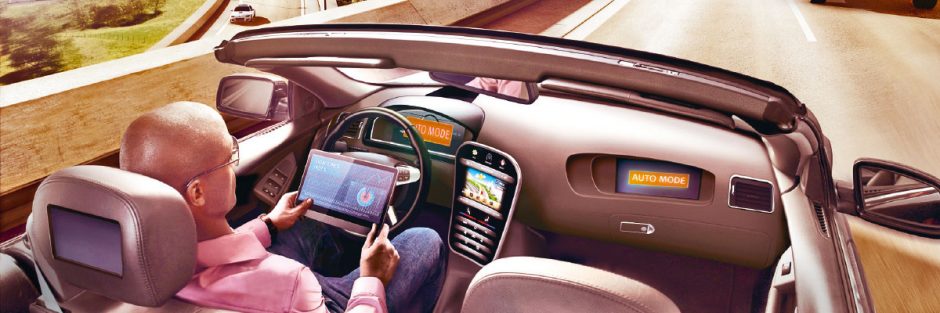
The future of autonomous driving will not only offer a completely new driving experience, it will also change the entire automotive industry.
Over 1.2 billion people spend more than 50 minutes a day in their cars – a large portion of that time, however, is spent in traffic jams. Wouldn’t it be nice if you could take your hands off the steering wheel during this time and get on with other things? This dream became reality in 2017: this year has seen the presentation of the world’s first production cars to be developed for highly automated driving. Vehicles can now take over driving functions such as parking or autonomously accelerating and braking in traffic jams.
Sharp eye for the surroundings
A basic requirement for automated driving is the ability to reliably perceive vehicle surroundings and evaluate them accurately on the fly. “In order for the system to acquire this information step-by-step, a range of sensors such as radars, cameras, and surround view systems are needed. The aim is to achieve an understanding of the vehicle’s surroundings which is as good as or better than a person’s own understanding. More range, more sensors, and the combination of acquired data with powerful computer systems will help to sharpen the view and is the key to achieving a consistent view of our surroundings,” says Karl Haupt, Head of Continental’s Advanced Driver Assistance Systems business unit.
British car-maker Jaguar Land Rover has taken this a step further. “We don’t want to limit future highly automated and fully autonomous technologies to tarmac,” says Tony Harper, Head of Research, Jaguar Land Rover. “When the driver turns off the road, we want this support and assistance to continue. In the future, if you enjoy the benefits of autonomous lane-keeping on a motorway at the start of your journey, we want to ensure you can use this all the way to your destination, even if this is via a rough track or gravel road.” For this purpose, Jaguar Land Rover has combined cameras, ultrasound, radar and lidar sensors in a concept vehicle. These systems not only enable a 360-degree view of the car’s surroundings, but are so highly developed that they can determine surface properties down to the dimension of a tyre width – even in rain or snow. Ultrasonic sensors can also detect the surface conditions within a range of up to five metres so that the vehicle can automatically adjust its traction and driving behaviour when switching from tarmac to snow or from grass to sand.
The car comes to the driver
The sensor system is only one part of the solution; the other is having the intelligence to generate commands from the data collected. This requires cars to be equipped with high-performance control units. The Audi A8 revealed in July 2017, for example, features a central driver assistance controller with deep-learning-based software which constantly froms an image of the surroundings from the sensor data during piloted driving. Daimler is also pushing to develop the software behind fully automated and driverless driving. In April 2017, the manufacturer entered into a development agreement with Bosch to bring fully automated and driverless driving to urban roads by the beginning of the next decade. The objective is to work together to develop software and algorithms for an autonomous driving system. The idea behind this thinking is that the car will come to the driver and not the other way around. Users will be able to conveniently order an automated shared car or robot taxi via their smartphone. The vehicle will then make its way autonomously to the user. “The car as we know it will soon be history,” says Dr Volkmar Denner, Chairman of the Board of Management of Robert Bosch. “Today you use the Internet to book a hotel room; in the future, you’ll arrange your mobility online as well.”
An autonomous fleet can effectively replace a much larger number of private vehicles.
Tapping into new business areas
Car-sharing is one of the big advantages of autonomous cars. David Alexander, Senior Research Analyst with Navigant Research: “Studies have shown that an autonomous fleet can effectively replace a much larger number of private vehicles in a city centre, which represents both an opportunity and a challenge for original equipment manufacturers (OEMs).” On the one hand, according to Navigant, 120 million autonomous cars will be sold between 2020 and 2035. On the other hand, automobile manufacturing is expected to reach its zenith and then decline because of shared cars. Therefore, automotive manufacturers should adapt their business models accordingly and sell additional value-added services, for example. “The more popular autonomous driving becomes, the greater the demand by users for services to meaningfully utilise the time freed up in the car,” concludes Ralf Gaydoul, Partner and Head of the Automotive Center at Horváth & Partners Management Consultants. “If the values were to be added up across all categories of need, this would give rise to a monthly amount of well in excess of 100 euros per driver.” Together with the Fraunhofer Institute for Industrial Engineering (IAO), Gaydoul has studied the willingness of motorists to pay for such services: according to the study, three-quarters of those surveyed would pay for value-added services. The willingness to pay for services is at its highest in relation to communication and productivity. “These services are the most heavily in demand in all three countries examined, though with different variations,” says Dr Jennifer Dungs, Head of the Mobility and Urban System Design Division at the Fraunhofer IAO. “For example, interest in in-car social media services is much higher in Japan than here in Germany (64 per cent compared with 23 per cent).”
(Picture Credit: Continental)
Retail: “Anyone who fails to adopt AI will die!”
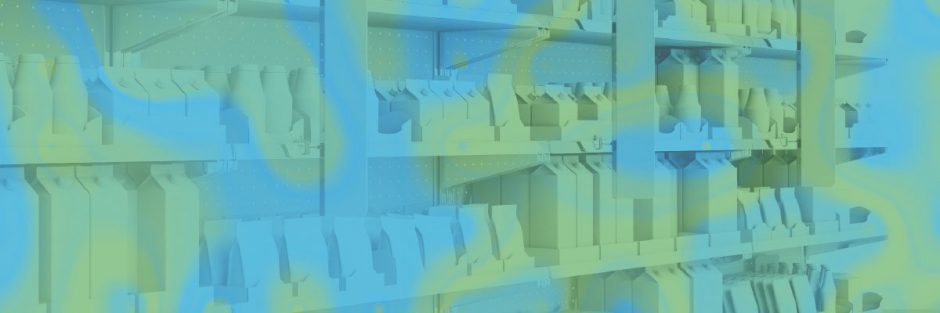
Applications of Artificial Intelligence are not just to be found in e-commerce. In high-street shops, too, selflearning algorithms are helping to balance supply and demand more closely, and understand customers better.
The retail sector involves a complex interaction between customers, manufacturers, logistics service providers and online platforms. To gain a competitive edge, retailers need to gauge their customers’ needs optimally, and fulfil them as efficiently and closely as possible. That means retailers have to make the right choices to find the ideal mix of partners. Self-learning algorithms and AI are opening up new dimensions in process optimisation, personalisation and decision-making accuracy.
Artificial Intelligence enables retailers to respond better to their customers’ needs and, for example, optimise their ordering and delivery processes.
Artificial Intelligence is a question of necessity
Prof. Dr Michael Feindt, founder of Blue Yonder: “Anyone who fails to adopt AI will die! But those who open themselves up to the new technology and make smart use of it will have every chance of achieving sustained success in the retail sector. For retailers, digital transformation through AI is not a question of choice, but of necessity. Only those who change and adopt the new AI technologies will survive.” One way that Blue Yonder is responding to that necessity is with a machine learning solution which optimises sales throughout the season based on automated pricing and discounting. The system measures the correlation between price changes and demand trends at each physical outlet and through each channel. Based on the results, the solution automatically sets prices to increase turnover or profit throughout the selling cycle, including the application of discounted pricing and running sale campaigns as appropriate. It analyses both historical and current sales and product master data, and enables hundreds of prices to be validated and optimised each day. Using such systems, retailers can meet consumers’ rising expectations and maximise their profits at the same time. According to Blue Yonder, this means profit can be improved by 6 per cent, sales turnover increased by 15 per cent, and stocks cut by 15 per cent.
Optimising processes in retail with AI
“Artificial Intelligence enables retailers to respond better to their customers’ needs and, for example, optimise their ordering and delivery processes,” says Stephan Tromp, Chief Executive Director of HDE, the German Retail Association. For example, retailers can use their suppliers’ data to measure performance and optimise processes. Combined with the data from their outlets and warehouses, they can also balance supply and demand more closely. For instance, intelligent forecasting systems learn from past orders, create buyer groups, and analyse seasonal effects. From their findings, they can forecast product sales volumes and ideally know before the consumer what he or she is going to order next. This means retailers can tailor their websites to the relevant product groups, trigger purchasing, top up stocks accordingly, and ultimately cut shipping lead times. As a result, bottlenecks in the supply of specific products can be predicted, and retailers can quickly identify which supplier is currently able to deliver top-up stocks of the required merchandise most quickly.
Keeping track of customers’ movements
AI not only has applications in retailers’ back-office operations, however. In the physical shops, too, deep-learning functions are helping to gauge customers’ behaviour. A company called Retailnext, for example, has launched an all-in-one IoT sensor which monitors customers’ movements when in the outlet: collecting their goods, trying on clothing, and walking around the shop. All those movements are monitored by a camera, and analysed directly in the unit with the aid of deep-learning functions. The data is then uploaded to the cloud in real time, so companies can gather valuable information on all the branches in their chain. “It’s precisely those projects that enable retailers to develop a deeper understanding of in-store shopping behaviours and allow them to produce differentiated in-store shopping experiences,” asserts Arun Nair, Co-Founder and Technical Director of Retailnext. “The more retailers know about what’s happening in store, the better.”
The Pioneer of Computer Vision
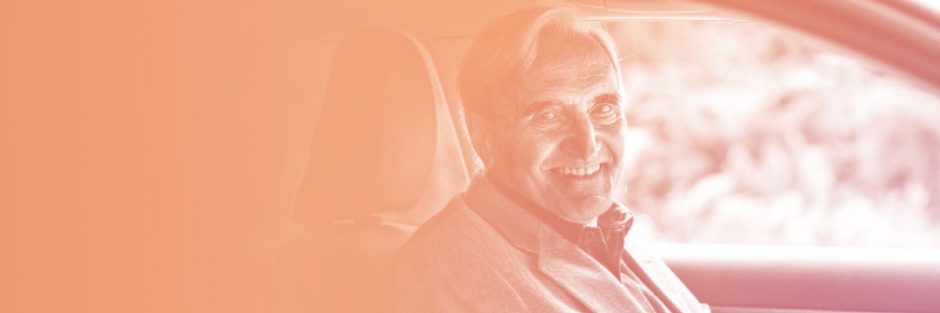
Prof. Dr Ernst Dieter Dickmanns is regarded as the pioneer of autonomous “seeing” cars. His “computer vision” methodology developed in the 1980s is still in use today in autonomous vehicles.
Prof. Ernst Dieter Dickmanns is more than a little envious when he sees the technologies that autonomous vehicle developers have at their disposal nowadays. “Computing power per microprocessor today is almost a million times greater than when we started. The volumes of computers and sensors are less than a thousandth of what they were back then.” “Back then” was the late 1980s, when Prof. Dickmanns, born in 1936, began developing an autonomous car. Right from the start, he worked on what is now commonly termed “computer vision”. “When you look at the role vision plays in biological systems, it has to offer major advantages for technical systems too.” That was the idea that led him to develop a method of teaching cars to see.
Seeing in real time, even without high computing power
“Even back in 1975, we were seeing computing power per microprocessor increasing by a factor of 10 every four to five years,” Prof. Dickmanns recalls. 1975 was the year when he – at the age of just under 40 – joined the University of the German Armed Forces in Munich. “It was likely that computing power would increase by a factor of a million by the time I retired. That was likely to be enough to permit video analysis in real time, which would be an entirely new technical accomplishment.” Dickmanns and his team began developing a method that was to turn “computer vision” into a reality before his retirement. In the 4D method, as he terms it, the data captured by cameras is digitised and processed by the computer merely as abstract lines with adjacent grey-scale areas. Rather than comparing the current image against the previous one, as was common practice at the time, he used motion models in three dimensions and integrated time (which is why the method is termed “4D”) in order to understand the observed process in the real world. These models predicted the expected features in the next image. As a result, much less data was created. Even using the processors available at the time, simplified scenes could be processed in 100 milliseconds – corresponding to real time in the automation world.
“I do consider safe autonomous driving on all kinds of high speed roads to be important.”
From Munich to Copenhagen – almost fully autonomously
The first vehicle fitted out in this way was running autonomously on blocked-off test routes as far back as 1987. The technical systems needed to do that literally filled cabinets – the first test vehicle was a Mercedes van with a five-ton payload: the VaMoRs (a German acronym standing for “test vehicle for autonomous mobility and computer vision”) provided sufficient capacity to accommodate a power generator and several metres of industrial control cabinets for the electronics. But just a few years later, the space taken up by the equipment had shrunk significantly. The “VaMP” vehicles from the University of the German Armed Forces and ViTA-2 from Daimler were both based on a Mercedes saloon car, and were results of the Prometheus project initiated by the European automotive industry. Both projects were supervised by Prof. Dickmanns. From 1993 onwards, the cars were able to run completely autonomously on roads with normal traffic. The crowning glory was a trip from Munich to Copenhagen. The test vehicle covered 95 per cent of the 1,700-kilometre journey with no intervention by the driver – changing lanes, overtaking other vehicles, and attaining a top speed of 175 kilometres per hour. It was a sensation back then – and remains a benchmark for modern-day self-driving cars. The 4D methodology is now an integral element of autonomous vehicles, and Prof. Ernst Dieter Dickmanns is acknowledged globally as the pioneer who taught cars to see.
Recipe for successful research
By the time he retired in 2001, he had developed many more solutions in the fields of computer vision and autonomous driving. Asked what his recipe for success might be, he lists four points: “The conviction that your idea is better than any other; acquiring adequate research funding; selecting appropriately qualified staff and doctoral students; and engaging widely in intensive dialogue with partners in industry as well as with international scientist colleagues at conferences.” He believes that anyone capable of bringing those attributes to bear on a project, alongside their own creativity, has what it takes to be a successful researcher and inventor. Not everything Dickmanns experienced in his scientific career was positive, however. Looking back, he offers a word of advice: “I would be even more cautious than I was in selecting industrial and scientific research partners, and make sure that key points are laid down in writing.”
No worries
But Prof. Dickmanns is far from being truly retired. He continues to follow developments in autonomous driving, gives lectures, and still has ideas about how to improve computer vision: “Given the current state of the art in technology, I would directly target solutions that have proven their worth in biological systems. One of the features I would install would be little eyes with dynamic direction-of-view stabilisation and control, fitted at the top of the A-pillar on the left- and right-hand sides of the car.”
Would he really be prepared to ride in a fully autonomous car today? His answer is clear: “If there was one, yes.” He can also imagine buying one of the new cars that are able to park themselves and drive autonomously in traffic jams: “Though I don’t place great value on parking. I do, however, consider safe autonomous driving on all kinds of high-speed roads to be important.
More efficient in a platoon
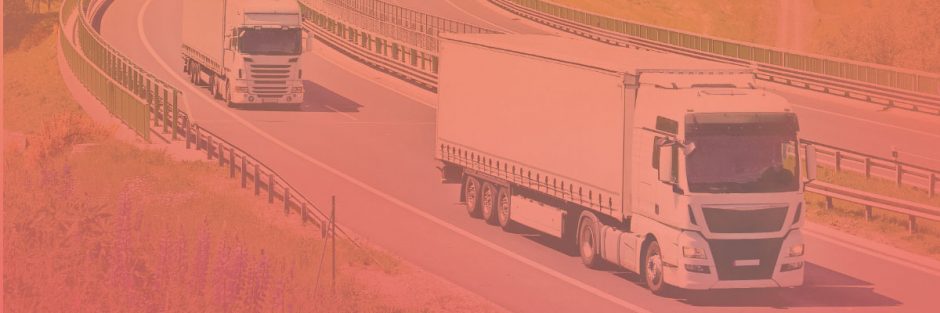
Lorries which automatically drive in convoy – known as platooning – are nearly ready for the market. The first fully autonomous test vehicles are already on the roads. Forwarders and manufacturers are hoping for a significant reduction in operating costs.
Strict legal regulations regarding driving times and safety regulations, personnel shortages, rising operating costs – the transport sector has been struggling with the same problems for a long time. Most of these could be solved through the use of autonomous lorries.
Platooning: The final development stage
“We are getting closer to a point where lorries will be increasingly controlled by technological intelligence, starting on motorways to begin with,” says Norbert Dressler, Senior Partner at Roland Berger and commercial vehicles expert. “This will represent the final stage in an incremental, 15+ year developmental process with a gradual reduction of driver engagement. While driver assistance systems such as ACC or Lane Keep Assist are already implemented in many trucks, automated vehicle operation will be possible in the final stage (level 5 or full automation) under all traffic conditions and potentially no longer needing a driver.” Each stage of higher automation brings with it higher system complexity and increasing costs, ranging from 1,800 dollars per truck to implement level 1 to 23,400 dollars per truck all the way to the final level 5. A key driver behind the high cost is software, which accounts for approximately 85 per cent of total cost. “All manufacturers are already working on new solutions to counteract the pressure of digitalisation and new competitors,” says Romed Kelp, expert for the commercial vehicle industry at global management consulting firm Oliver Wyman. “They all have prototypes on the road and are investing hundreds of millions in digital technologies.” Vehicles from major European manufacturers are already travelling in electronic convoys in initial inter-brand platooning demonstrations. Platooning is an on-board system for road transport where two or more truck-trailer combinations are driven one behind the other a short distance apart, using current technical driving assistance and control systems as well as car-to-car communication. Far from compromising road safety, this actually increases it. The distance between the vehicles is about ten metres or around half a second’s driving time. All the vehicles travelling in the platoon – the whole group of articulated lorries – are connected by an “electronic towing bar”. During the drive, the first vehicle sets the speed and direction of travel. The necessary commands are transmitted to the following vehicles via the car-to-car communication technology. These also send data back to the towing vehicle. A wireless connection with a frequency of 5.9 GHz is used for the car-to-car communication. Diesel consumption and CO2 emissions can be reduced by up to ten per cent.
-15 % fuel consumption and co2 emissions is the reduction through platooning
Source: Continental
Autonomous driving on the highway
The first fully autonomous test vehicles are already out on the roads as well: in fact, the world’s first autonomous HGV – the Freightliner Inspiration Truck – received a road-driving permit for the US state of Nevada back in 2015. As soon as the HGV has safely reached the motorway, the driver can activate the Highway Pilot system. The vehicle switches to autonomous mode and adapts to the speed of the traffic. Highway Pilot uses a complex set of cameras and radar systems with lane-keeping and collision-prevention functions to brake, steer and regulate the speed. This combination of systems creates an autonomous vehicle which operates safely under all kinds of driving conditions; for example, the HGV automatically observes the legal speed limit, regulates the prescribed distance from the vehicle in front and uses the stop-and-go function during rush hour. Highway Pilot does not initiate autonomous overtaking manoeuvres – these must be carried out by the driver. The same applies to exiting the motorway and changing lanes.
Platooning: A wide range of savings
Although the costs for the technology are high, the operating costs will decrease with autonomous driving functions: “Fuel and driver cost savings are the main factors in payback of the initial investments,” says Roland Berger expert Dressler. The industry does not have to wait until the final automation stage to achieve savings: fuel cost savings of around six per cent are already possible in level 1 (through platooning, for example). The main cost reduction will come into effect in level 4, where the driver can take required rest breaks during automated driving. Driver costs will thus drop by a further six per cent. In level 5, when long-haul lorries don’t require a driver at all, driver costs will be reduced by as much as 90 per cent. Further savings result from lower insurance costs as automated driving enhances safety: and the number of HGV accidents could drop by 90 per cent by 2040.
(Picture credit: iStockphoto Milos-Muller)
Challenges of autonomous vehicles
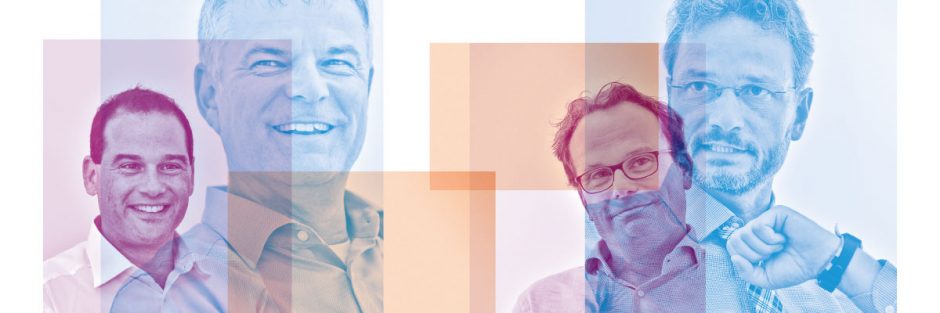
New regulations, questions about safety and cybersecurity, massive upheaval in the auto industry – the participants at the TQ round table listed many challenges of autonomous vehicles that must be overcome before the widespread use is possible. Nonetheless, they are certain that the time will come.
Why not?”, says Prof. Amos Albert, CEO of Deepfield Robotics, when asked whether he would have permitted a self-driving car to bring him to this meeting of experts. Prof. Eric Sax, Director of the FZI Research Centre for Information Technology in Karlsruhe, is a little more cautious: “I wouldn’t like to drive in a car without having a view of the road. The systems are not yet sufficiently sophisticated for that.” On that point, all the round-table participants are essentially in agreement. “At the moment, there is no vehicle on the market that has more than level 3,” according to Jens Kahrweg, General Manager EMEA at Savari. In his opinion, this is also because the legislation has not yet been adapted and liability issues must still be clarified. Prof. Sax also believes that the greatest difficulty is not in the technology, which is already available by and large, but more in making the autonomous systems safe: “With traditional methods, you look to see if the system is executing the action that was previously defined. But the plethora of situations that arise in daily road traffic can no longer be mapped with this method.” Albert agrees that it is simply not enough to calculate the failure probability of hardware and software in order to determine the safety of a self-driving vehicle. “When the ambient conditions are changing too quickly, the safety of the system cannot be calculated. We need other methods.” According to the CEO of Deepfield Robotics, one option is the proof test, for example allowing vehicles to drive as many kilometres as possible with the technology activated, and monitoring them to verify the reliability of that technology. Another is to define safe states and then ensure that the vehicle can return to such a state in the event of a problem. “This would obviate the need to calculate so many potential situations”, according to Albert.
“If autonomous driving is sold as a service, this will also reduce the entry barriers for users.”
Prof. Amos Albert, CEO, Bosch Deepfield Robotics
AI and V2X will be available in a few short years
However, the variety of possible real-world situations to which a self-driving car must respond is not just a massive challenge for safety and reliability. Prof. Sax explains: “As it is impossible to predict every event, a self-driving car needs artificial intelligence.” In this way, it can gain experience independently and learn how to respond correctly. The technology is still in its infancy, however. “But in just a few short years, this will no longer be an obstacle to progress,” asserts Prof. Amos Albert. “The computing power will then be available thanks to Cloud-based systems and deep learning applications.”
In the future, the knowledge gained would then be shared between the self-driving vehicles themselves. According to Jens Kahrweg of Savari, however, the vehicle networking required to do this still needs to be created. Savari was founded in 2008 and is in the process of developing the necessary V2X solutions. “Networking like this would act like another sensor and offer additional redundancy, in order to ensure the functionality of a vehicle.” While the future mobile communications standard 5G does not exist yet, this should not be a problem in five years’ time, according to Kahrweg. “How widely this is then rolled out and whether it can actually do everything it promises is something we are working on with the industry. But it should then be possible to network vehicles.” On the other hand, the modified Wi-Fi standard IEEE 802.11p has already been introduced. “The exciting question is whether both technologies will exist in parallel in the future – that’s what we’re hoping for at Savari. Because, among other benefits, having two systems increases the functional safety of self-driving vehicles.”
“Autonomous driving will -revolutionise entire business models.”
Thomas Staudinger, Vice President Marketing, EBV Elektronik
One of most important challenges of autonomous vehicles is cybersecurity
While networking and comprehensive communication do increase safety, they also simultaneously represent a risk, as pointed out by Thomas Staudinger, Vice President Marketing at EBV Elektronik: “Every point in such a network is a possible target for hackers. In a successful attack, not only could an individual vehicle be manipulated: an entire fleet could.” FZI Director Sax considers the over-the-air updates of future vehicles to be a particular risk: “Software is loaded onto the vehicle – and it will be a huge challenge to identify whether or not it is valid.” With hardware security building blocks, authentication and cryptography, a first firewall can be built. “We are also working on anomaly detection methods,” explains Prof. Sax. This is essentially based on the concept that the usual signals exchanged within the vehicle by the various components are known and can be checked for their plausibility. “The moment an unknown pattern emerges, the anomaly detection springs into action.” In the extreme case, the vehicle can then be brought into a safe state. “Ultimately the cybersecurity of the vehicle is a question of system architecture,” says Staudinger. “To create a solution here, however, the most varied of players must come together – by that I don’t just mean the car manufacturers or companies like Google and Facebook, but also all the very different stakeholders in such a network.”
“The networking of vehicles with one another has already begun; the missing connection, the networking with the infrastructure, will be made possible on a comprehensive scale by future mobile technologies.”
Jens Kahrweg, General Manager Savari EMEA
Upheaval for car manufacturers
Jens Kahrweg sees advantages here for car manufacturers who are just coming into the market: “For a manufacturer who has been producing millions of cars for decades, it is much more difficult and costly to completely overhaul their entire vehicle architecture to suit this new reality. Someone starting from scratch, however, can achieve this innovation leap much more easily.” The established car manufacturers will have to deal with significant upheaval as a result of self-driving cars – because the points of focus will change and software will play a very different role. Prof. Sax adds: “This is why the companies from Silicon Valley are walking all over us. But there is a limit to how much they can do that, because the traditional car manufacturers have other know-how – for example, in the fields of mass and variant production. However, the German car industry in particular will have to make some effort to tackle this learning curve. In future, it will no longer be the gap size that determines whether a car is sold.” Prof. Amos Albert does not believe that the demise of the traditional car manufacturers is imminent either: “Naturally, other companies are very strong in selected software technologies at the moment. But everyone is working at full throttle on new algorithms and at some point, this will even out and the old strengths will play a role again.”
On one thing, all the round-table participants are agreed: the success of car-makers will depend more on whether they can adapt their business models. “In the future, we will not be selling a car, we will be selling mobility,” says Thomas Staudinger of this development away from a product and towards a service. “Perhaps the manufacturers of the future will no longer sell their cars to private customers, but to fleet operators instead,” opines Jens Kahrweg, pointing to transport service operators like Uber or car-sharing operators like Car2go. “When a car-maker is manufacturing only for large customers, the OEM can quickly become a Tier1,” according to Staudinger. Kahrweg continues the line of thought: “And when the OEM develops into a mobility service provider, the suppliers can move up from below and take over the corresponding added value.”
“Electromobility with electrified, decentral actuators and auxiliary equipment will be a door-opener for autonomous driving functions.”
Prof. Eric Sax, Director, FZI – Research Centre for Information Technology
From niche to mainstream
And yet these huge changes will only be relevant if the autonomous car actually establishes itself on the market. The best way of doing that is for it to offer a financial advantage. “Of course, it is wonderful to have a goal of completely eradicating road deaths by 2050,” says Thomas Staudinger. “But ultimately someone must be paid to make autonomous driving a reality. Everything moves more smoothly when the bottom line shows a profit.” This is also why Prof. Sax is firmly convinced that autonomous driving will first be realised in the commercial vehicle sector. “According to estimates, a haulier or municipal service provider can save up to 50 per cent on their costs by introducing self-driving vehicles.” FZI has, for example, run the figures for the Stuttgart public transport service and calculated that it could save well in excess of 100,000 euros on personnel costs per year by automating the trips to the depot. “Autonomous driving will develop from precisely these niches, from applications with manageable scenarios. And that can be done right now,” says Sax.
Foundations of autonomous vehicles
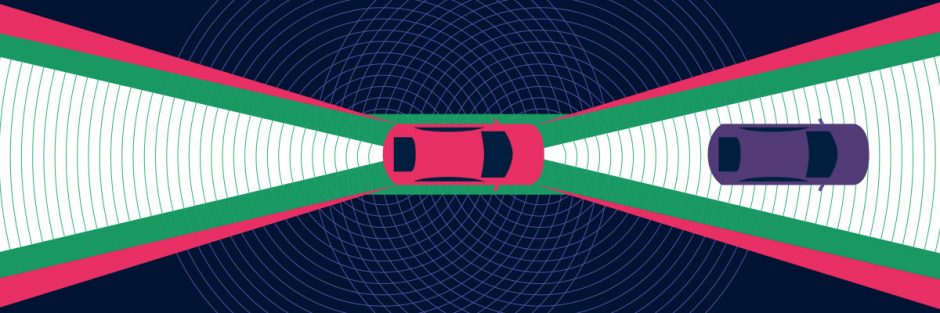
Sensors, computing power and the ability to learn are the technological foundations of autonomous vehicles. The more functions that are taken over by technology, the higher the level of automation – right up to the completely driverless vehicle.
The roots of autonomous vehicles reach further back than is generally assumed: as early as the start of the 20th century, Elmer Sperry developed the first control system controlled by a gyrocompass, enabling ships to be kept on course automatically. Then, in 1928, the first automated aeroplane control system, developed by Johann Maria Boykow, was showcased at the International Air Exhibition in Berlin. However, true autonomous driving requires far more than simply keeping the vehicle on a set course: the vehicle must be able to reach a specified destination independently, without human control or detailed programming. In doing so, it must be able to respond to both obstacles and unforeseen events.
From assistance systems to self-driving vehicles
The path to a fully autonomous system is gradual, with developments on a sliding scale. A worldwide system with six levels for classifying degrees of automation is now recognised throughout the world – it was defined, among others, by SAE International (Society of Automotive Engineers), but is now also used for other vehicle segments. According to this scale, level 0 corresponds to a vehicle without any assistance system, where the driver is solely responsible for all functions. At level 1, the first assistance systems, such as cruise control, support the driver. Partly-automated vehicles with parking and lane guidance systems, which can already carry out automated steering manoeuvres, constitute level 2. At level 3, the vehicle controls itself for the most part, and the driver no longer has to oversee the vehicle at all times. The fully-automated vehicles classed as level 4 can master even high-risk situations without human help, but are restricted to known sections of road. Only at level 5 do we find completely autonomous driving, in every environment and all situations. Within limited areas, such as agriculture, intralogistics, light-rail systems or mining, highly and fully-automated vehicles classed as levels 3 and 4 have been in use for quite some time. However, only level 3 cars are currently found on the roads. The first series-production cars that can cope without drivers in real road traffic, at least under specific conditions (level 4), are set to be on offer from 2020 onwards.
Sensors pick up on the surroundings
In order for a vehicle to reach its destination autonomously, it requires various capabilities: firstly, it must pick up on the environment through which it is moving – otherwise it would simply fall at the first hurdle. To prevent this, autonomous vehicles are equipped with a very wide range of sensors: ultrasound sensors are required for automated driving, particularly for detecting close-up surroundings up to six metres away and at low speeds, for example when parking. Radar sensors provide important information on the environment at a greater distance, through 360 degrees. The main task of a radar sensor is to detect objects and to measure their speed and position compared with the movement of the vehicle on which it is fitted. A relatively new addition is lidar sensors, which “scan” the environment with invisible laser light and can generate a high-resolution 3D map of the surroundings. Video sensors, above all in stereo-video cameras, supply additional important visual information such as the colour of an object. Each of these sensor systems has its strengths and weaknesses. In order to obtain an image of the environment that is as exact and reliable as possible, multiple sensors are used together in autonomous vehicles – depending on the application – and the data from these is “merged” or drawn together.
High-resolution maps via the cloud
As well as the ability to “see” the surroundings, an autonomous vehicle must also be able to navigate. Thanks to satellite navigation systems such as GPS, the vehicles know where they are currently located and can calculate their route based on this information. In doing so, they rely on high-resolution maps that are kept extremely up to date, with these maps not just showing the topology, but also incorporating current events such as traffic jams in a dynamic manner wherever possible. These maps can be stored locally in the vehicle or in the Cloud. In the latter case, a high-performance communication system is particularly necessary, so that the map data can be updated in real time. For example, the 5G mobile telecommunications standard can form the basis for this. It enables a “tactile Internet” that, in addition to transmission rates in excess of ten gigabits per second, guarantees an ultrafast response with a delay of less than one millisecond. With this type of networking, the almost unlimited resources of Cloud computing can be called on to carry out complex calculations involved in analysing the situation or route finding.
Learning as a basis for the correct response
After all, analysing the huge data volumes that are generated by the vehicle’s sensor systems requires considerable computing capacity, as does interpreting situations. Technologies that are grouped under “artificial intelligence” are becoming increasingly important. Machine learning, in particular, is an essential part of an autonomous system: only with this is it possible for vehicles to act intelligently and independently of humans. Through machine learning, autonomous systems can generate new knowledge from data that has been collected and provided, and are able to constantly extend their knowledge base. Without this independent learning, it would be almost impossible to specify appropriate reactions to all theoretically possible situations in programming.
Functional Safety – Emergency Plan
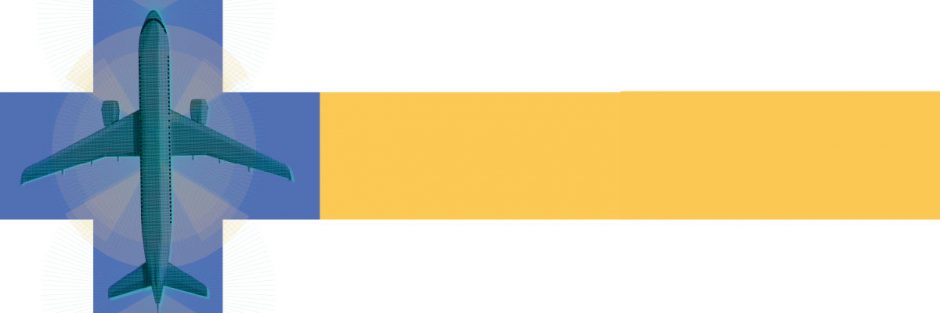
If people can no longer intervene in good time when a malfunction strikes, the technology needs to be especially safe. Special electronics and new testing methods are necessary to ensure the functional safety of an autonomous vehicle.
Confidence in the technology of autonomous vehicles is key to their success. The systems used need not only to be highly reliable, but must also be designed to prevent any failure endangering people or systems in case of a technical defect. “Functional safety” is an umbrella term for the relevant requirements and methods needed to accomplish this. “The automotive industry’s transformation is essentially asking the public to trust their lives to a computer and a machine,” says Zach McClellan, the former baseball star who currently runs the training division at US engineering firm LHP. “Functional safety in practical terms is defined as the steps engineers and organisations take to avoid failures that harm the public,” he adds.
No More Simple Switch-Offs
Achieving safety in autonomous vehicles requires more than simply switching off a system; drones would fall from the sky, while cars would lurch to an emergency stop. Consequently, critical systems are kept on temporarily, even after a fault has occurred. An emergency plan is needed; this must be defined as soon as an autonomous vehicle is in development. There are already standards today to define the required development and production methods for this. For instance, ISO 26262 applies to road vehicles, ISO 25119 to agricultural vehicles and ISO 15998 to construction machinery.
Safety Starts with the Electronic Components
Developing a functionally safe vehicle starts with the individual components, especially the semiconductors. These are built into the systems for environment recognition, and they contain the accumulated information from the various sensors, calculate the necessary control commands and determine how a vehicle is to operate. A malfunction could have disastrous consequences for the autonomous vehicle, for any occupants and for the environment. The semiconductor industry has therefore now created special semiconductors for use in automated driving with their architecture already developed based on an audited, ISO 26262-compliant process. The processors must provide a high degree of reliability and must continue to perform their tasks safely in the event of vibration, radiation (such as sun rays) or heavy temperature fluctuations, all of which are typical ambient conditions for their use in vehicles. Functionally safe processors self-monitor while executing processes. In these multithreading systems, each instruction is processed in parallel in two or more cores or processes. The results are then compared in real time by hardware logic. A discrepancy in the results means that a fault has occurred within one of the lines of calculation. If this is the case, the system issues a fault message or triggers a pre-set emergency action for this circumstance.
New Test Methods Needed
Safe electronics is one thing, but testing is also needed to demonstrate the functional safety of a vehicle. However, there are not yet any methods or test certificates to this effect. Testing agencies such as the TÜV are engaged in various projects to define new standards and testing criteria for autonomous driving, seeking to establish a basic safety level for the new technology’s practical application. In the course of this, it is impossible to reproduce on a test route all the potential situations encountered while operating an autonomous vehicle. As a result, additional, new methods are needed to test the effectiveness of the safety systems. Simulations play an important role in this and will be crucial as an accompaniment to real tests.
In addition, networked autonomous vehicles have to contain new technologies from different suppliers and industry sectors, which must be integrated into end-to-end systems and validated within the networked vehicle ecosystem. To this end, vehicle engineering service provider FEV has specially established a global Center of Excellence for the development of smart vehicles. Stephan Tarnutzer, Vice President of Electronics at FEV North America and Head of the Center, emphasises: “To keep control of the wide variety of interactions inside, outside and around the vehicle that occur as a result, it is essential to take the whole system into account at every stage of development.”
(picture credit: iStockphoto Edi_Eco)
Lidar – Laser replaces Radar
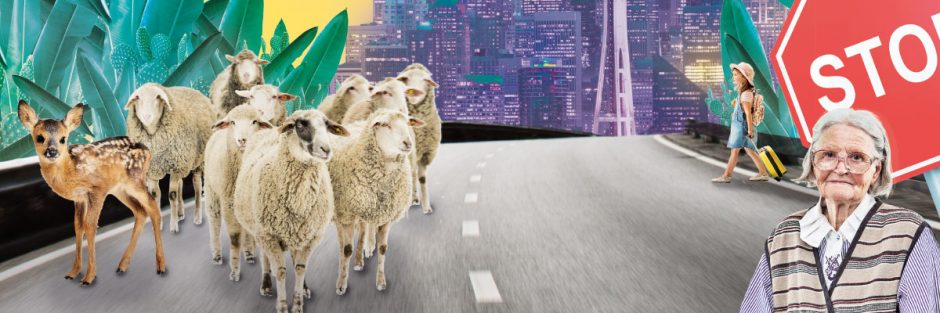
Detecting small or fast-moving objects – that is where lidar excels. The laser-pulse-based technology is increasingly being seen as a valuable addition to radar and camera systems.
Various sensor technologies are being used in autonomous vehicles in order to capture as broad an image of the surrounding area as possible, while minimising the risk of error. Whereas radar technology is based on high-frequency electromagnetic vibrations, lidar – as it is termed – works with laser beams to determine the distance of objects within close range of the vehicle. Lidar is primarily used to detect smaller objects on roads.
However, roads are not this sensor technology’s only area of application: “Everyone is talking about autonomous vehicles, but autonomous systems are also trending in the industry,” emphasises Dr Dirk Rothweiler, CEO at Berlin-based company First Sensor. The company produces what are known as avalanche photodiodes, which detect laser reflections in lidar systems. Lidar technology is already a technical standard in a whole host of industrial applications, as Rothweiler explains: “When it comes to industrial length measurement, our customers have been relying on lidar for years – to measure speed or to monitor safety areas for machines, for example. A growing field of application is autonomous systems. These are more readily implemented in industrial settings where surroundings and influencing factors are controllable.” Here, lidar systems make sure that mobile robots do not collide with the people working in production systems or that autonomous transport and logistic systems can take on complex tasks such as the loading of pallets, for example.
Laser diodes as a basis
Lidar stands for “light detection and ranging”. Its basic principle is time-of-flight measurement: a very brief laser pulse is emitted, makes contact with an object, is reflected, and then recorded by a detector. The laser beam’s travel time gives the distance of the object. Incredibly high-performance infrared pulse laser diodes with a short switching time form the technical basis of lidar systems. With an optical pulse power of approximately 25 watts and a spectral range of 905 nanometres, their pulses go virtually unnoticed by humans. They are completely safe for the human eye.
Scanning lidar systems use a laser beam to scan the area around the vehicle horizontally above a certain angular segment and create a high-resolution 3D map of the surroundings. Initially, the deflection of the laser beams in scanning lidar systems was implemented with mechanically driven mirrors. However, miniature laser scanning modules with integrated silicon-based MEMS micro-mirrors – which have appeared on the market in recent times – are more cost-effective, compact and robust. The micro-mirrors combine and fine-tune the laser beams. Up-to-date systems with solid-state lasers achieve ranges of more than 200 metres and a high resolution of less than 0.1 degrees.
An accompaniment to other sensor systems
The major advantage offered by lidar over other sensor systems is its extensive data acquisition: lidar collects more information from individual data points than any other system – x, y and z coordinates, period and degree of reflection to name but a few. Reflective surfaces such as road signs or even road markings provide a stronger signal, allowing lidar to detect such surfaces faster. A camera would also be capable of this. It could, however, be dazzled by backlight or sunlight and can only be used to a limited extent at night-time or in conditions of poor visibility. However, lidar systems also only deliver limited data during torrential rain. This is where radar impresses, as it is capable of “seeing through” objects. Yet to do this, radar systems do not possess the high resolution required to register objects when they are small or great in number and while moving at high speeds. Lidar, on the other hand, can also clearly distinguish small objects.
“Even the most aggressive start-ups do not expect lidar to be the silver bullet for the detection and perception of autonomous vehicles,” says James Hodgson, Senior Analyst at ABI Research. “Still, the natural characteristics of this technology are very well suited to that of radar and cameras, which until now have constituted core elements in obstacle detection.” In his view, however, the high costs in particular still prevent their wider application. Yet it is to be noted that the systems are becoming ever more affordable: Dutch company Innoluce, which is now part of Infineon, is planning to bring automotive lidar systems to the market for less than 100 dollars in the future.
(Bildnachweis: iStockphoto: Jevtic, Kaphoto, photobac, sturti)
Autonomous Bus – A Summary
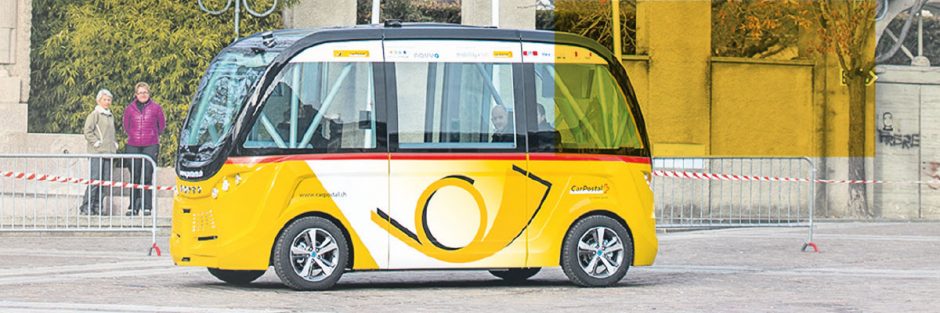
A year after the introduction of two autonomous bus shuttles in Switzerland, an initial conclusion can be drawn: levels of passenger acceptance are high, but the technology is still in its infancy.
A small Swiss city is taking a pioneering role in the use of automated buses. Since June 2016, two electric shuttles from French manufacturer Navya have been running in the centre of Sion in the canton of Valais – making their operator Postauto one of the first providers in the world to use automated buses for passenger transportation on public roads.
The trial in Sion will run for two years. A driver is still on board for safety reasons to monitor the system and intervene should this prove necessary. The aim of the pilot project is to test the new technology in the public arena in order that these experiences will inform the future development of the technology and its possible applications. Daniel Landolf, CEO of PostAuto Schweiz: “We want to learn from the new technology and the possibilities it will open up to us, so that we can develop new mobility solutions for the whole of the public transport sector.”
21,500 people in a single year
Approximately one year into testing, the two 11-seater electric shuttles, which are each 4.8 metres in length, have carried more than 21,500 passengers. In operation for 312 days, they have clocked up more than 4,500 kilometres. Despite passengers’ scepticism prior to boarding, after alighting, their reaction is very different, with the older generation being particularly impressed by the technology. With neither steering wheels nor pedals, the small -driverless buses look nothing like their normal -counterparts. Two stereo cameras towards the bottom of the windscreen monitor the road and are able to read traffic light signals and road signs. In addition, six lidar sensors scan the area around the vehicle through 360°, covering a circumference of between 50 and 100 metres. The SmartShuttle uses satellite navigation to find its way through the city centre. However, the route has to be driven manually first, with the autonomous bus being steered via a console. During this “exploratory trip”, the vehicle’s sensors capture its surroundings and use the information gathered to create a 3D map. After this, the shuttle is able to determine its own position for automated driving along the route and can detect obstacles. The vehicle runs as if on virtual rails. However, it is not completely autonomous. If it has to deviate from the programmed line (because of vehicles parked incorrectly, for example), the driver accompanying the vehicle will take manual control via the console. In addition, the shuttles are monitored by a teleoperator in a remote control centre who can intervene immediately to halt the bus at the next stop or send it to the charging station.
The Cloud at the wheel
The Cloud-based fleet management software by Swiss company Bestmile enables the driverless vehicles to collaborate as a fleet. It manages both timetabled journeys and chartered trips and is compatible with vehicles of any make. With modern algorithms for journey planning, automatic operations management in real time, route calculation and energy management, Bestmile brings together the “individual robots” to create an integrated, intelligent and flexible mobility system. The fleet management software also communicates with the shuttle’s control software in real time. The software in the bus steers the vehicle, sets its speed and applies the brakes. Although the buses are capable of a maximum speed of 45 km/h, they travel at no more than 20 km/h in Sion, with the average speed being just 6 km/h.
It’s still early days for autonomous buses
The past year has shown just how much potential automated buses offer. However, it has also shown that despite sophisticated technology, it is still early days for travel by automated buses. They are difficult and expensive to build and run and require strict monitoring. Manual intervention by the driver accompanying the bus is still a mandatory requirement, with eight out of ten manual interventions being necessary in order to avoid cars parked incorrectly. The system cannot operate in heavy snow, either. Plus, there has already been one accident: in September 2016, one of the two shuttles was involved in a minor collision with the open tailgate of a parked delivery van. Both vehicles involved in the accident sustained slight damage. However, lessons can be learned from mistakes of this kind: minor technical and organisational adjustments have been made by Postauto and the vehicle manufacturer Navya. The safety distance for cornering has been increased, for example, so that the vehicles are more sensitive in how they respond to obstacles and can stop more quickly.
Looking to the future
Tests are currently ongoing to widen the route network in Sion and make adaptations so that the SmartShuttles can be integrated into the overall mobility chain. In supplementing and improving the services available to customers over the last mile, the SmartShuttles would be meeting one of Postauto’s declared aims. Investigations are also under way to ascertain if the test phase can be extended in order to offer passengers further benefits including a dial-a-bus service.
(picture credit: PostAuto Schweiz)
3D Cameras in autonomous vehicles
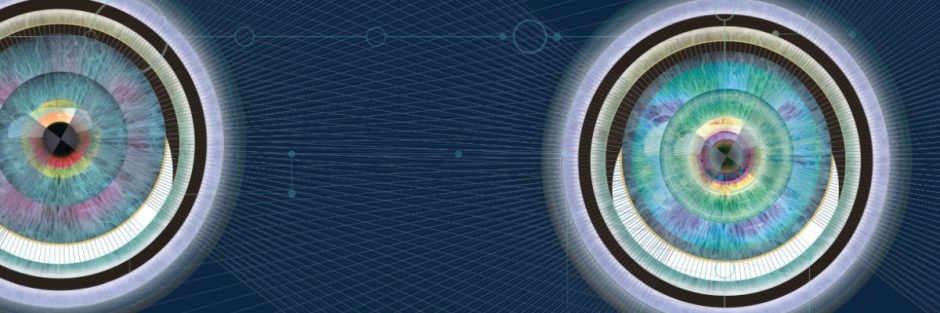
With today’s 3D cameras, autonomous vehicles can reliably detect obstacles in their path. Modern systems deliver information so accurate that it can even be determined whether it is an object or a person causing
an obstruction.
Precise detection of the surrounding area is a crucial basis for the successful application of autonomous vehicles. Alongside sensor systems such as lidar, radar and ultrasound, 3D cameras can also be used to enable an autonomous vehicle to precisely recognise its own position and that of the objects around it at any time in order to facilitate the accurate coordination of manoeuvres. A variety of technologies are employed.
Stereo cameras simulate a pair of eyes
In the case of stereo cameras, two digital cameras work together. Similar to the stereoscopic vision of a pair of eyes, their images enable the depth perception of a surrounding area, providing information on aspects including the position, distance and speed of objects. The cameras capture the same scene from two different viewpoints. Using triangulation and based on the arrangement of pixels, software compares both images and determines the depth information required for a 3D image. The result becomes even more precise when structured light is added to the stereo solution. Geometric brightness patterns are projected onto the scene by a light source. This pattern is distorted by three-dimensional forms, enabling depth information to also be determined on this basis.
ToF cameras measure the speed of light
Another method is time-of-flight (ToF), which determines distance based on the transit time of individual light points. Achieving centimetre accuracy calls for rapid and precise electronics. Time-of-flight technology is highly effective in obtaining depth data and measuring distances. A time-of-flight camera provides two types of information on each pixel: the intensity value – given as grey value – and the distance of the object from the camera, known as the depth of field. Modern ToF cameras are equipped with an image chip with several thousand receiving elements. This means that a scene can be captured in its entirety and with a high degree of detail in a single shot.
More precise information by combining cameras
While the basic technologies are already in use to a large extent – in car assistance systems, industrial robots, on the land as well as in drones – research is looking to further optimise systems. 3D cameras that need to function in vary-ing lighting conditions are hindered by large pixels and therefore lower resolution. To offset this, work is under way to develop a piece of software which can fuse together 3D camera images with those of a high-resolution 2D camera, for example. This will enable high-resolution 3D data to be obtained, which can then be further processed with the help of artificial intelligence: thanks to high-resolution images, the detected objects can be classified – and it is a safe bet that a person will not be mistaken for a rubbish bin. Other projects are also using colour cameras to enable classification to be made according to colour as well as shape.
Eagle-eyed vision
A further aim is to reduce the number of cameras required. Until now, a whole host of cameras and sensors all around the vehicle, or a rotating camera on the roof, was needed to generate as wide a viewing range as possible. At the University of Stuttgart, the widening of a single camera’s field of view was modelled on the eye of an eagle. An eagle’s eye has an extraordinary number of photoreceptors in its central fovea – the part of the eye where vision is at its sharpest. Additionally, eagles have a second fovea at the corner of their eye, allowing for sharp peripheral vision. Scientists have developed a sensor which all but emulates an eagle’s eye across a small area. Research was carried out under the umbrella of the SCoPE research centre at the University of Stuttgart and was able to be put into practice thanks to the very latest in 3D printing technology from Karlsruhe-based company Nanoscribe. The researchers in Stuttgart imprinted a wide range of micro-objective lenses with different focal lengths and fields of vision directly onto a high-resolution CMOS chip. The smallest has a focal length equivalent to a wide-angle lens, two lenses have a medium field of view, and the largest lens has a very long focal length and a small field of view just like a typical telephoto lens. All four images created by the lenses on the chip are electronically and simultaneously read and processed. In the process, a small computer program constructs the image to display the telephoto lens’ high-resolution image in the centre, and that of the wide-angle lens on the very outer edge. Owing to the fact that the sensor system as a whole has dimensions of only a few square millimetres – the lenses have a diameter in the region of just one to several hundred micrometres – a new generation of minidrones could also be set to profit from the technology alongside the automotive industry.
Autonomous vehicles are the future
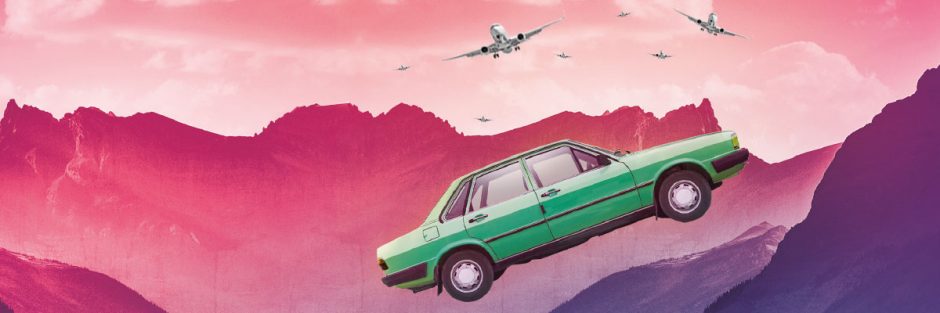
Autonomous vehicles will change all mobility sectors. The reasons for removing the driver, helmsman or pilot are diverse, and range from increased safety to greater efficiency and less environmental impact.
Self-driving cars, unmanned aircraft or driverless tractors – autonomous vehicles stopped being merely an idea on paper a long time ago. Now they are becoming increasingly “active” among us, at least in the form of prototypes: machines that act autonomously, independent of human commands, and make the “right” decisions, at least in everyday situations. New sensor technologies, networking possibilities and self-learning algorithms make it possible for the new vehicles to react quickly and sensitively to their environment, taking a wide range of data into consideration.
Driving without human control
According to the definition of the German Specialist Forum for Autonomous Systems (Fachforum Autonome Systeme), an autonomous vehicle exists when a system can reach a specified destination independently, irrespective of the driving or environmental situation in question. In line with this definition, the ability to learn is not a prerequisite, but rather a possible characteristic of autonomous vehicles. With regard to automated road traffic, this objective is achieved if, for example, the on-board system takes over the task of driving “completely, on all road types and in all speed zones and environmental conditions”. This means that driverless cars make decisions and take on tasks in unstructured environments, without a human driver exercising a control function.
46.8 % of people worldwide would allow their children to be driven by an autonomous car.
Source: Cisco Systems, 2013
New business models are opening up
At present, vehicles with these capabilities are not only being developed for the roads, but also for deep-sea journeys and flights in the upper atmosphere. They do not just replace the driver, helmsman or pilot, but have the potential to create completely new business models worth many billions: autonomous drones that can remain in the air for months and bring the Internet to 4.5 billion people who were previously offline are just one example of this.
Autonomous vehicles will probably lead to the most significant revolution in road travel. Automotive manufacturers can establish innovative business models based on this new technology, for example with entertainment offers or individually customised servicing packages that pilot the vehicle into the manufacturer’s own workshops.
At the same time, companies must adapt to shorter development cycles and new competitors from the IT and high-tech sector. Above all, however, there will be significantly less revenue from car sales: analysts from Barclays investment bank are working on the assumption that, thanks to car sharing and autonomous taxis, sales of private cars will fall by up to 50 per cent in the next 25 years. The companies still have time to prepare for these upheavals, as completely autonomous vehicles are not expected in complex road traffic until around 2030. However, in controllable environments such as agriculture or mining, self-driving vehicles are already in use today.
Autonomous vehicles do not just replace the driver, helmsman or pilot, but have the potential to create completely new business models worth many billion.
Diverse benefits
There are a wide range of arguments in favour of autonomous vehicles, with improved use of infrastructure and a reduction in the number of accidents being the key points of focus for road transport. After all, 90 per cent of all road accidents are due to driver error. The same is true of air travel – electronic systems remain alert 24 hours a day and the current microprocessors can react approximately 1,000 times faster than humans in dangerous situations. However, the staffing costs for pilots are also a reason for using autonomous aircraft. The same argument can be made in the construction sector for driverless vehicles – after all, autonomous excavators and HGVs will save up to 90 per cent of labour costs. At the same time, the machines can remain in use 24 hours a day, without the breaks that a person would require. Within the logistics sector, fully automated HGVs could therefore enable better usage of fleet capacity and make supply chains more efficient in the medium term. A shortage of workers is a further reason for fully automated vehicles: this is true of both HGV drivers and for maritime shipping. At the same time, autonomous vehicles also reduce the impact on the environment: with construction and agricultural machines, it should be possible to reduce the amount of CO2 produced by up to 60 per cent. What is more, completely new methods of farming can be implemented in the agricultural sector, making it possible to significantly reduce the use of spray agents and protect the soil.
Who is responsible?
However, there are still a number of ethical, legal and social questions that must be answered before we can make use of these advantages: after all, who is responsible for the “actions” of autonomous vehicles if users themselves are not involved in the vehicles’ decisions, or only marginally involved? And in the event of conflict, what criteria should machines use to “decide”, and who will determine said criteria? Nevertheless, the experts are certain that these questions will be clarified and that autonomous vehicles will trigger a revolution in mobility in the near future.
2 m is the maximum distance a current NASA Mars Rover is able to travel in one go before it has to stop and recalculate. (Source: NASA)
57 % of people worldwide would ride in a driverless car. (Source: Cisco Systems)
1⁄3 of land area in big US cities could be freed up by autonomous parking. (Source: www.2025ad.com)
50 % of a ship’s operating costs are down to the crew. (Source: Moore Stephens LLP)
70 % is the potential increase in global farming yield through the use of autonomous vehicles, drones and other related technologies. (Source: Goldman Sachs)
Thanks to quick-responding electronics, the safety clearance of connected truck convoys can be reduced from 50 to 15 m (Source: www.2025ad.com)
Evolution of mobility
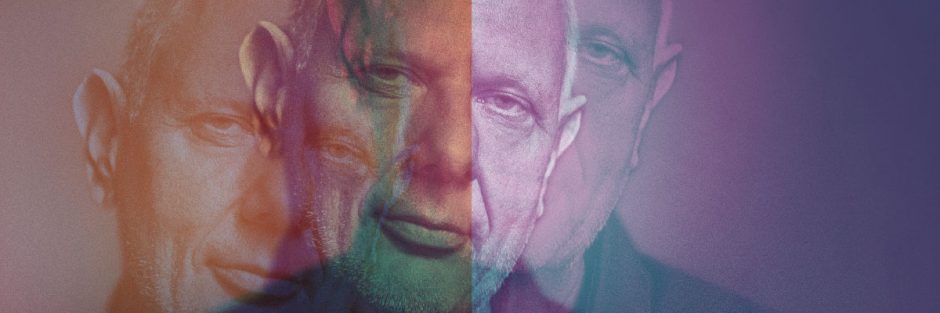
Try to see into the future and you’ll receive a vision that is fraught with uncertainty. “It’s not possible to say exactly what will happen in the future, of course – either in general terms or focusing on specific aspects,” says Matthias Horx. “But you can shine a light on it.” Horx is considered to be the German-speaking world’s most influential futurist and trend researcher. At the turn of the millennium, following a career in journalism, he founded the Zukunftsinstitut, which advises numerous companies and bodies on the directions that the future is set to take. Today, he dedicates himself to his life’s work of transforming futurology from the format in which it emerged in the 1960s and 1970s into a specific discipline of consultancy, which can be used by companies, areas of society, and the political sphere alike. He adopts an evolutionary approach to the technologies of the future rather than believing them to exist at the end of a straight line, with the new simply replacing the old. His view is instead that human skills and needs will develop alongside what technology is able to provide. As a result, the future will emerge as these aspects combine and recombine with one another – with combinations breaking through and others being left by the wayside. Working with Germany’s ADAC motoring association, the Zukunftsinstitut has now shone its light – or should that be headlight? – on mobility. Its study into the evolution of mobility, “Die Evolution der Mobilität”, looks ahead to 2040 – and forecasts significant changes affecting this field. We are on the cusp of a new multi-mobile age, the study states. Matthias Horx sat down for an interview with The Quintessence to explain exactly what the study predicts and what role autonomous vehicles are set to play in this.
“If you really want a good insight into what the future is likely to hold, then you need to understand how social and technical processes interact with one another.”
The Quintessence: Your work at the Zukunftsinstitut examines all kinds of different areas. Do you find trends cropping up in more than one area at the same time?
Matthias Horx: Looking at fashion-based trends, socioeconomic trends, technological trends and megatrends, those are all long-term drivers of specific changes such as globalisation or urbanisation. Then there are metatrends – these have a long-term impact on the way we evolve as a whole. Once upon a time, the famous 1960s futurist Herman Kahn talked about a concept called a long-term multifold trend. This could be seen as an evolutionary principle in and of itself – a kind of theory of everything that revolves around the world becoming more and more complex. It’s also important to note that the term “complex” shouldn’t be confused with “complicated”!
What role do technological developments play in your predictions of the future?
M. H.: Technology is an important driver of change, but it’s not the only thing doing that. If you really want a good insight into what the future is likely to hold, then you need to understand how social and technical processes interact with one another. Not every innovation will make a breakthrough on the market. Right now, for example, we’re seeing a lot of hype around robots with increasingly human features. However, I predict that they’ll be a flop. Although we’re fascinated by the idea of creating artificial humans, at the same time it makes us uneasy – that’s a natural response. So ultimately, we’ll end up sending our metal and plastic friends back to the lab. I do think that industrial robots will make huge inroads in factories everywhere, however.
What does the future of mobility look like?
M. H.: In general, mobility is set to dematerialise and become more attuned to certain cultural aspects. Nowadays, it’s still perceived as somewhat functional – something to take us from A to B. In most cases, we travel simply because we need to cover a certain physical distance. That’s starting to change, however. Increasingly, we are gaining the possibility of crossing the miles using virtual technologies and being in places thanks to telepresence. At the same time, we are seeing the emergence of a new kind of nomad – people who are always on the move and have forged a lifestyle out of this. Mobility has become a lifestyle.
Generally speaking, where do autonomous vehicles come into this – including ships, planes and trains?
M. H.: All these areas are going to feel the effects of autonomous driving – it’s a question of when, not if. It could be that ships are actually even better suited to full automation than cars are, because road traffic is exceptionally complex to navigate. In the first 20 to 30 years, I believe we will have a role as pilots in our vehicles; it will be around 2040 or 2050 before we see full autonomy making a genuine breakthrough. The technology will need to develop rapidly at that point, though – a hybrid situation in which some vehicles are still being driven and some are autonomous is unlikely to work. However, I believe that most trains will already be running as automated systems by 2040: the technology involved in this is much easier to master.
In your study into the evolution of mobility, were there any results or findings that surprised you?
M. H.: It was surprising to see just how open people are to an alternative kind of mobility. When it comes to cars, people have extremely divergent opinions. On the one hand, you have the 40 per cent who are still dyed-in-the-wool car fans – for them, owning a car is inextricably linked with identity and they are still zealous advocates of the combustion engine. On the other hand, there are people who drive frequently but hate being stuck in all that traffic. Diesel cars and their impact on the environment are a factor for this group too. Particularly in cities, there are a lot of people who no longer own a car and are quite happy with that – in fact, they see it is something liberating and an essential part of their quality of life.
Did the study only consider road traffic?
M. H.: It was a significant part of the study, of course, although we did seek to gain an all-encompassing understanding of mobility processes. In today’s world, road traffic primarily refers to cars, but that view isn’t set to last. Cities are on the brink of becoming Copenhagenised – which means that cars will soon become bit players and streets will be the domain of pedestrians, cyclists and mobile traders instead.
You also talk about multimodal mobility in this context. What does that refer to?
M. H.: To put it simply, it means creating seamless networks of various modes of transport so that they can be used around the clock and combined with one another. What’s admittedly great about cars is that you can just throw your luggage in and then go from A to B. However, we can make that happen in the future by combining other modes of transport too – let’s say, electric scooters and delivery bicycles, or trains and drones. And it won’t involve too much expense or effort to do so.
Many analysts believe that the automotive industry will reach its zenith within the next 10 to 15 years. When 2040 comes around, what role do you think cars will be playing then?
M. H.: I would be careful about making any pronouncements on this subject. Technically, the automotive industry has already reached its zenith – it can’t progress any further as it currently stands. But it’s also set to change. It’s going to merge with the energy and computing industries, and when that happens, we’ll have to start calling it something different.
What impact will autonomous vehicles have on our lives?
M. H.: We do lots of things in the car that we used to do in the office or at home – we sleep there, we live there, we work there, and so on. However, that also raises difficult questions: for example, does driving count as time spent at work? The answer isn’t clear. For many people, the reason they love driving is that it has a certain relaxing quality to it – provided traffic is flowing smoothly, at least. You can sit and listen to music, or you can have a snooze if you’re a passenger. It doesn’t have to be a very intense activity, and that’s where many people believe the sense of relaxation and autonomy comes into it. Self-driving cars could eliminate that feeling, so many people are instinctively against them. Then there are the people who don’t like autonomous driving because it removes the aggressive aspects of the activity. It means no more tearing along the roads and controlling the wheel; no more flashing others on the motorway – so the question really becomes a case of where the outlet for all their pent-up rage is going to be.
But won’t autonomous vehicles afford us more freedom too?
M. H.: It’s true that they will free us from the need to spend whole years of our lives behind the wheel of a car. But you can already do that simply by taking the train. The fact remains that many people enjoy being slaves to their vehicles – in the same way that they seem to enjoy buying furniture from Ikea and then building it themselves. We’re actually quite happy to be dependent on technology and give our freedom a back seat. Why else would people put up with the bizarre experience of sitting in traffic jams?
Hand on heart, what developments have personally surprised you the most, because you simply didn’t see them coming?
M. H.: Trump.
One of your books is called “Guide to Future Optimism”. Why should we take an optimistic view of the future?
M. H.: Being an optimist is quite a silly thing in and of itself, because it usually means taking a naïve approach. The smartest people among our ancestors weren’t optimists – if they had been, they would have been dead before the point at which they were able to procreate. I see myself as a possibilist. I think in terms of possibilities, and I choose the best ones as visions of the future that we should strive towards.
Mobility 2040:
Digital organisation and individual networks“In the future, the challenges that mobility is set to face will be in individual, intelligent networking,” said President of the ADAC motoring association Dr August Markl upon the publication of the Zukunftinstitut’s study “Die Evolution der Mobilität” (“The Evolution of Mobility”). “Our models of mobility are becoming more multifaceted and complex. What we are on the brink of is not a disruptive mobility revolution, but rather evolution and change that will become increasingly deep-seated.”
The Zukunftsinstitut’s study exploring the future states that our need for security, good health, an unspoilt environment and a generally high quality of life is set to become even more important. Digitalisation will be one of the pillars of tomorrow’s mobility solutions. Futurists believe that these growing mobility requirements will also bring about changes in the way in which we use cars as we approach the year 2040. They expect to see a much stronger network developing for the different modes of transport that move individuals around. Digital platforms will make it possible to integrate public transport infrastructures and sharing services.
New lifestyles, brought about through changes such as the way in which we work or our increasing lifespans, are also set to have a long-term impact on individual patterns of mobility. By 2040, the researchers believe that personal mobility will be found in all kinds of different formats to suit different groups – ranging from IT-savvy “mobile innovators” to “silver movers”, the over-75s with their own distinct set of challenges.
Job killer or Job driver?
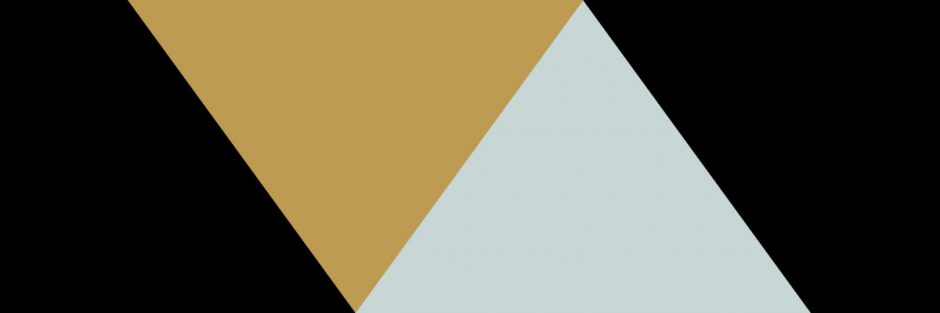
Will robots kill jobs, or create new ones? The issue is a controversial one among experts. We investigate the differing views.
The introduction of robots will turn much of our world of work upside-down,” claims Dr Martin Sonnenschein, Partner and European Head of Management Consultancy A.T. Kearney. “In 20 years’ time, almost half the current jobs in Germany will have been replaced by robots which can do them more efficiently.” Dr Sonnenschein made that claim back in late 2015 at the presentation of a survey conducted by his organisation as part of its social initiative titled “Germany 2064 – our children’s world”.
According to A.T. Kearney’s analysis, a quarter of all job profiles in Germany – that’s over 300 – are at high risk of being lost to automation in the next two decades. The potential impact on the labour market is dramatic, because the sectors concerned employ 17.2 million people – 45 per cent of the total workforce. However, even a job that is highly likely to be automated will not necessarily disappear completely.
A survey conducted in the same period by the Ing-Diba bank highlights the issue in concrete figures: It claims that 18.3 million jobs are threatened by advancing technology in Germany alone. Both surveys transfer the methodology applied by economist Carl Benedikt Frey and engineer Michael Osborne to the US labour market in 2013 to Germany. According to Frey and Osborne, 47 per cent of jobs in the USA are highly likely to be “robotised”. The World Bank has also calculated the prospects for India and China using the same methodology: Its conclusion is that in India 69 per cent of jobs would be under threat, and in China even 77 per cent.
Low-paid jobs are particularly impacted: According to the “Economic Report 2016” published by the Council of Economic Advisers (CEA), 83 per cent of such jobs are at risk of being replaced by robots with artificial intelligence in the long term. But it is not just unskilled workers who need to be worried: Market analyst Gartner forecasts that more than 3 million workers worldwide will be supervised by “robo-bosses” by as early as 2018. Those “robo-bosses” are not robots in the conventional sense, but software systems which measure and monitor employee’s performance data – more efficiently and accurately than a human manager could do.
One thing is certain: Automation will have a major impact on the world of work. “That means we need to be willing to embrace change, and show flexibility,” warns A.T. Kearney’s European Head Dr Martin Sonnenschein. “Anyone capable of doing that will be in a position to profit from these dramatic changes too – whether as an employee or an employer. We can wait, and be rolled over by automation. Or we can engage courageously with the change, seeking flexibly and with curiosity for the new opportunities it will open up.”
The German Engineering Federation VDMA regards the job losses forecast by Ing-Diba as a clear misjudgement: It claims the survey overestimates the potential of robot chefs, humanoid hotel robots and parcel delivery drones for example. A survey conducted on behalf of the German Federal Ministry of Labour also contradicts Ing-Diba’s claims: After investigating the automation potential of activities – rather than jobs – it shows that no more than 12 per cent of job profiles are highly likely to be automated.
The fact that worries about robots taking over human jobs are unjustified is demonstrated by the German automotive industry: Between 2010 and 2014, the numbers of industrial robots in use increased by 15 per cent, to 92,000 units. In the same period, the number of people employed in the industry rose by 10 per cent, to 775,000. Moreover, it is claimed that the collaborative robots now coming onto the market will open up lots of new potential applications.
The Boston Consulting Group also sees automation as a positive development. It reasons that, while some jobs are disappearing, entirely new ones are being created. According to its calculations, the loss of some 610,000 jobs will be countered by around a million posts that could be created by 2025. “New technologies such as Augmented Reality and robot-aided workstations can even help low-skilled workers to integrate back into the labour market,” asserts Markus Lorenz, BCG partner and expert in Industry 4.0 (also referred to as Smart Manufacturing).
Workers, in Germany at least, are aware of the changes happening in the world of work. Rather than regarding them as a threat to their jobs, they are focused primarily on the benefit such technologies will bring to their everyday working lives. That is demonstrated by a survey from Accenture Strategy published to coincide with the World Economic Forum in Davos in early 2017. Frank Riemensperger, CEO of Accenture in Germany, Austria and Switzerland, nevertheless has a warning: “Digital change can only succeed if companies invest more heavily than they have to date in helping their workforce develop new skills and acquire additional qualifications. That is less about retraining them in new jobs than ensuring their skills keep pace with new technologies. Ultimately, digitisation will not result in job losses, but it will impose new demands on workers. The importance of continuous professional development will rise greatly, not least because the half-life of our knowledge is becoming ever shorter in view of the rapid advances in technology.”
Creative freedom
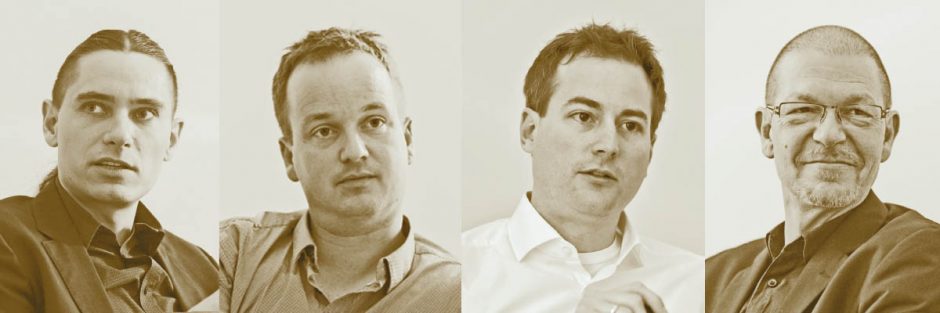
With new technologies and ever expanding capabilities, robots will have gained a foothold in every area of life in just a few years. That not only offers companies creative opportunities for new business models, as the participants in the TQ Round Table believe, but will also deliver more creative freedom for working people.
In 10 years at the latest, there will be robots in every area of our lives,” asserts Roger Seeberger, CEO of Jinn-Bot. “In the past, robots worked only in static settings, now we are seeing them progress to dynamic environments,” Seeberger continues. “It all started with the collaborative robots from Universal Robots, but nowadays it extends even further. In Switzerland, for example, the assistance robot Pepper from Softbank Robotics is deployed to accompany customers around supermarkets.”
Focus on collaboration
Lasse Kieffer, who worked at Universal Robots until a year ago, illustrates the trend by quickly sketching out a pyramid: “Initially – at the top of the pyramid – robots were mainly used in the automotive industry. Having proved themselves in that environment, they expanded into other sectors,” Kieffer adds, drawing his pen down towards the pyramid’s base. “Collaborative robots then enabled still wider applications, in which conventional robots had not previously been viable. That trend will continue, and the capabilities of collaborative robots will expand further.” For Kieffer, who is currently preparing to launch his own business, it is a natural development that is creating an ever increasing market for robotics applications. Dr Claus Lenz, Co-founder and CEO of Blue Ocean Robotics Germany, also believes that collaboration is the key word in relation to the ongoing development of robots. He sees three trends: “Firstly, robots and humans are coming ever closer together in industrial manufacturing. Secondly, we are also seeing that convergence in our day-to-day lives – with robotic vacuum cleaners and personal assistance robots for example. And thirdly, robots will start to collaborate more closely among themselves – multiple robots with different capabilities working together to perform a shared task.“
“The robotic sector now has technologies at its disposal that did not yet exist just a few years ago”
Jim Welander, System Field Applications Engineer, EBV Elektronik
Ever easier to control
Jim Welander, a Field Application Engineer for EBV Elektronik in Denmark specialising in support to robotics firms, sees developers of new robotics solutions also benefiting from advances in areas such as consumer electronics and the automotive sector: “As a result, the robotics sector now has technologies at its disposal that did not yet exist just a few years ago.” Moreover, robots are becoming ever easier to control, as Welander points out: “In earlier times you needed a degree in engineering to program a robot. Today it is something that every child learns in school – in Denmark at least.” People are generally becoming increasingly comfortable in the use of high tech. “Young people have no problem with it at all,” Roger Seeberger agrees. “But for older people it’s different. It will take a generation until they are also able to handle robots.” Claus Lenz disagrees. For him it’s just a question of usability. “If we can build a robot with a naturalistic interface, that is easy to understand, we will be able to integrate robots into older people’s lives too.” Roger Seeberger is entirely in agreement with that view – as long as the robot is working flawlessly. “But we are not yet currently advanced enough for non-technical people to deal with a malfunctioning robot.” Dr Lenz does not see that as an obstacle however: “When a dishwasher stops working, we call a service engineer. The same could happen in future with home robots. It might even create a new business model …”
Prices of the required electronics will fall
But how far can collaboration between humans and machines really go? “As far as a sex robot,” Lasse Kieffer asserts. Though Claus Lenz poses the question of what exact “collaboration” means: “Would the robot merely respond to people? Then we’re talking more about interaction. Genuine collaboration would mean people and robots pursuing the same goals.” But it will be a while yet before such robots actually appear on consumer markets. “The technology for collaborative, mobile systems already exists today, but it is still too expensive for domestic users,” says Jim Welander. The necessary sensors, such as laser scanners and lidar systems, as well as high-tech electric motors, are major cost drivers of multi-functional mobile assistance robots, which various forecasts estimate will be as much as 25,000 dollars. Yet it is precisely the advances in consumer electronics and the applications of electronics on this mass market that will drive down prices of electronic components dramatically in the years ahead. That is something all the round table participants agree on. Lasse Kieffer points out that the automotive market will also ensure the availability of cheaper systems for sensing the environment around robots for example: “The trend towards ever more autonomous cars will see increasing numbers of systems such as lidar and radar being fitted. That will ultimately make them cheaper for use in robots.”
“The ISO/TS 150GG technical specification standard for collaborative robots itself took six years to get published.”
Lasse Kieffer, ISO expert and future entrepreneur
Robots are becoming part of the Internet of Things
“But there will be no single robot capable of performing every task in the near future,” states Claus Lenz. “Rather, we will have specialised small-scale devices in our homes that are able to interact.” The Blue Ocean Robotics CEO foresees a combination of physical machines and smart Internet of Things (IoT) devices which will communicate with each other and share tasks among themselves. “Robots might provide a way of interlinking the digital and real worlds.” Roger Seeberger agrees: The training robots his company builds can be controlled on a smartphone using an Android app for example. “The boundaries between IoT devices and robots are now historical; they no longer exist today in fact.”
But that fact entails not only advantages – because robots are exposed to the same risks over the Internet as any other connected device. Cybersecurity is thus also an issue for robot developers too – or at least it should be. “Even though current robots actually process their data locally, and the Cloud is only used to distribute it, the same security standards should apply to the Internet connection as for a PC or a mobile device. That also includes regular security updates, which is an area where the robotics industry still needs to raise further awareness,” Claus Lenz warns.
Simulating the human brain
Cybersecurity is just one of the challenges that modern robots have to confront however. Another is intelligence: robots need it to move around an unfamiliar environment, or in order to communicate with people. That is not a problem in terms of the electronics, in Jim Welander’s view: “Chips featuring processor cores offering sufficient computing power for artificial intelligence are increasingly coming onto the market. The problems will rather lie in the software and related algorithms.” Claus Lenz stresses that for the time being, systems first have to be developed to interconnect different knowledge sources, and to combine machine learning with the data. Properly functional voice recognition also requires a certain level of intelligence, as the Blue Ocean CEO goes on to explain: “Context is key, because spoken words can have different meanings.” But it will doubtless be years before a robot actually acquires human-like intelligence. Roger Seeberger is convinced that robots which develop their own consciousness will most likely be created more by chance than anything else: “We don’t yet know much about our thought processes, so there is a lot of speculation, and a variety of theories and methods are being tried out. I suspect that at some point someone will suddenly say: oh, there it is! I believe it is much more important to consider whether we really should have robots with their own consciousness – but that is more of a political question.”
“The standards for secure internet connections should also be applied to robots.”
Dr. Claus Lenz, Co-founder and CEO, Blue Ocean Robotics
Machine safety also necessary for robots
As robots become more intelligent – or rather, become more autonomous – new challenges are also arising with regard to the safety of people and of the environment in which the robots operate. “Artificial intelligence is about making autonomous decisions that are hard to predict,” comments Welander. “But in order to build a safe robot you must be able to predict how it will behave in given situations.” There are of course also regulations governing robots within the machine and functional safety standards drawn up by the International Organization for Standardization (ISO), as Lasse Kieffer explains: “There are committees covering industrial robots, as well as non-medical personal care robots, and service robots – including robotic vacuum cleaners for example. But the standards being developed will be very wide-ranging and generalised.” Kieffer worked intensively on the subject of functional safety for collaborative robots during his time with Universal Robots, and also attended relevant ISO meetings. So he knows that it normally takes several years for an ISO standard to be agreed and adopted – which means it might well have by then already been overtaken by new technology. That does not mean that robots not conforming to the standards are unsafe, as he stresses: “Nevertheless, the standards are helpful in designing new, safe robots.” He sets out his recommendation for how to build a truly safe robot with artificial intelligence: “There will be a number of simple safety features ensuring that the robot can be turned off.”
Improved understanding between humans and robots
An off-switch of such a kind is the simplest example of an human-robot interface. But our future mechatronic helpers will have much more complex solutions at their disposal for communicating with people and understanding their commands. “Present-day programming solutions for industrial robots are not an option for use in the domestic sector,” says Claus Lenz. “We need systems by which a robot can learn based on gestures, imitation, or speech.” Context-specific understanding of language will be key. Moreover, studies conducted by Blue Ocean Robotics have determined that being able to predict robots’ movements is important for trouble-free collaboration between robots and people. “If a robot’s movements simulate those of a human, and are not technical, the person concerned is more likely to grasp where an object is being passed for example,” Lenz explains. However, Roger Seeberger believes that it will not just be about robots adapting to human communication: “Living with robots will also alter the way we communicate – you only have to think about the impact smartphones have had on our communications.”
“There will one day be robots with their own consciousness.”
Roger Seeberger, Managing Director and Developer, Jinn-bot
The world of work will change
Robots will not only change our communication habits; they will also have an enormous impact on the world of work. “There have been numerous studies on the subject,” Claus Lenz reports. “A recent survey by McKinsey predicts that there will inevitably be changes as a result of increasing automation. But the use of robots will not necessarily lead to job losses; in fact, new jobs might be created. What kind they will be is not yet foreseeable – perhaps a robot’s safety assistant?” It is an idea that Lasse Kieffer likes: “Then we will not have robots assisting us, but people assisting robots!” Jim Welander is also optimistic, recalling the changes brought about by smartphones: “Just consider how many people are working in app development today – a job that no one would have dreamt of a few years ago.” Roger Seeberger expects to see much more profound effects on the world of work however: “It is essentially an ethical and political question, because we are going to have to reshape our society.” As one example, he cites the unconditional basic income, on which a referendum was held in Switzerland in 2016. “In future, the quality of work will no longer be expressed in hours, but in the extra time that people have to be creative.” Of course new technologies such as robotics entail risks, as Claus Lenz concurs: “People are naturally worried that their lives are going to change. But we can either worry about technology destroying our life, or we can work to ensure technology brings positive change to it.”
A robotic helper around the home
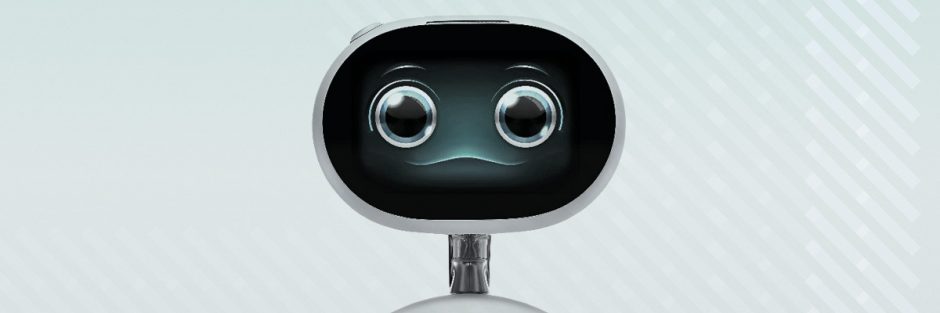
In Zenbo, the Asus corporation has launched a robot for the whole family onto the market. It plays with the children, gives reminders of appointments, takes care of the elderly, and watches over the home when the family is out.
The way the little guy looks at you with his big eyes, you just has to love him: Zenbo, the household robot from Asus. He has been available to pre-order in Taiwan since January 2017, though the rest of the world will have to wait a while yet. While revealing ASUS Zenbo, Chairman Shih said: “For decades, humans have dreamed of owning such a companion: one that is smart, dear to our hearts, and always at our disposal. Our ambition is to enable robotic computing for every household”. At an expected price of less than 600 dollars, the signs indiate that the company’s ambition can be achieved.
An untiring carer for the elderly
For that price, buyers get a robot designed to be a helper, entertainer and companion for families. Zenbo moves autonomously around the home on a spherical body, interacting with the human residents by speech and via his round “head”, featuring a display which shows different faces depending on his current “mood”: blushing red when embarrassed; eyes sparkling when excited; or laughing heartily. Although intended for the whole family, Asus’s robot is focused primarily on the older generation: Zenbo is designed to look after their health and help them connect to the digital world. He watches over the home and in an emergency, such as if an elderly person suffers a fall, alerts a designated relative by sending a signal to their smartphone. The relative can then control Zenbo remotely, and use his built-in camera to check that everything is in order. A touch-screen, displaying Zenbo’s “face”, also enables elderly family members to carry out a range of online tasks with ease – such as making video calls, using social media, shopping, or streaming movies and TV shows. The robot is voice controlled.
Educational playmate
The family’s children will also enjoy Zenbo – as a fun playmate, with a distinctly educational touch. He can entertain them with interactive stories, and teach them games that encourage them to be creative and think logically. With his stereo sound system, the electronic companion can play the children’s favourite songs – and even dance along with them too. At bedtime, Zenbo will read the little ones a good-night story, complete with pictures displayed on his monitor face. And he will also monitor the light in the room at the same time.
Interface to the Smart Home
But Zenbo is also a household helper, designed to make the lives of all the family easier based on a range of capabilities. He can be linked to many so-called Smart Home functions, to control the lights, the TV or the air-conditioning system for example. The family can also check the monitor to see who’s calling when the doorbell sounds – and issue a voice command for Zenbo to unlock the door. In the kitchen, the household helper can read out recipes, or act as a voice-activated timer, so the residents are not distracted from their cooking. When the family is out, the robot’s camera monitors the home, and the residents can view the captured images on their smartphones.
Open for third-party apps
In order to continually expand the range of tasks that Zenbo can perform, Asus is committed to cooperation agreements with partners and developers in a wide variety of sectors – from education and local public transport, to building cleaning services. The aim is for all of them to expand the capabilities and potential applications of the household robot with complementary apps or services. To that end, the company has launched a dedicated developer program, and offers third parties the relevant development tools. For the version sold in Taiwan, for example, Asus worked together with the country’s police service. The result is an app by which family members can contact their local police station in an emergency, talking to an officer via Zenbo’s videophone system.
Robot on-Board

Cruise company Costa Crociere has taken five robots on-board to entertain its passengers. They not only provide the guests with up-to-date information, but are also able to detect how they are currently feeling.
On the Costa Diadema, the flagship of the Costa Crociere line, guests are now welcomed on-board and provided with assistance by five “Pepper” model robots. The Pepper is the world’s first robot capable of read human emotions and needs, and interacting proactively with people.
“Ahoy” in English, French and Italian
The robots developed by Softbank Robotics operate on the Costa Diadema’s seven-day cruises around the western Mediterranean. Their mission: to entertain the guests on-board, and make the cruise even more unforgettable than it would otherwise be. The robot was first launched in Tokyo in 2014. It incorporates lots of innovative features, and has high-level communication skills. It is able to converse faultlessly in English, French and Italian, and can recognise voices and faces. “By our deployment of emotionally interactive robots on-board our cruise ships, we have once again demonstrated our great innovative capability. For us this is a major step towards the digital future of our cruise brands. I am certain our guests will love Pepper,” comments Michael Thamm, CEO of the Costa group.
Recognising human emotions
Pepper is the first humanoid robot in the world capable of recognising the key human emotions: joy, sadness, anger or surprise. It can also smile, furrow its brow, as well as interpreting the tone of a voice. Pepper is also able to interpret the words a person uses, as well as non-verbal signs such as a nod of the head. By combining all this information, the robot can determine whether its human counterpart is in a good or bad mood. The robot’s 2D and 3D cameras enable it to find its way around, and object recognition software allows it to recognise faces and objects. A wide range of other sensors complement the camera systems, including ones to measure distances to obstacles for example. Among these are two ultrasonic and six laser sensors, as well as three obstacle detectors in the little robot’s legs. Pepper also has tactile sensors in its hands which it uses when playing games or interacting socially.
Entertaining and informing
The guests on-board the Costa Diadema encounter the robots in various areas of the ship. Pepper is not only able to answer questions put to it, but can also interact proactively as soon as a passenger approaches. Pepper dances and plays with guests, and poses for selfies with them. The robots are designed not only to make the guests smile, however, but also to provide them with useful information about their journey and the amenities available on-board. The robots know everything about the ship’s restaurants, bars and shops, and can give details of shore excursions and on-board activities. They can also provide information on upcoming destinations and the ship’s route. And Pepper also asks the passengers on the Costa Diadema what they think about the cruise and the on-board amenities.
Anyone who visits EBV Elektronik’s stand at optoelectronic trade fairs can experience Pepper in action; giving presentations and joking around with visitors. Sebastian Hülck, Director Segment Lighting at EBV, talks about his experience with Pepper.
How long have you been using Pepper?
Sebastian Hülck: We rolled him out for the first time at the LED Professional Symposium 2016 in Bregenz, Austria. I believe we were the first ones to have used Pepper at a European trade fair.
How difficult is it to adapt Pepper for your own applications?
S. H.: It does take a great deal of effort, that much is true. The firm NoDNA in Ismaning took care of that for us: they are one of the authorised dealers for Softbank Robotics/Aldebaran. It took around three months to get Pepper up to his current standard – and to equip him with the various gimmicks which he has mastered to date.
So Pepper can’t be programmed quickly using an app?
S. H.: No. There are specific development environments for Pepper which you have to get to grips with first, such as the Choreographe Suite. In addition to that, the robot is a very complex piece of kit – with his eight-core Intel Atom platform, 20 motors and array of sensor systems. As an operating system, the machine runs the Linux-based Qi OS.
Does Pepper actually recognise human emotions?
S. H.: If you interact with him on a one-to-one basis, that actually works pretty well. On the other hand, he is overwhelmed when surrounded by lots of people. In that scenario, he doesn’t recognise individual people and no longer listens to one specific person. What’s more, the speech engine could still be optimised further. Amazon Echo or voice recognition systems from Apple or Google work much better, in fact.
How do people react to Pepper?
S. H.: The robot makes a fantastic impression on visitors. At the trade fair in Bregenz, our gimmicks made Pepper a real crowd-pleaser. Anyone who is interested in doing so is more than welcome to experience our robot for themselves: either at the trade fairs we attend with our Lighting Segment or in our “LightSpeed Experience Center” in Poing, Germany. Here, we demonstrate future technology related to optoelectronics. With his six lidar sensors, Pepper fits into this environment perfectly – that includes our focus on retail solutions this year.
Help to get people back on their feet
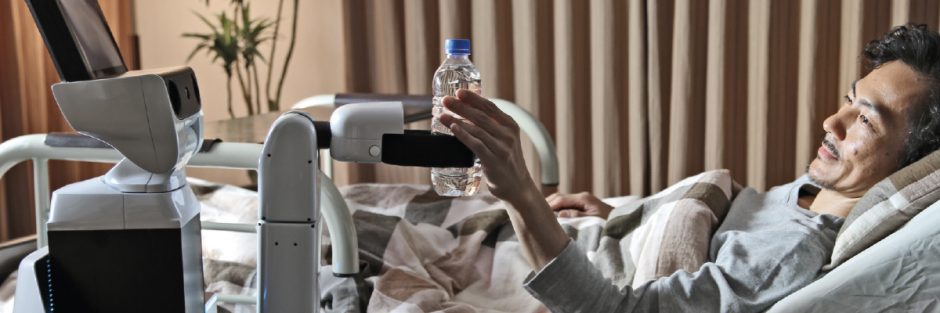
Robots are one of the potential solutions to the issues of an ageing society. They can assist nursing staff, and enable disabled people to live a more independent life. US veteran Romy Carmago had the opportunity to try out Toyota’s HSR assistance robot in his day-to-day routine. There is still a lot of development work to be done, however, before the technology is fit for everyday life.
Since his third tour of duty in Afghanistan, Romy Carmago has been paralysed from the shoulders down. But Romy has not given up. Although the doctors told him he would never again be able to breathe without a ventilator, he now doesn’t need one. The doctors have also told him he will never walk or be able to use his arms again, but he is sure he will do it one day.
Getting back on their feet thanks to technology
Using technology if necessary: Romy Carmago has established the “Stay in Step” rehabilitation facility near to his home city of Tampa, Florida. The centre offers programmes and provides technical aids with which paraplegics can stand again and perform exercises. It literally helps people get back on their feet.
In late 2016 Romy had the opportunity to try out a totally new piece of kit: the “Human Support Robot” (HSR) from Toyota. The Japanese group’s research institute has supported Romy Carmago’s rehab centre from the very beginning. So it was no surprise that it chose to trial its new care robot, launched in 2015, as a means of assisting paraplegic patients at the centre.
Help for an aging society
The HSR was originally designed to help care for the elderly. It is a lucrative market, particularly as the World Health Organisation (WHO) forecasts that 22 per cent of the global population will be over 60 by the year 2050. The trend in Japan is even more dramatic: almost 40 per cent of its population is predicted to be 65 or older by 2060. So demand for long-term carers is correspondingly high. And that is also the reason why so many care robots are being developed in Japan.
This is also where the versatile HSR seeks to help. By taking over everyday tasks, it enables people who need care or rely on assistance to continue living an independent life at home. The lightweight (37 kilogram) robot, standing about a metre tall, can use its flexible gripper arm to pick up objects from the floor or from a shelf and put them back again, and open and close curtains for example.
The HSR can also be controlled remotely. Family or friends can operate the robot even when they are away from the location. When doing so, the remote operator’s face is shown on the display and their voice is heard in real time, so enabling the person being assisted to interact with family and friends.
In order to drive the ongoing development of the robot, Toyota has established the HSR Developers’ Community in conjunction with a number of research institutions. Its aim is to put the HSR through practical trials, assure its continuous improvement, and achieve its launch as rapidly as possible. The robot is also being made available to various partner organisations such as universities, and also care homes, in order to refine the software further.
Practical trial
And so it was that the HSR also found its way to Romy Carmago, who tested the robot in his home. The assistance robot was required to perform two quite simple tasks in helping Romy with his day-to-day routine: open the house door as soon as he approached; and give him a drink when needed. They were two tasks that took a lot of training for the little robot. The appraisal by Toyota’s research team at the end of the trial is anything but euphoric: they note that there is still a long way to go before the technology is fit for the real world. But the development work goes on. The researchers are confident that the HSR will one day possess the necessary capabilities to enable people like Romy Camargo to live a more independent life.
Mission to Mars
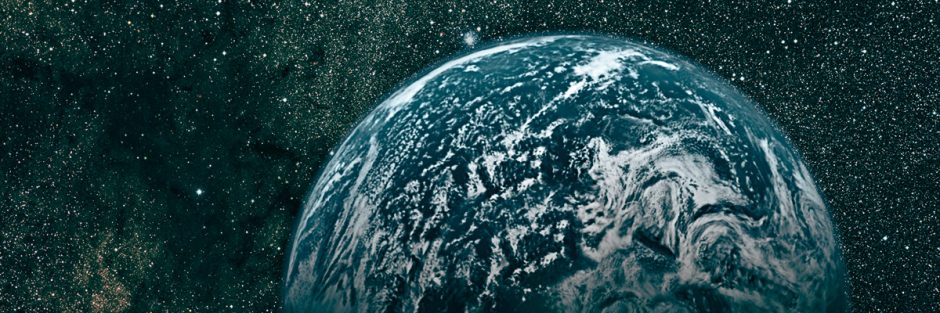
NASA is developing a humanoid robot which will be deployed ahead of any manned missions to Mars in the future. However, a little more research work is needed before “Valkyrie” will be able to operate autonomously on another planet.
A mission to Mars is one of NASA’s loftiest goals, yet robots are set to do the dangerous groundwork before humans set foot on the Red Planet. “Advances in robotics, including human-robotic collaboration, are critical to developing the capabilities required for our journey to Mars,” says Steve Jurczyk, Associate Administrator for the Space Technology Mission Directorate (STMD) at NASA Headquarters in Washington. With this aim in mind, the US space agency has been working for several years to develop the R5, a humanoid robot constructed by the Johnson Space Center. Its design featuring two legs, two arms and a head allows the robot to work alongside humans or perform high-risk tasks in their place.
Versatile Valkyrie
NASA named its model “Valkyrie” after the messenger to the gods in the Norse sagas. The robot is around 180 centimetres tall, weighs 136 kilograms and walks almost like a human does. 28 torque-monitored joints allow it to perform a wide range of movements. Each upper arm alone is equipped with four elastic rotary actuators arranged in series and has seven joints together with the lower arm. One further rotary actuator allows the robot to rotate its wrist, whilst linear actuators control the tilt and yaw angle. A simplified humanoid hand with three fingers and a thumb enables it to grip different objects. This means that the R5 can even turn a door handle. Three additional rotary actuators are accommodated in the robot’s pelvis to control movement in its waist and hip joints.
More than 200 sensors
The robot perceives its surroundings using a multi-modal sensor made by the firm Carnegie Robotics: the system collects distance data using a laser scanner and a stereo camera and supplements these with a video image of its surroundings. Further cameras are built into the torso. More than 200 sensors produce additional information; each hand alone is equipped with 38 sensors (six on the palm and eight along each of its four digits). Valkyrie processes this array of data using two Intel Core i7 COM Express processor cores.
Robots don’t always work
A little more development work is still needed before this robot can actually be sent to Mars, however. NASA has therefore tasked three universities – among others – with improving certain functions. As a consequence, work is being carried out on the autonomous functions, environmental perception and movement optimisation at MIT, the University of Edinburgh and Northeastern University in Boston. Sarah Hensley, an MIT student who is working on the elbow control system, understands only too well why this work is necessary. When Valkyrie is turned on and moves, Hensley says, it often “kind of shivers and falls down. Sometimes robots work, and sometimes they don’t. That’s our challenge.”
A million dollars for the winner
NASA therefore wants to hold a competition in order to provide additional incentives for improving Valkyrie. A million dollars are offered to anyone who succeeds in demonstrating that a virtual model of the R5 can repair the damage done to a Mars habitat by a sandstorm in a digital environment. To be specific, it would need to align a satellite dish, repair a solar energy system and patch up a leak in the habitat. The winners will be announced at the end of June 2017. The software developed in this competition should be transferable to other robot systems. In this way, the new technology is intended to benefit older robots and future systems alike. “Precise and dexterous robotics which are able to work with a communications delay could be used in space flight and ground missions to Mars and elsewhere for hazardous and complicated tasks, which will be crucial to support our astronauts,” says Monsi Roman, Program Manager of NASA’s Centennial Challenges.
Out of the box
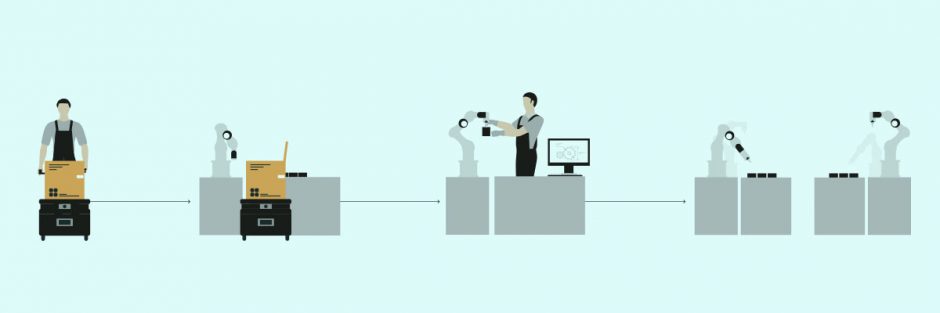
A new sensitive and teachable robot available to everyone is intended to democratise robotics. The system can be set up and programmed in just a few minutes.
A cheap, sensitive robot that can be deployed as a universally accessible multifunction tool – that is the vision of start-up company Franka Emika. With this goal in mind, the Munich-based company will release the first “out-of-the-box” robot onto the market in 2017: Franka Emika – the robot bears the same name as the company – can be set up and programmed in no time at all. Including a control unit and cloud software for management and programming, Franka Emika will cost less than 10,000 euros to buy. This will make the model particularly appealing to smaller companies. However, the price and hardware aren’t its only unusual features.
Working with sensitivity
The robot’s arm – with its seven axes and torque-based design – can immediately detect even the lightest touch and switches off when a human colleague comes too close, for example. This is crucial in scenarios where man and machine have to work in close quarters with one another. The modular, ultra-light construction, highly integrated mechatronic design and the ability to handle objects skilfully and sensitively enable the robot to fulfil tasks which necessitate direct physical contact with its surroundings. In turn, this means that frequently occurring but predominantly monotonous actions such as delicate insertion, twisting, joining or testing, inspection and installation tasks are able to be automated for the first time ever.
Programming via drag & drop
Instead of programming algorithms directly into Franka, workflows can be compiled in a visual user interface in the space of a few short minutes. To do so, users are able to select from pre-programmed motion sequences such as pressing a button or gripping an object. Franka is already pre-programmed with a selection of these so-called “skills”, while others can be purchased as add-ons in an online store. The store is also envisaged to offer apps soon. In other words: complete, pre-configured workflows.
The democratisation of robotics
For the start-up, the robot is an opportunity to completely re-think automation. The company’s founder, Sami Haddadin, views this as a democratisation of robotics: the key technology is not only powerful, but also affordable for anyone, flexible in operation and globally available.
Hands off the wheel
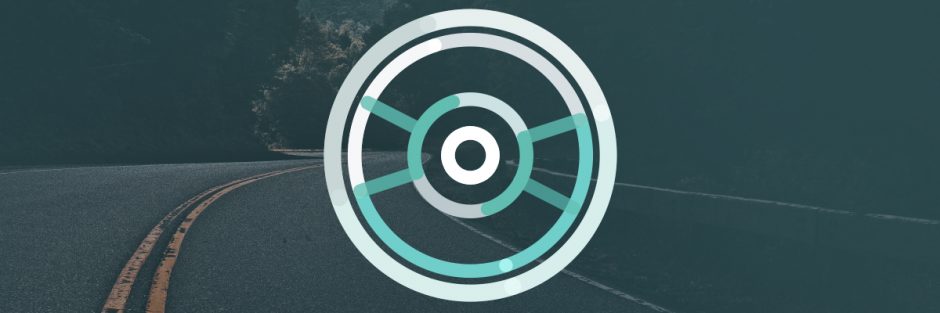
Amsterdam is home to the world’s first self-driving city bus to operate in real traffic. But there is still a driver on-board.
Somewhere between Amsterdam’s Schiphol airport and the town of Haarlem, on the longest express bus route in Europe: a bus stands at a traffic light, waiting for the signal to move off. At this light, two horizontally adjacent red dots mean stop; two vertically arranged white dots mean go. The light switches to white, the bus smoothly sets off, and pulls into its lane. Red light ahead – the bus brakes safely and gently, and comes to a stop. In all these manoeuvres the bus is controlled not by a human driver, but by electronics. It is, in fact, the world’s first self-driving city bus to operate in real traffic.
Autonomous driving
There is still a driver on-board, but he is somewhat underemployed as the bus continues on its journey, even as it negotiates two bridges and an underpass. The bus keeps safely in lane. As it reaches the outskirts, it accelerates up to the 70 km/h limit. The maximum speed is pre-programmed, and even at this speed the driver is doing nothing. The bus comes to a halt at the stop, the doors open and close, the bus sets off again – all automatically. At the next traffic light, the bus’s high-tech camera system detects the position of the signal. The bus also communicates with the road infrastructure and receives information on the status of traffic lights via its on-board wireless network. This enables it to run through all the lights as they automatically switch to green for it. As the light changes, there are still pedestrians crossing the street. The bus waits until they have crossed and the road ahead is clear before setting off. The bus has an automatic braking system which activates as and when necessary to avoid collisions.
Developed specially for cities
Daimler has been trialling this so-called Future Bus in real traffic on a BRT (Bus Rapid Transit) route around Amsterdam since 2016. Daimler has been working for a number of years to advance self-driving technology to production maturity. The new Mercedes-Benz E-Class, for example, was the world’s first mass-produced car to be granted a test licence for autonomous driving, in the US state of Nevada. And the Highway Pilot developed by the Daimler Trucks division is currently being trialled as a partially automated driving system for trucks. Dr Wolfgang Bernhard, Member of the Management Board of Daimler AG and Head of Daimler Trucks & Buses, comments: “Launched almost two years ago, our Highway Pilot demonstrates that autonomous driving will make long-distance truck transportation more efficient and safer. We are now bringing the technology to our city buses as the City Pilot. The system has been specially adapted to operate in cities. It runs in partially autonomous mode in specially assigned bus lanes.” BRT networks such as in Amsterdam are ideal candidates as a first step towards fully automated city bus operation: an unchanging route, running in a separated lane; a clearly defined timetable; unambiguous and identical actions at stops.
Cameras and sensors intelligently interlinked
The self-driving bus detects whether the route ahead is suitable for automated running, and signals the fact to the driver. A press of a button by the bus driver activates the City Pilot. For the system to operate, the driver must lift his foot off the accelerator or brake pedal and not steer, because any manual action would override the system. So the driver always retains control, and can intervene as necessary. The autonomous driving solution incorporates the latest assistance systems, as are used on-board Mercedes-Benz coaches for example, as well as additional systems which have been partially adopted by Daimler Trucks and enhanced to handle urban traffic. They include long and short range radar, a multiplicity of cameras, and the GPS satellite navigation system. Cameras and sensors are intelligently interlinked to create a detailed picture of the surroundings and identify the bus’s exact position.
Legal framework still lacking
The partially autonomous city bus aims to significantly enhance safety in urban traffic. Experts predict that autonomous driving will cut the number of accidents by 80 per cent by 2035. The predictive capabilities of the Future Bus also improve efficiency, place less strain on components, and reduce both fuel consumption and emissions. And the smooth, uniform ride additionally enhances passenger comfort. The law does not yet allow regular autonomous driving on the road however. “We urgently need to adapt the rules of the 20th century to the 21st,” Dr Bernhard warns. “But we should not get bogged down in bureaucracy. Before we start debating all the potential issues linked to fully autonomous driving, we first need to make partially autonomous driving possible. We need to allow drivers to take their hands off the wheel.”
AI – the Learning Robot
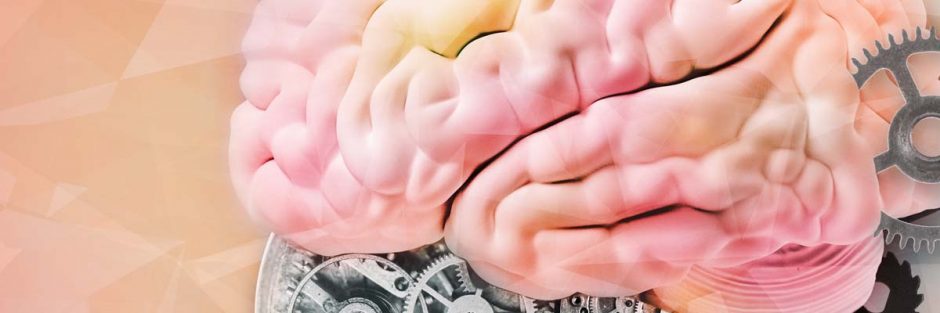
Artificial intelligence enables robots to perform tasks autonomously and find their way around unfamiliar environments. Ever more powerful algorithms and ultrahigh-performance microprocessors are allowing machines to learn faster and faster.
The term artificial intelligence (AI) has been in existence for over 60 years. During that time, research has been conducted into systems and methodologies capable of simulating the mechanisms of intelligent human behaviour. It might sound quite simple, but it has to date posed major challenges to scientists. Because many tasks that most people would not even associate with “intelligence” have in the past caused computers serious problems: understanding human speech; the ability to identify objects in pictures; or manoeuvring a robotic vehicle around unfamiliar terrain. Recently, however, artificial intelligence has been making giant strides, and is increasingly becoming a driver of economic growth. All major technology companies – all the key players in Silicon Valley – have AI departments. “Advances in artificial intelligence will allow robots to watch, learn and improve their capabilities,” said Kiyonori Inaba, Board Member, Executive Managing Officer and General Manager of Fanuc.
Simulating the human brain
Findings from brain research, in particular, have driven advances in artificial intelligence. Software algorithms and micro-electronics combine to create neuronal networks, just like the human brain. Depending on what information it captures, and how it evaluates it, a quite specific “information architecture” is created: the “memory”. The neuronal network is subject to continuous change as it is expanded or remodelled by new information. The technological foundations for state-of-the-art neuronal networks were laid back in the 1980s, but it is only now that powerful enough computers exist to permit the simulation of networks with many “hidden layers”.
Becoming ever better by learning
“Deep Learning” is the modern-day term used to describe this information architecture. The concept involves software systems which are capable of reprogramming themselves based on experimentation, with the behaviours that most reliably lead to a desired result ultimately emerging as the “winners”. Many well-known applications, such as the Siri and Cortana voice recognition systems, are essentially based on Deep Learning software. “Deep Learning will greatly reduce the time-consuming programming of robot behaviour,” asserts Kiyonori Inaba. His company Fanuc has integrated AI into its “Intelligent Edge Link and Drive” platform for fog computing (also referred to as edge computing). The integrated AI enables connected robots to “teach” each other, so as to perform their tasks more quickly and efficiently: whereas one robot would otherwise take eight hours to acquire the necessary “knowledge”, eight robots take just one hour.
New algorithms for faster learning success
Ever-improving algorithms are continually enhancing the ability of machines to learn. As one example, the Mitsubishi Electric Corporation recently launched a quick-training algorithm for Deep Learning, incorporating so-called inference functions which are required in order to identify, recognise and predict unknown facts based on known facts. The new algorithm is designed to aid the implementation of Deep Learning in vehicles, industrial robots and other machines by dramatically reducing the memory usage and computing time taken up by training. The algorithm shortens training times and cuts computing costs and memory requirements to around a thirtieth of those for conventional AI systems.
Special chips for Deep Learning
To obtain the extremely high computing power required in order to create a Deep Learning system, current solutions mostly involve so-called GPU computing. In this, the computing power of a graphics processor unit (GPU) and the CPU are combined. CPUs are specially designed for serial processing. By contrast, GPUs have thousands of smaller, more efficient processor units for parallel data processing. Consequently, GPU computing enables serial code segments to run on the CPU while parallel segments – such as the training of deep neuronal networks – are processed on the GPU. The results are dramatic improvements in performance. But the development of Deep Learning processors is by no means at an end: the “Eyriss” processor developed at the Massachusetts Institute of Technology (MIT), for example, surpasses the performance capability of GPUs by a factor of ten. Whereas large numbers of cores in a GPU share a single large memory bank, Eyriss features a dedicated memory for each core. Each core is capable of communicating with its immediate neighbours. This means data does not always have to be routed through the main memory, so the system works much faster. Vivienne Sze, one of the researchers on the Eyriss project, comments: “Deep Learning is useful for many applications, such as object recognition, speech or facial recognition.”
Robots in the fog: Fog-computing
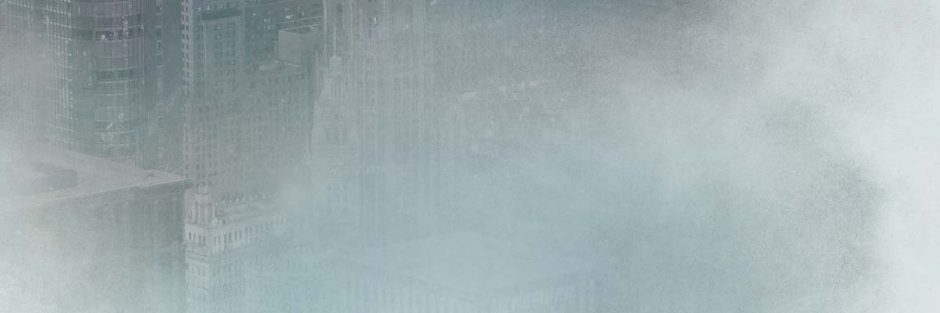
Even autonomous robots have to be integrated into a wider control system – whether in production or in the service sector. That demands fast, reliable communications standards, and the resources of the cloud. The latest keyword being used in this context is “fog computing”, which brings resources closer to the robot.
For robots to move autonomously around an environment and perform tasks, they have to record and compute vast amounts of data. It doesn’t necessarily have to happen “onboard” though. For small robots, especially, the resources needed would be too costly. One alternative is to monitor the robots by external systems, and also to transmit control commands to them from an external source, such as by wireless radio communication.
The autonomous bionic eMotionButterflies developed by Festo, for example, are coordinated three-dimensionally by an external real-time communicating control and monitoring system. The communication and sensor technology creates an indoor GPS system which collectively controls the butterflies, guiding them so as to avoid collisions. Ten cameras installed inside the room monitor the spheres by way of their active infrared markers (LEDs) and relay the position data to a central master computer. The computed action commands are transmitted back to the objects, where they are executed remotely.
Communication in real time
In industrial applications especially, robots are not “island solutions”, but have to interact with the other production systems. That demands communication solutions which transmit the signals not just in real time, but synchronised too. Only in that way can a mobile robot and a fixed machine work together on a component for example. Previously, most real time-capable control applications were realised by non-standardised network infrastructures or through separate networks, which made it much more difficult – and sometimes impossible – to access machinery and data. So a task group of the Institute of Electrical and Electronics Engineers (IEEE) is currently working to standardise real-time functionality in Ethernet. The result is Ethernet TSN (Time Sensitive Networking), a technology which many companies see as the future of communications in the age of Industry 4.0 (Smart Manufacturing). TSN enables a conventional, open network infrastructure to be implemented with cross-manufacturer interoperability, and offers guaranteed feed and performance. The technology supports control and synchronisation in real time – such as between motor control applications and robots – over a single Ethernet network. TSN is also suitable for other commonly used production data transfer methods, however, meaning IT and Engineering are able to share a network. So the biggest advantage of TSN is that it converges and more efficiently interconnects technologies in order to provide the critical data required for Big Data analyses.
Fog instead of Cloud
This means all data relevant to the robot functionality can be combined in one digital model in real time. And it happens in the Cloud, implementing the new era of Industry 4.0. However, the increasing numbers of cloud computing services and the large numbers of machines with access to cloud resources are heightening concerns that the network will become overloaded, resulting in bottlenecks and delays in data processing. A technology solution known as fog computing is intended to provide a remedy. It creates an intermediate level of data processing. This means the data is not – as it was previously – uploaded to the Cloud or to a remote data centre without being processed at all, but rather undergoes processing in server systems, storage and networking components close to the IT infrastructure. These so-called edge devices provide services and perform tasks that previously came from the Cloud, and so reduce the volumes of data being transferred. So whereas the Cloud is a rather nebulous, remote place, the “fog” is down close to ground level, where the work is actually being done. “Fog computing brings analytical, processing and storage functions right to the edge of the network,” explains Kay Wintrich, Technical Director of Cisco Germany. “In the Internet of Everything – in a completely connected world – that is the only way of handling the huge volumes of data.”
Flying robot in micro-format
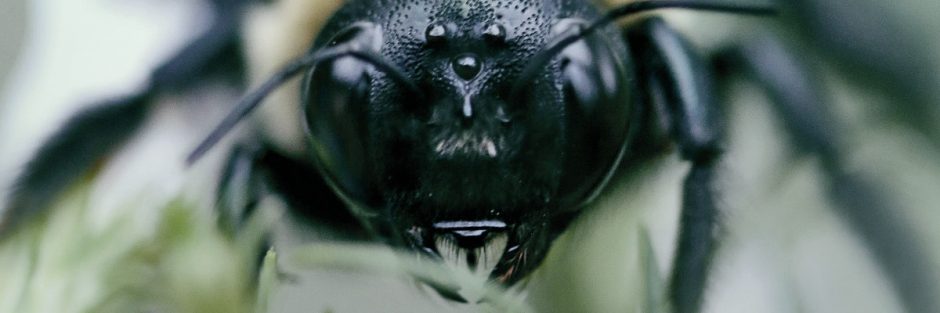
At Harvard University, researchers from a wide range of fields are working on a minuscule flying robot. The size of a bee, it is intended to explore inaccessible areas in a swarm or act as an artificial pollinator in the future.
More and more robots are being released onto the market whose form and function confound our preconceptions of machines. One of these rather unusual robotic applications is being developed at Harvard University: the “RoboBee”. In their project, the scientists aim to create an autonomous robotic insect which can fly continuously and independently. One day, such robots are intended to conduct fact-finding missions, help with telecommunications or even operate as artificial pollinators.
Controlled flight
The researchers from Harvard have been working together with colleagues from Northeastern University on the project for several years. As early as 2012, the scientists were able to astound the public by demonstrating the first controlled flight taken by an insect-sized robot. Since then, they have developed ever smaller and more demanding robots. One day, these should be able to fly entirely autonomously.
In order to achieve this goal, the scientists needed to push forward with preparatory research in many different fields: these include micro-manufacturing methods and materials for microscopically small propulsion systems, energy storage devices on the smallest possible scale and even algorithms needed to effectively control individual RoboBees or entire swarms of them.
Highlights include new methods for manufacturing devices that are just millimetres in size, for which layering and folding technology were implemented. New sensors have also been developed which can be used in the low-power range or in portable computers. Special architectures for ultra-low-power computer systems are another result of the development efforts. Finally, the scientists wrote algorithms for coordinating hundreds or even thousands of robots, thus enabling them to operate together effectively.
Taking inspiration from nature
The project team also took a cue from nature – particularly from the capabilities that let insects take off, navigate and perform agile manoeuvres without external help and in spite of their tiny bodies.
“Bees and other social insects provide a fascinating model for engineered systems that can manoeuvre in unstructured environments, sense their surroundings, communicate and perform complex tasks as a collective full of relatively simple individuals,” says Robert Wood, who heads the project. “The RoboBees project grew out of this inspiration and has developed solutions to numerous fundamental challenges – challenges that are motivated by the small scale of the individual and large scale of the collective.” The current RoboBees weigh a mere 84 milligrams – making them even lighter than real bees at roughly the same size. Currently, the team is working on enabling the mini-robots to detect their surroundings with a laser.
Stopovers save energy
What’s more, the RoboBees can now even land on a branch or similar object in order to save energy – just as bats, birds or butterflies do. “The use of adhesives that are controllable without complex physical mechanisms, are low-power and can adhere to a large array of surfaces is perfect for robots that are agile yet have a limited payload – like the RoboBee,” Wood adds. “When making robots the size of insects, simplicity and low power are always key constraints.” Wood’s team used an electrode patch which exploits electrostatic adhesion, allowing the RoboBee to stick to almost any surface – whether it be glass, wood or the leaf on a tree. For a brief intermediate landing, the patch only requires around a thousandth of the energy needed to take off.
Out in the real world in five to ten years
“Aerial micro-robots have enormous potential for large-scale sensor deployment to inaccessible, expansive and dangerous locations. However, flight is energy-intensive, and the limitations of current energy storage technologies severely curtail in-air operations,” says Jordan Berg, a Programme Director at the NSF (National Science Foundation) who is familiar with the project. The NSF has been co-financing the project for some years. However, the project team won’t be deterred; their work to advance the RoboBee is incessant. It is envisaged that the landing mechanism will become independent of direction, allowing the mini-robot to land anywhere. Work is also being conducted on onboard energy sources for the robot. These would enable the robot to generate its own energy and fly without the wire that has been required until now. Wood estimates that it will take another five to ten years before the RoboBee can actually be let loose in the real world.
(Picture Credits: Unsplash: Kelsey Krajewski)
Security robot on patrol
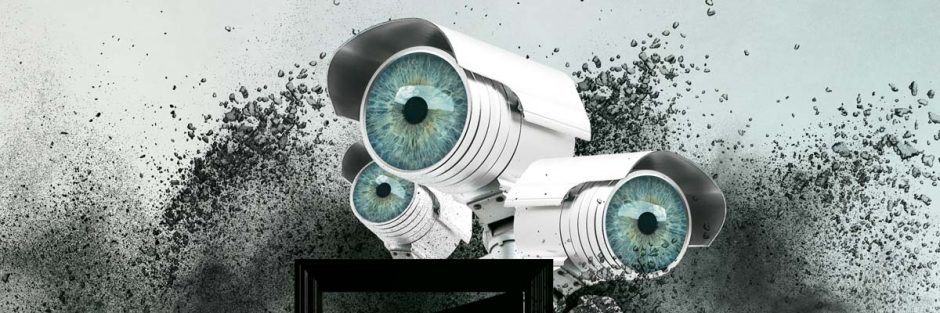
The security robot SAM3 was designed to be a security guard’s best friend. Using its sensor systems, it can detect intruders, defects in electrical equipment or dangerous gases. It knows no weakness in operation and can provide effective, 24/7 support to its human colleagues.
The small, box-shaped robot completes lap after lap of the car park and keeps watch over the cars parked there. It never gets tired; its attention levels never drop. Although it may look innocuous, the five antennae on its “roof” give an idea of just how much technology its façade conceals: a 360-degree camera, a thermal camera, a laser scanner, various sensors and an ID reader – among other things – are all contained in the diminutive, half-metre-tall housing of the SAM3. Using these systems, the highly advanced smart security guard can detect objects and people while on the move. The SAM3 knows the floor plan of the guarded building inside out, can easily dodge obstacles on its patrol route, take the lift and even open automatic doors.
A helpful colleague
The robot was developed by the firm Robot Security Systems, based in The Hague. The firm has been performing operational tests of the SAM3 as a mobile car park guard since August 2016 in cooperation with facility management company Sodexo. “Technology can make our work easier, safer and more efficient,” says Sepp Rickli, Sodexo General Services Manager and supervisor of the joint project. “The robot will not replace security personnel, but it will be a helpful colleague.”
The founders of Robot Security Systems have themselves worked for many years in the security industry and came up against one particular weakness time and time again: the human element. After all, human security personnel can get distracted or tired and their attention levels can drop on routine patrols – and people can be bribed. SAM3 is now heralded as the solution. The robot was conceived especially for security operations. Its sensors and cameras make it the eyes and ears of the human security guards, meaning it can be easily integrated into existing security systems. It will immediately raise the alarm in the control centre if it detects an intruder, for example. It then falls to a human to decide what action to take.
SAM3 can be more than a security guard
“The robot is pre-programmed with a variety of features such as a site plan, the locations of detectors and a catalogue of measures for the security personnel,” explains Edwin Lustig, CEO of Robot Security Systems. SAM3 can be additionally equipped with various extra sensors to help it detect heat or fire, radiation, faults in electrical systems, CO2 or other poisonous gases.
Sodexo manager Rickli can easily imagine the metallic car park guard performing other tasks as well: “The robot can also contribute to hospitality, for instance by taking people to their destination, identifying free parking slots, or by contacting a human colleague.”
Human & Robot: From a tough guy to a softie
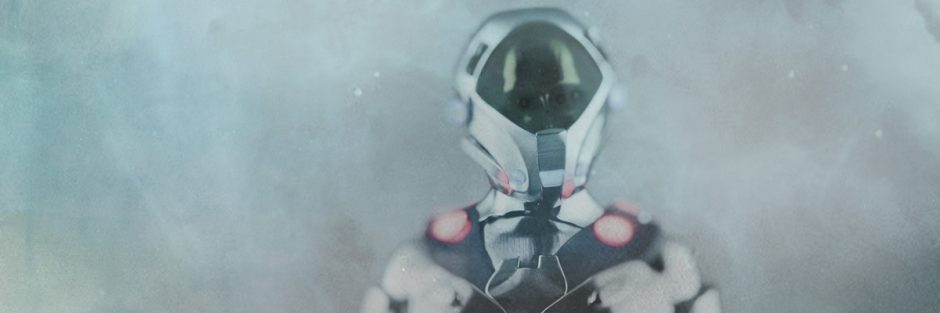
Robots are going to be interacting ever more closely with humans in future. For that to happen, it is essential that robots are able to perceive people, react to their actions, and do not endanger them in any way.
One of the key trends in robotics is direct collaboration between humans and machines. Whereas just a few years ago robots were mainly to be found in industrial applications, they are nowadays also helping people in areas such as disaster relief, in the home, and in surgical operations. In doing so, they are increasingly moving out of their former protected, encapsulated work spaces and interacting directly with humans. That demands very high standards of safety and functional reliability. There are already numerous standards ensuring that industrial robots do not pose a danger to humans or to their environment even in the event of a malfunction. EN ISO 10218, for example, sets out the requirements for collaborative robots, and can also be applied outside the industrial sphere.
All of this is based on the huge recent advances in electronics and software development. Because in order to avoid harming humans, such service robots have to possess special abilities. Equipped with complex sensor capability, they are able to reliably sense the world around them and their human partners. In future, cognitive skills will additionally enable them to predict and interpret human actions in order to derive their own helpful, safe responses.
Sensing their working area
Interactive robots monitor their environment, and their allotted working areas, by means of multiple different sensor systems such as cameras and ultrasonic and pressure-sensitive sensors. Using such multi-sensor systems, robots are able to sense their environments with precision and detect any motion within the space. Merging data from different sensors means dynamic obstacles can be tracked and their position and speed estimated. As a result, robots are able to compute how an obstacle – such as a person – will move, and whether a collision might ensue. As the robot moves, its distance from the obstacle is continuously monitored. If the robot detects an unexpected obstacle, it slows its movement or adjusts its planned path so as to avoid a collision.
Commands by gestures
Genuine interaction demands efficient communication between humans and machines. Thanks to great technical advances, new possibilities are available today which extend well beyond keypad input or swiping. Robots that work with humans need a voice – and in particular intelligent understanding of the spoken word – in order to be truly useful assistants in everyday life. Reliable voice recognition in as many languages as possible, complex semantic processing, incorporating context (whether that be time and place, or information from apps and databases), and natural sounding speech are key requirements. According to the Consumer Technology Association (CTA), the accuracy of word recognition has today reached 95 per cent, thanks in part to developments such as IBM’s Watson, Google Home, Apple’s Siri and Amazon’s Alexa. Back in 1990, recognition rates were virtually zero, and even by 2013 had only reached around 75 per cent. Based on such developments, it is likely that in 2017 computers will for the first time understand the spoken word as well as humans do.
People take in about 80 per cent of all information visually, so it makes sense to communicate with robots visually too. New 3D sensor technologies allied to fast data processing and interpretation methods are enabling machines to sense and understand gestures and commands. For example, in future a person will merely have to point to an object and a robot will bring it.
Copying nature
Some quite new impulses are being delivered by a very new but highly promising field of research: soft robotics. It uses biologically inspired technologies to create soft, organic structures, copying movements observed in nature. Robots built according to this principle will no longer be made of rigid materials, executing unstoppable movements and so posing a major hazard to humans. The research involves a wide range of different scientific disciplines, with the fields of electronics, materials, software development, sensor technology and drive engineering all being interlinked. The aim is to create intuitive, safe, sensitive interaction between humans and robots.
No hard parts
Researchers at Harvard University have developed a small, 3D-printed soft robot which requires no electronics. The Octobot – named in keeping with its octopus-like shape – needs no batteries, but instead draws its power from a fuel. It is driven by a chemical reaction which is controlled by microfluids. Such entirely soft robots have no hard edges or solid bodies that might pose a hazard to people. Soft robots are also opening up entirely new potential applications, as their softness allowed them to squeeze through narrow gaps where conventional robots would falter. This is providing particularly exciting potential for robots assisting in disaster relief, for example.
Robots in Automotive Industry: A real help
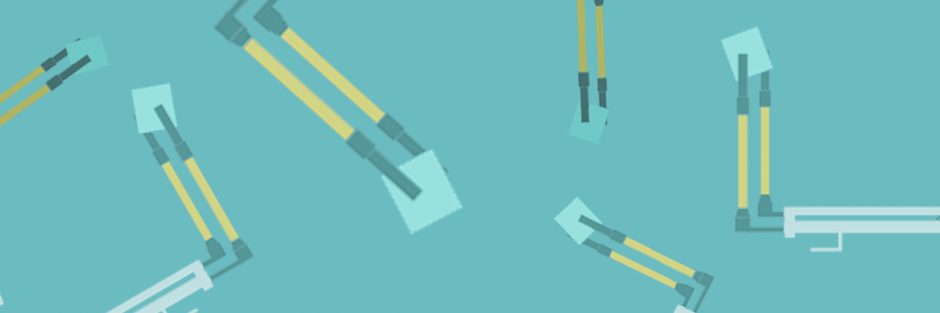
Ford has integrated collaborative robots into the Fiesta assembly line at its Cologne plant. They are working hand-in-hand with the human personnel, and assisting them by carrying out the difficult overhead installation of shock absorbers.
Collaborative lightweight robots from Kuka have been operating for some time at the Ford plant in Cologne. The LBR Iiwa robots help install high-performance shock absorbers in the Ford Fiesta – a task that proved very difficult using conventional automation solutions. Previously, the staff on the line had to carry out ergonomically challenging, high-tech work in a fast-moving environment by purely manual means.
“The Fiesta production facility in Cologne is the first Ford plant in the world to employ this innovative technology,” reports Karl Anton, Director Vehicle Operations of Ford Europe. Previously, the staff on the shock absorber assembly line had to perform manual tasks overhead. “The overhead working was not the only difficulty. The staff had to hold a pneumatic bolting tool and the shock absorber in their hand simultaneously, additionally supporting the weight of both. The new system has eliminated the overhead working and the weight-bearing, so it is a major ergonomic enhancement for the staff,” explains René Zimmermann, Manufacturing Engineering Manager on Ford’s final assembly line in Cologne.
Sensitive robotic arm
The collaborative lightweight robot features state-of-the-art sensor technology, and has no edges. The articulation movement sensors on the LBR Iiwa immediately detect any contact, causing it to reduce the force and velocity of its motion. That means – in contrast to previous automated systems – it needs neither guards nor additional safety coverings.
Today, all the employee at the workstation has to do is position the bolts and the shock absorber on the system – effectively placing it in the robot’s “hand”. A light touch gives the robot the signal to start working. If working with a robot without collaborative capabilities, the employee would first have had to move out of the safety zone around the robot and activate the start signal from a control panel, which would be much more time-consuming. By contrast, the robotic arm of the LBR Iiwa reacts directly, first retracting a short way, and then checking by a built-in camera that the shock absorber is correctly positioned. The employee can thus stay within the operating space of the arm the whole time. If everything is in the right place, the robot automatically moves towards the wheel housing. There the employee checks the position again and gives the signal to start bolting.
At this point at the latest, it becomes clear that a conventional industrial robot cannot be used for the task, because the employee has to position the button directly adjacent to the robot’s arm in order to check the position. With a non-collaborative robot, the risk that the employee might be injured by a movement of the robot would be much too great, meaning the safety systems would bring the robot to a complete stop. So the task could no longer be performed on the running production line.
“The LBR Iiwa is sensitive, compliant, safe, precise and flexible, and is equipped with mechanical systems and drive technology for industrial operation.”
Jakob Berghofer, Product Manager LBR Iiwa & Sunrise.OS, KUKA Roboter
Physical stress reduced
The new collaborative robots operate in both production systems at the Cologne automotive plant. “The Ford final assembly line currently has four of the lightweight robots working on shock absorber assembly. The feedback from the staff at the workstations so far has been highly positive. Their physical stress has been greatly reduced as a result,” Zimmermann reports. “On these lines we have successfully integrated the lightweight robots into our existing vehicle production. At present we are investigating additional possibilities for the use of collaborative systems. The key to any decision, though, is that the ergonomics and workflows should be improved as well as enhancing efficiency,” Anton concludes.
Seeing more: New camera technology for 3D vision
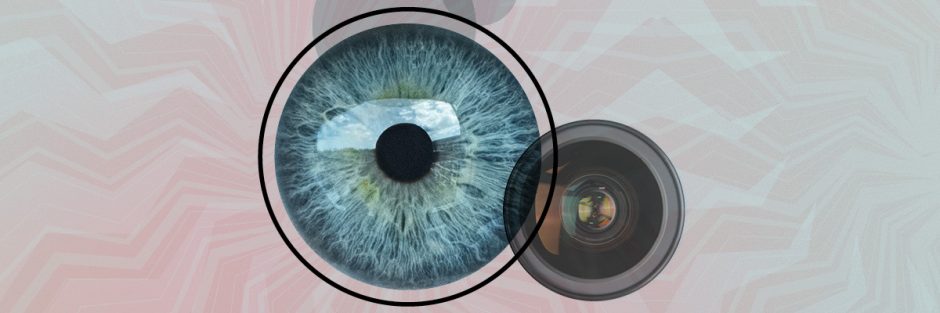
Sight is a key factor in enabling robots to assist humans in their everyday lives. Equipped with various sensor systems, they not only detect objects, but also precisely measure their alignment and position. The technology is by now so advanced that robots are even able to view an object down to its molecular level – and so see much more than their human model, the eye.
In order to assist people as flexibly and autonomously as possible, a robot must be able to recognise objects and detect their exact position. Only in that way can it conceivably execute commands such as “bring the glass”, or “hold the component”. To achieve those goals, robot manufacturers are employing the full range of sensors that are familiar from the industrial image processing field – from ultrasonic sensors to laser scanners.
The main systems in use, however, are various types of cameras. Even “simple” 2D solutions enable contours to be traced and objects identified. They come up against their limits, however, when items are stacked in an undefined way. And with just “one eye” it is impossible to gather height data – yet that is important for a robot in order to pick up an object and to judge the position of its own gripper relative to the object.
Seeing in three dimensions
That is why – as robots become increasingly flexible and mobile – systems providing a three-dimensional view are gaining in significance. One method frequently employed nowadays to provide robots with such 3D vision is based on nature: Like a human being, the machine has two eyes in the form of two offset cameras. Both cameras capture the same image, but owing to the offset their perspectives are different. An electronic evaluation unit in the stereo camera calculates the distance to the viewed object by way of this parallax shift. At present, camera systems use either CCD or CMOS sensors to capture the light signals. The trend is shifting clearly towards CMOS sensor technology, however. It is virtually glare-free, features high temperature resistance and low power consumption, while offering comparable image quality, and is also cheaper to produce.
Measuring and imaging in one
Three-dimensional imaging by stereo camera is complex and costly, however. So ToF (Time-of-Flight) technology is increasingly being used to provide robots with 3D vision. For this, a sensor chip both captures the image of an object and at the same time measures how far away it is. The core feature of ToF technology is that it measures the time the light takes to travel from the source to the object and back to the camera. The sensors used thus deliver two data types per pixel: an intensity value (grey scale) and a distance value (depth of field). This results in a pixel cloud comprising several thousand pixels, depending on the chip, from which the associated software can very precisely compute the nature and distance of an object. The cameras have their own active exposure unit which emits laser or infrared light. This means ToF systems are independent of the ambient light. As opposed to 3D stereo imaging, the distance is not calculated. Instead, an exact measurement is performed pixel by pixel. This means that ToF cameras work at very high speed. The resolution of the resultant image is lower than from stereo cameras, however. Consequently, ToF systems are frequently coupled with stereo systems in order to utilise the benefits of both, and produce an optimally dense and exact depth map. State-of-the-art camera systems also enable motion vectors to be captured. To do this, the position of a structure in two consecutively captured images is compared by software in order to obtain movement data.
Faster with micro-mirrors
Another method utilises the attributes of MEMS technology: DLP (Digital Light Processing) systems consist of a chip with several million microscopically small mirrors. Each micro-mirror is less than one fifth the width of a human hair. Each mirror can be activated individually, switching several thousand times per second. This means a precisely structured light pattern can be reflected onto an object from a light source. By projecting a series of such light patterns onto an object, and recording the distortion of the light by the object using sensors or cameras, a very detailed 3D pixel cloud can be created. Thanks to their high switching speed, the large number of grey scales and the ability to capture light in the visible range as well as in the UV and infrared range, 3D solutions for optical measurement using DLP technology are faster and more precise than the conventional solutions.
Viewing chemical properties
3D systems featuring hyperspectral image processing are also relatively new innovations. They use more than 100 different wavelengths to analyse an object. Broken down into their spectra, they are reflected differently by each material with its specific chemical and molecular properties. So every object has a specific spectral signature; a unique fingerprint based on which it can be identified. This enables really profound insights down to the molecular level of an object. In achieving this, robots have surpassed their role model – because for humans X-ray vision is still science-fiction.
The father of the Laws of Robotics
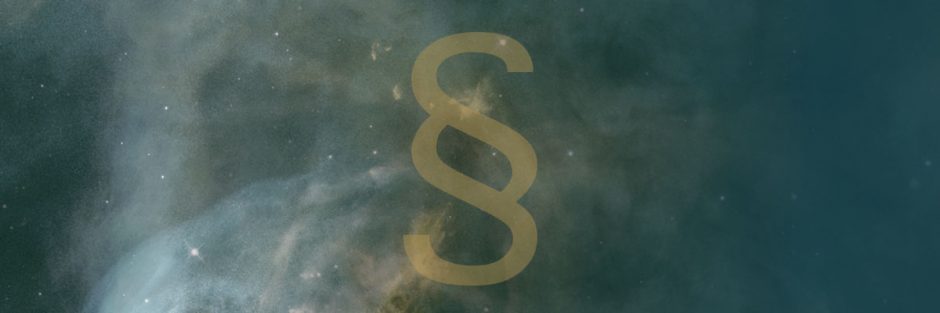
By publishing his three Laws of Robotics in 1942, Isaac Asimov defined rules for humans and robots to coexist which are more relevant today than ever before. The author and scientist, who died in 1992, didn’t just inspire the science-fiction community with his stories, but robotics developers as well.
Speedy no longer knows what to do. The nimble robot has been sent to gather the urgently required raw material selenium on the planet Mercury, yet this entails huge risks to his very existence. On the other hand, he wants to obey orders given to him by humans. The conflict between the laws governing his behaviour is just too much to take, causing Speedy to go haywire. He recites Gilbert and Sullivan and drives around and around in circles. Only when his human handler risks his life in front of Speedy’s eyes can the robot’s electronic brain think clearly again: after all, Speedy must obey the First Law at all costs.
- A robot may not injure a human being or, through inaction, allow a human being to come to harm.
- A robot must obey orders given to it by human beings except where such orders would conflict with the First Law.
- A robot must protect its own existence as long as such protection does not conflict with the First or Second Laws.
Ethical questions are becoming relevant today
Isaac Asimov cemented his place in history with this scene from his short story “Runaround”, published in 1942. Over the years that followed, numerous authors and film-makers took inspiration from the Laws of Robotics laid down for the first time in the short story and used them as a basis for creating their own works of fiction. Asimov’s ideas are not purely the stuff of fiction, but attracted a great deal of interest from researchers from the fields of Robotics and Artificial Intelligence – and continue to do so. Even today, the Three Laws serve as a fundamental code of practice for developers when programming their robots. “With the impending arrival of the first autonomous robots in the midst of our society, certain ethical questions that science-fiction author Isaac Asimov first formulated as his famous Laws of Robotics in 1942 will become very relevant – for example, whether a robot may kill or injure humans,” says Philipp Schaumann from website Sicherheitskultur.at. He also gave a lecture on the topic of “Ethics for autonomous vehicles” at the IT-Security Community Xchange symposium in late 2016.
From biochemist to author
Yet it wasn’t just the relationship between humans and robots that occupied Asimov, who was born on 2 January 1920 in Petrovichi, Russia. Among his bibliography of over 500 books, there are numerous scientific works on physics, chemistry and other natural sciences, yet also books on the Bible, William Shakespeare or Greek and Roman history. Asimov was a scientist by vocation, after all. After emigrating to the USA with his family at the age of three and growing up in Brooklyn, he studied chemistry at Columbia University and gained his Doctorate in Biochemistry in 1949. The scientist first came into contact with the American science-fiction scene while it was still in its infancy during his studies. He published his first short story in 1939, yet only became a full-time author in 1958, giving up his position as a lecturer at Boston University in order to dedicate himself fully to his writing.
Spotlight on the conflict between technology and ethics
The “Foundation” trilogy was one of Asimov’s most successful works. In this science-fiction series played out on a galactic scale, Asimov tells how a scientist succeeds in predicting and guiding the development of humanity for millennia using so-called “psychohistorics”. Asimov combined this trilogy with his many robotics and Galactic Empire novels to form a comprehensive series about the emergence of a new civilisation in outer space.
The conflict between technology and ethics is one theme which is common to all of his novels and short stories. It wasn’t just Asimov’s preoccupation with futuristic technology that came to the fore in his stories about robots, but also the effects of this technology on human society – and the dilemmas that this might bring about.
EU Parliament demands legislation on robots
A press statement from the European Parliament released at the start of 2017 shows just how far ahead of his time Asimov really was. In it, delegates call on the EU Commission to submit legislation concerning robotics and artificial intelligence. “One option might be to assign robots the status of ‘electronic persons’, at least as far as compensation for damage is concerned,” reported Mady Delvaux in Luxembourg. It was she who formulated the report in question and submitted it to the Commission. The EU Parliament delegates propose that researchers and designers should have a voluntary ethical code of conduct for robotics in order to ensure that their actions comply with legal and ethical standards and that the design and use of robots respect human dignity.
Asimov foresaw this ambivalence between ethics and robotics: in his fictitious version of 2015, robots are indispensable helpers in the conquest of distant planets. On Earth, however, they are forbidden due to people’s fear of them.
(Picture Credis: United States Library of Congress; Unsplash: NASA)
Turning ideas into reality
As Europe’s largest semiconductor distributor, EBV Elektronik supplies all the electronic components necessary for the development of autonomous robots capable of interacting closely with people. But that is not all, as Bernard Vicens, EBV Director Segment Smart Consumer & Building, emphasises. If required, the company offers its customers a complete ecosystem of solutions, identifying new potential business fields in conjunction with them.
Do you have a robot at home already?
Bernard Vicens: Not yet. I’m thinking about getting a robotic lawnmower in the future.
What makes robotics so exciting for you as an electronics distributor?
B. V.: First of all, robotics remind me – and probably all of us – of science-fiction movies and novels I saw and read when I was young … which sometimes can be scary! In the scope of our business, we are still at the very early phase, but applications are across all market segments. Also requirements are huge in terms of computing power, power management, security, sensors, human-machine interfaces and communications, so soon this market will become strategic.
Which components does EBV offer for robots?
B. V.: We have solutions for all the technologies listed above. Moreover, our portfolio is expanding, particularly with new sensor technologies.
Where do you see the most exciting market for robots at present?
B. V.: I think the home assistant robot market is the next big thing, with voice recognition such as Amazon Echo or Google Home. We will soon see a similar approach with mobile robots addressing various home applications like child-care, entertainment, security, comfort …
Do you have any “favourite projects” that you have seen recently?
B. V.: Well, there are several interesting projects. For example the NemH2O. This swimming pool robot is able to stay in the water forever, thanks to high-power induction charging. Another favourite is Wiigo, an autonomous, self-driven shopping cart designed to follow people with or without reduced mobility in supermarkets. Then there is Keecker, a smart multimedia robot that moves throughout the home to bring entertainment, communication and security to each room. Another exciting project is Buddy, an open-source companion robot that connects, protects, and interacts with each member of a family.
The field of robotics is currently making giant strides in development. Which technologies or trends are largely “responsible” for this in your view?
B. V.: The improvement that voice recognition has recently made is greatly facilitating interaction between humans and robots. For example, it is possible to embed a voice trigger in your application – you just have to utter a “key word” to wake up a system. And the Cloud provides unlimited capacities to manage sophisticated voice recognition – there is virtually no limit to the performance of voice recognition.
Which aspects of robotics do you find particularly exciting at the moment?
B. V.: Human-machine interfaces are really improving. Again, voice recognition will definitely simplify our interaction with robots, but the fact that robots are interacting ever more closely with humans – for example as care robots – means that very strict safety processes must be implemented. Which reminds me of the three laws of Isaac Asimov …
A Tesla causes an accident because it cannot differentiate a jack-knifed lorry from a bridge. A security robot knocks over a child in a shopping centre. Are robotic systems really advanced enough that they can be used in day-to-day tasks?
B. V.: Obviously, your examples show that safety rules for robots still need improvement. However, if we use a larger-scale comparison, we might want to check how many accidents were avoided by an autonomous driving Tesla as opposed to human driving. Don’t forget that a robot is able to react in a thousandth of the time a human takes.
Many of the robots presented in the magazine have been developed by start-ups. Is the market for robot applications a start-up market?
B. V.: Maybe established industrial companies are under-represented in the magazine? But yes, innovation is, as usual, coming from start-ups. Nevertheless, I expect big consumer players such as Samsung and LG to bring similar products onto the market soon.
It is evident that there are many company founders among them who originated from the target market – for example, agriculture or security – but who had never previously had anything to do with electronics, sensors or artificial intelligence. How can EBV help them to successfully realise their robotic visions?
B. V.: There is a similar situation in the IoT market. Our main goal is to turn our customer’s ideas into reality. Of course, we are offering the right electronic component solutions, thanks to our best-in-class sales organisation that includes specialist engineers, but we also provide the complete ecosystem including partners that can offer hardware, software design support, manufacturing, and so on.
Can EBV also offer something to experienced robotics manufacturers beyond mere components?
B. V.: As I said earlier, we are offering a complete ecosystem. In fact, in some cases we can also create awareness for our customers and give them an idea of potential additional business.
There is a wide range of different technologies in the field of navigation and environmental recognition in particular. How can the right solution be identified among them?
B. V.: It really depends on the application context. First, we need to differentiate outdoor applications where a GPS signal can be used. Then some technologies, such as radar, offer longer-range detection. In some cases, infrared Time-of-Flight sensors are adequate. In other cases, motion detection and magnetometer MEMS can help to determine position and orientation. I strongly believe customers will often combine technologies to gain the best solution for their system. Especially be aware that autonomous cars will in the near future combine at least three different technologies to determine their position.
From your point of view, which regions or countries are currently leading the way in the development of new robots?
B. V.: The United States, Korea, France, Denmark, Germany and Italy are countries with many activities and companies. Moreover, in those countries universities and public institutions are also driving the market by investing in specific programmes and events.
Which markets or industries are particularly exciting in terms of robotics at the moment? How will this develop in the future?
B. V.: Home service robotics could really improve our day-to-day life. With multiple applications, such as taking care of children and elderly people, or entertaining the family. When we add appropriate sensors, robots could even check air quality and control comfort and – why not – switch off appliances to save energy if we forget.
Do you believe that we will all have a robot at home one day?
B. V.: Yes, definitely.
Robotics start-ups
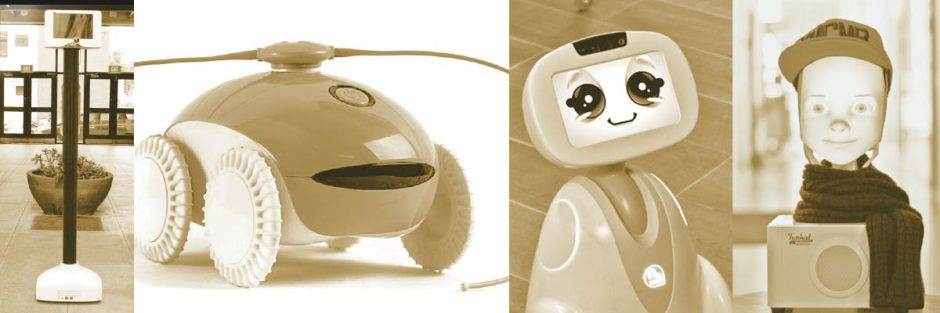
According to “The Robot Report”, 2016 was the best year for robotics start-ups: Almost 2 billion Dollars – around twice as much as in the previous year – was invested, and 128 new businesses were established. here We present some of the start-ups in the robotics sector.
Workmate robot
The robots named Baxter and Sawyer from Boston-based company Rethink Robotics, running on the Intera software platform, are able to adapt to the variable demands of the working day, quickly switching applications and performing tasks exactly like humans. As a result, manufacturers of all kinds and sizes, and in any sector, have at their disposal a rapidly deployable, user-friendly and highly versatile automation solution.
www.rethinkrobotics.com
Experience anywhere without having to travel
Australian company Aubot has developed a telepresence robot which enables people to take part in trips or meetings without having to actually go to the location in person. The mobile robot is controlled by thought (via the MindWave interface) and using a Web browser, enabling users to move around an office, meet other people or tour a city without actually leaving home.
www.aubot.com
Autonomous suitcase
US company Travelmate Robotics has devised a suitcase which follows its owner faithfully around. The case can move while upright or laid flat, and continually adjusts its speed to keep pace with its owner. Integrated sensors detect obstacles and record the distance travelled. A smartphone app and a GPS module inside the case indicate its location at all times.
www.travelmaterobotics.com
Starting early
The German company Kinematics develops and manufactures robotic kits named Tinkerbots with which children aged 6 and over can take their first steps into the world of technology. The Tinkerbots are able to learn, and can be brought to life by watching and copying movements, or can be controlled by an app on a smartphone or tablet.
www.tinkerbots.de
A social head
Machines with social intelligence – that is what Swedish company Furhat Robotics is looking to build. A pioneer in the field is the robotic head named Furhat. It features a 3D mask onto which eyes, a nose and mouth are projected from behind. This allows the head to mimic a person in a very authentic way. Furhat is controlled by an AI platform which enables it to engage in complex dialogue with humans.
www.furhatrobotics.com
Intelligent massage
The Californian company Dreambots has developed a robotic massager the size of the palm of a hand which utilises sensor technology to move autonomously around the body. Its special sensors ensure that it does not fall off. With its special wheels and gentle vibration, the WheeMe provides a relaxing massage.
www.dreambots.com
Material transporter for small businesses
The LeanAGV from Portuguese company Talus Robotics is specially designed for small and medium-sized enterprises. The driverless transport system’s core components are a drive module, a control system, and a set of safety sensors. From them, customers can construct a tailored system that is easy to use and flexible.
www.talusrobotics.eu
A real pal
Blue Frog Robotics presents Buddy, a social robot who provides a communication link, protects, and interacts with all the family. The 60 centimetre tall robot designed in France watches over the home, entertains the children, and maintains contacts with friends and family. The little robot was developed as an Open Source project, and is child’s play to operate.
www.bluefrogrobotics.com
(Picture Credis: Aubot; Blue Frog Robotics; Dreambots; Furhat Robotics; Kinematics; Rethink Robotics; Talus Robotics; Travelmate)
Parcel delivered by 6D9
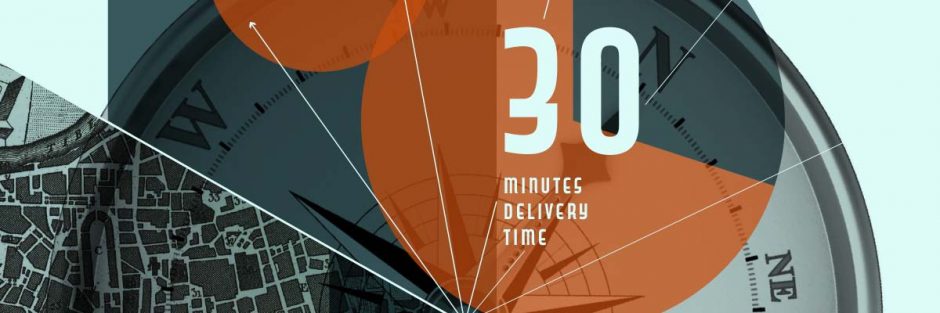
Delivery robots are the solution to steadily rising parcel volumes resulting from online retail. They will deliver parcels to customers’ doors quickly, safely and autonomously, and so relieve road congestion. Logistics service provider Hermes has been testing the robot 6D9 from Starship Technologies over a number of months in Hamburg.
The consequences of the trend towards ever more online shopping are seen in many towns and cities, especially in the run-up to Christmas. It often seems that there are parcel service vans standing by the roadside every few metres, usually double-parked too – an irritating obstruction that causes congestion on the roads and increases pollution. So experts are working feverishly, and with great creativity, to come up with new, sustainable solutions.
With a little luck, it might be possible to experience one such solution in operation around the Ottensen area of Hamburg. For a period up to March 2017, logistics service provider Hermes Germany has been testing delivery robots from start-up company Starship Technologies. “Using robots can revolutionise parcel delivery, particularly in urban areas,” asserts Frank Rausch, CEO of Hermes Germany.
Using the pavement
The delivery robot 6D9 is a six-wheeled vehicle, 50 centimetres tall and 70 centimetres long. It incorporates a secure compartment capable of carrying a payload with a total maximum weight of 15 kilograms. The delivery robot runs solely on the pavement, at a maximum speed of 6 km/h – that is to say, at walking pace. It only crosses cycle paths and roads after checking first that all is clear. The built-in cameras and sensors ensure that approaching obstacles are automatically detected, and the robot immediately stops. Bright LEDs mean every robot is clearly visible from a distance.
A new service channel
The robots can operate within a radius of up to five kilometres. This means automated deliveries can be made within 30 minutes of a customer placing an order. During the pilot, the delivery robots run back and forth between the participating parcel shops and selected test customers. The consignments carried are regular orders which customers have requested to be delivered to a Hermes parcel shop rather than to their home. Instead of going to the shop to collect their order in person, the test customers can use their smartphone to arrange for a robot to then bring the parcel to their home. So the robots do not replace conventional parcel delivery routes, or indeed parcel services. Rather, with the Starship robot Hermes is piloting a new service channel which eliminates the need for customers to come and collect their order in person from a shop.
Well protected against theft
The consignments in the transport compartment are protected against unauthorised access by a security lock, a surveillance camera and a PIN code. Once the robot has reached its destination, the recipient receives a text message notification and can go to the door to take delivery of the order. The transport compartment is opened using an encrypted personalised link. If any attempt is made to open the compartment by force, the robot immediately triggers an alarm and notifies the operator. The robot’s position can be tracked at all times by its continuous GPS signal.
Still with human assistance
6D9 navigates by a combination of locating signals (such as GPS) and visualising its surroundings based on multiple cameras. The system automatically recognises pedestrian crossings and traffic lights. This is done by means of sensors and nine camera lenses, which convert the received image data fully automatically into appropriate commands in real time. For guidance at tricky spots, and if any uncertainty arises, a human remote operator located at the Starship control centre in Tallinn can connect to the robot over the Internet. The operator is able to view the camera images from the robot and also receives its navigation data, so as to help it out of a difficult situation by remote control. With every trip the parcel delivery robot “learns” more and more, so continually enhances its autonomy. Nevertheless, on their pilot runs the robots are permanently accompanied by a human guide, in order to gather as much information as possible on how they operate.
(Bildnachweis: Istockphoto: Altayb)
Ultra-precision surgery
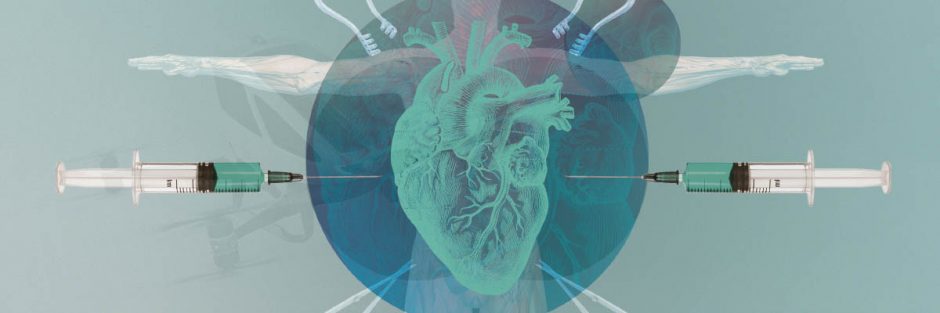
The STAR robot has performed operations on soft tissue with greater precision than a human surgeon – and entirely autonomously. An innovative tracking system allows it to compensate for any movements during the operation, such as in the muscle itself.
The use of robotic systems in operations is no longer something new; the da Vinci Surgical System has been used in hospitals around the world for several years already, to name just one example. Until now, however, the machines have still been controlled by doctors, making the systems in use little more than remote-controlled tools. Yet now there is a robot which can perform operations autonomously – that is to say, it does not require any input from doctors. The “Smart Tissue Autonomous Robot” (STAR) developed by researchers at the Children’s National Health System in the USA is even able to operate with greater precision than a human surgeon. While the robot needed more time to fulfil the task than its human counterpart, the results were extremely impressive. Surgeons and scientists from the Sheikh Zayed Institute in Washington demonstrated the robot’s capabilities during operations on dead pig tissue and living animals. STAR planned and implemented the suture autonomously under the watchful eye of a doctor.
Improving the quality of operations
STAR was specifically developed to operate on soft tissue such as muscles, tendons, ligaments, nerves, blood vessels and connective tissue. Around 44.5 million surgical procedures are currently carried out on soft tissue every year in the USA. “Our results demonstrate the potential for autonomous robots to improve the efficacy, consistency, functional outcome and accessibility of surgical techniques,” says Dr Peter C. Kim, Vice President and Associate Surgeon-in-Chief, Sheikh Zayed Institute for Pediatric Surgical Innovation. “The intent of this demonstration is not to replace surgeons, but to expand human capacity and capability through enhanced vision, dexterity and complementary machine intelligence for improved surgical outcomes.”
Previously, operations on soft tissue were entirely manual activities and were not able to be supported by robots. The main reason for this is that operations on soft tissue constantly entail unpredictable, elastic and plastic changes which require continuous adaptations on the part of the surgeons.
The system adapts in real time
STAR solves this problem with the help of a tracking system that combines near-infrared fluorescence markers and 3D light-field cameras. Plenoptic cameras also record the direction of incident light rays alongside the usual two image dimensions. This additional dimension affords plenoptic images extra information about the picture depth. Using this system, it is possible to precisely detect any movements and changes in the tissue in the course of the surgical procedure. The tracking system is combined with a smart algorithm which guides and controls the procedure, all the while performing real-time adaptations to changes in the tissue. What’s more, STAR is equipped with pressure-sensitive sensors, sub-millimetre positioning and powered surgical tools. Its robotic arm features a lightweight construction that permits movements with eight degrees of freedom.
Now that the robot has proven its efficacy, Dr Kim says that the next step is to reduce the size of the surgical tools further and improve the sensors in order to expand the range of application for the STAR system. He anticipates that the system – or certain aspects of the technology – could be ready for use in hospitals within two years.
Optimal orientation: future navigation
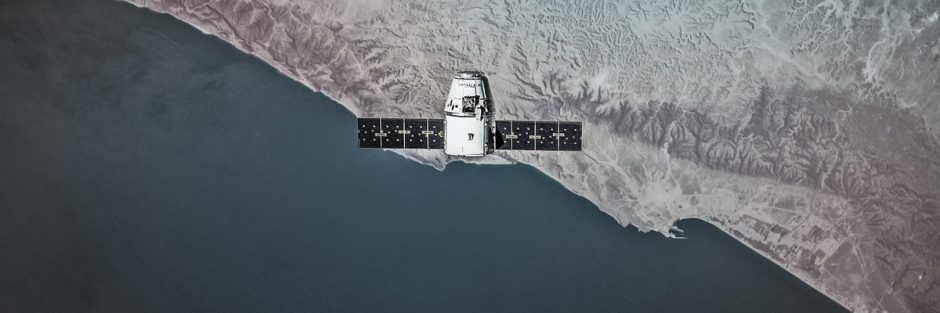
Where am I? Where am I going? And via what route? Those are the three key questions robots have to answer in order to move around autonomously within a given environment. In doing so, they employ a wide variety of different navigation solutions, which are frequently also combined, to ensure optimal orientation.
Robust autonomous navigation is essential to all mobile robot applications. For a mobile robot to be able to perform tasks such as “move to destination A” in different operating environments, it must be able to correctly identify its current location and to navigate. One of the basic methods used for this is global satellite navigation, as is also used in cars. The best known of them is without doubt the US Global Positioning System (GPS). Other countries have their own systems, too, including the Russian Glonass system, the emerging Chinese system Beidou, and Europe’s Galileo, which already has satellites in orbit. Satellite navigation offers positioning accuracy to within a few metres, depending on the number of satellites the given system can receive. As a result, robots are quite able to navigate through terrain, provided they have a map of the locality stored in their memory.
Satellite navigation with centimetre precision
To provide even more precise navigation, the signals from the satellites can be processed by software. US company Swift Navigation, for example, markets a software-based system which attains accuracy to within just a few centimetres using the standard positioning data from the satellites. Low-cost smartphone components provide the hardware. “This is not your cell phone’s GPS, which is accurate to about 10 feet. That’s good enough if you’re looking for a restaurant, but doesn’t come close to helping autonomous vehicles navigate the world,” said Tim Harris, CEO of Swift Navigation. “With our centimetre-accurate GPS, a car knows what lane it’s in, (…) and a drone can drop the package on your doorstep, not in your neighbour’s pool.” But satellite navigation does have one basic disadvantage: it stops working if the satellite signals are blocked, such as on a city street between skyscrapers, or inside a building. So a number of vendors have developed systems which replace the satellites with different signal sources.
Radio signals replacing satellites
For the indoor sector especially, Wi-Fi is a prime candidate: Wi-Fi routers, whose position and signal strength is known to the system, can be used to locate the position. In very densely populated areas, this enables a position to be located with an accuracy of 10 to 20 metres in just a few seconds. WPS (Wi-Fi Positioning System) is usually used in conjunction with other systems, such as GPS. Combining these systems creates a hybrid form of navigation which significantly improves the performance of the overall navigation system. Similar systems are also realised using Bluetooth technology.
A prime example in this context is Apple’s iBeacon technology. It involves placing small transmitters at predetermined points in a building, which are marked on a digital map. The transmitters send signals at fixed time intervals with unique identifiers. The data transfer runs via Bluetooth Low Energy technology, which means the transmitters can also be battery-operated. The receiving robot detects the transmitter’s identifier, measures its signal strength, and compares the data against the digital map. If it is receiving from four transmitters, it can even determine its location in three-dimensional space.
A still relatively new technology is Ultra Wideband (UWB): It involves installing transmitters which emit wideband signals of more than 500 MHz in the frequency range between 3.1 GHz and 10.6 GHz. If the robot knows the positions of the transmitters, it can determine its position by triangulation. The system devised by Irish company Decawave, for example, guarantees an accuracy of 10 centimetres on the basis of UWB technology. “The market for next generation indoor location technologies with improved accuracy is beginning to advance with solid use cases and adoption. UWB is clearly carving out its space, with ABI Research forecasting strong growth across a range of verticals,” said Patrick Connolly, Principal Analyst at ABI Research. “The market opportunity is quite large and companies like Decawave that are leading the charge in UWB are well positioned to experience continued growth.”
Navigation based on natural features
An alternative – or addition – to navigation by radio signals is offered by onboard systems which map the surrounding environment. They use radar or lidar. Lidar (Light Detection and Ranging) is a technique in which a pulse of light is emitted and a distance can be calculated based on the propagation time and speed of the light. Lidar is closely related to radar as a method of optical distance and speed measurement, though using laser pulses rather than the radio waves used by radar.
Ultrasound systems operate according to the same principle. They utilise special chips which emit sound waves in the ultrasonic range. If the waves encounter objects, they are reflected back and received again by the chip. The distance to the object can then be calculated from the propagation time of the sound wave.
Another method of 3D mapping is by stereo cameras: Colour and depth cameras generate a pixel cloud with exactly assigned distance values. On that basis, and by comparing against a previously created map, the robot is able to plot its position very accurately. Laser scanners are a solution for navigation typically employed in areas such as large-scale warehouses or in automotive applications. They are able to identify reflective targets in a 360 degree range by means of a rotating laser beam. A robot can determine its position by calculating the distance and angle to the target – provided it is able to cross-check the reference points against a previously created map of the surroundings. “This approach is today referred to as ‘natural feature navigation’,” says Nicola Tomatis, CEO, Bluebotics. The ANT (Autonomous Navigation Technology) system developed by the Swiss company achieves an accuracy to within one centimetre using conventional safety laser scanners for navigation. To do so, however, the system combines the data from the laser scanner with measurement values from additional sensors which monitor the movement of the robot. These may be sensors which monitor the rotary motion of the drive wheels, and so measure the distance travelled, or gyrometers which measure the robot’s rotary orientation.
Navigating by light
Robot manufacturer Adept has enhanced its indoor navigation systems with so-called “Overhead Static Cues” technology: If the surroundings have been altered so much – such as due to pallets or boxes lying around in a warehouse – that the measurement results fall below a certain probability of recognition, an upward facing camera can be optionally used to supply additional sensor data. The camera is oriented to the ceiling lighting for example. Based on a minimum of three visible ceiling lights, the position in the room can be additionally determined and the other sensory data corrected accordingly.
Researchers at Lund University in Sweden are also focusing on light. They have devised a concept for a new drone orientation system based on the evasion techniques of bees: The insects assess the intensity of light penetrating through holes in the dense undergrowth in order to evade objects. The researchers claim the system can be ideally tailored to small, lightweight robots. “I predict that our vision system for drones will become a reality in five to 10 years,” says Emily Baird from Lund University. As she points out, using light to manoeuvre around complex environments is a universal strategy which can be deployed by both animals and machines. Detecting and safely passing through openings is made possible as a result. “It is fascinating that insects have such simple strategies for solving difficult problems for which scientists have as yet not found solutions,” Emily Baird concludes.
(Picture Credits: Unsplash: NASA, SpaceX)
Smarter cleaning
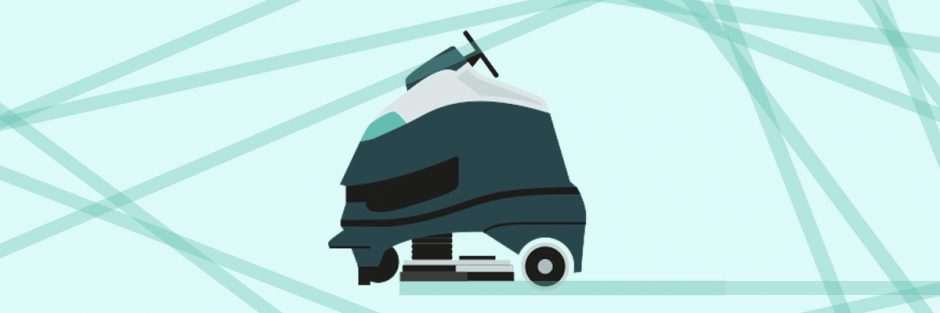
The cleaning industry suffers from a permanent shortage of personnel and a high staff turnover. This is where robots can help. Danish manufacturer Nilfisk is bringing out a machine in 2017 that can wet-clean rooms autonomously.
The interest in automated solutions has grown massively in the cleaning industry over the past few years. Cleaning robots can help the industry counter increasing cost pressure, personnel shortages and the customary high turnover of staff more effectively. What’s more, cleaning robots enable practically flawless results and improved productivity in light of the increased demand for results-driven cleaning. In 2017, Nilfisk – one of the leading manufacturers of professional cleaning appliances – will address this demand with the launch of the Advance Liberty A 50: a scrubbing robot which autonomously wet-cleans floors.
New prospects for the cleaning industry
The cleaning robot is the first result yielded by the Horizon programme – a joint venture between Danish firm Nilfisk and Carnegie Robotics, an American manufacturer of modern robotics sensors and software. The aim of the Horizon programme is to launch autonomous cleaning solutions onto the market and allow customers to clean floors precisely and reliably without any kind of human operation. “With this programme, we are paving the way for the long-term, strategic development of autonomous, networked cleaning solutions. Thanks to state-of-the-art technology, productivity and overall operating costs need to be viewed from an entirely new perspective,” says Jonas Persson, CEO of Nilfisk.
Military and space-grade technology built in
The cleaning robot incorporates a system comprising sensors, cameras and software, using which it can recognise a room by passing through it just once. Even obstacles the size of a tennis ball can be detected and independently avoided by the robot. This means that it can also be deployed on busy surfaces or during the opening hours of supermarkets, for example. The combination of sensors, cameras and lasers also enables the scrubbing robot to clean close to obstructions and walls. The sensor systems can also operate in low ambient light levels, meaning that the rooms which are to be cleaned don’t even need to be brightly lit. That saves on energy costs. “We’ve adapted military and space-grade technologies to provide the Advance Liberty A 50 scrubber with state-of-the-art perception and intelligent navigation that deliver safe and reliable floor cleaning,” says Steve DiAntonio, CEO of Carnegie Robotics. “At the same time, we’ve engineered a simple-to-use interface that enables flexible and efficient operation.”
Cleaning made simple
In the name of simplicity, the robot features just three buttons for setting the cleaning mode: in a building that’s still unfamiliar, the operator can first switch it to manual mode. While standing on the machine, they can guide the robot once through the entire room. In “Fill-in” mode, it’s enough just to delineate the outline of the surface. The robot subsequently cleans the entire surface and dodges every obstacle; even ones which have appeared in the meantime. Alternatively, the operator can also plot a specific course in “CopyCat” mode to show the robot which route it should follow when cleaning the room. “The Advance Liberty A 50 is our most important product innovation yet,” emphasises Nilfisk CEO Jonas Persson. “It will set the standard and lead the way for intelligent equipment going forward in the commercial cleaning industry.”
Robots in the military
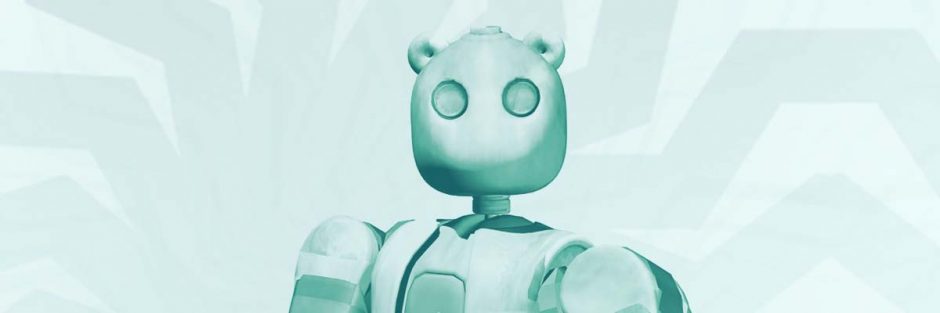
Robots are increasingly being deployed for military purposes, too. Until now, these robots have mainly been remotely controlled, but they are becoming ever more autonomous on the battlefield. The construction of fully autonomous systems which can make the decision to fire of their own accord is not a question of technology these days, but rather of ethics.
It’s no longer just the stuff of fantasy to imagine robots fighting on battlefields around the world. Nowadays, they are already exploring dangerous areas, defusing mines or recovering the wounded like the robotic medic Bear does. The Battlefield Extraction-Assist Robot developed by Vecna Technologies was conceived to rescue injured soldiers from combat zones without risking the lives of human medics. Work is currently in progress to integrate more autonomous capabilities into the robot. “The current generation of robots is dedicated to specific missions, and most are still remotely controlled,” says Thierry Dupoux, Research and Technology (R&T) Director at French manufacturer Safran Electronics & Defense. With its eRider, the company has developed a transport platform for the military that is reminiscent of a large quad bike. The vehicle can be controlled by a soldier – or move autonomously through the terrain. “We are using a complementary approach, inspired by the auto industry, which entails the rational and gradual introduction of autonomy functions. This approach can be applied to any modern transport, intelligence or combat platform.”
Robots still need firing orders
The trend towards increasingly autonomous combat robots is even viewed critically by military forces themselves, however. Up to this point, no army in the world has deployed robots that can make a decision to shoot on their own. This also applies for the drones that are currently the object of public concern. Robots like the SGR-1 – which is manufactured by a Samsung subsidiary, armed with a machine gun and deployed by South Korea to monitor the border with the North – require an explicit order from a human to fire. The weapons on the Maars military robot developed by QinetiQ are also triggered by remote control. Yet in terms of the technology, it is not too much of a stretch to imagine that combat robots will use their weapons entirely independently in the future – the decision as to whether robots should be granted this power is no longer a technical question, but a purely ethical one.
Relevant for the UN Weapons Convention
The annual conference to review the UN Weapons Convention in Geneva at the end of 2016 decided to address the topic of fully autonomous weapons that can select and attack their targets without any significant human intervention. “The governments meeting in Geneva took an important step towards stemming the development of killer robots, but there is no time to lose,” says Steve Goose, Arms Director of Human Rights Watch and Co-founder of the Campaign to Stop Killer Robots. “Once these weapons exist, there will be no stopping them. The time to act on a pre-emptive ban is now.”
(Picture Credits: Vecna Technologies)
Untiring warehouse operative
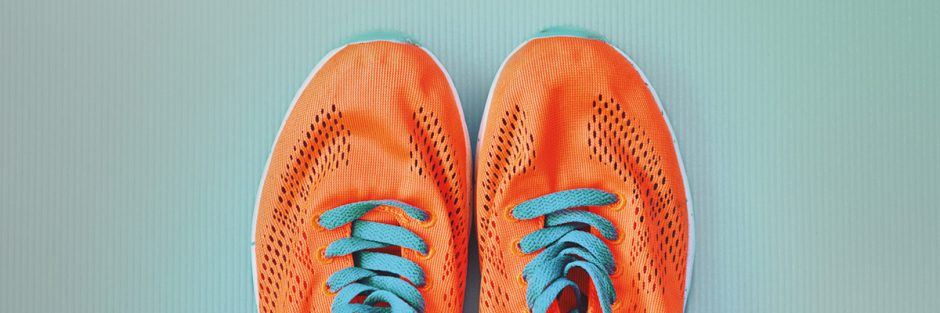
At the footwear warehouse of logistics service provider Fiege, autonomous robots pick online orders.
Since September 2016, logistics service provider Fiege has been employing three Toru Cube mobile robots at its footwear warehouse in Ibbenbüren, Germany, to pick online orders. Using its laser and camera system, the perception-controlled, network-connected robot from Munich-based start-up Magazino can autonomously localise and identify individual objects on shelves, pick them, and then transport them to their intended destination.
Flexible working with no rigid programme
Jens Fiege, Executive of the family business of the same name, comments: “Our aim in deploying new technologies is always to make our logistics processes faster and more efficient.” In fulfilling those aims, the robots the company operates possess a high degree of autonomy. When a robot sets off on a job, it does not yet know in detail what it is going to do, but instead decides on the way to its destination. Only once it is close enough to the storage location to actually see the ordered shoes does it make the final adjustments. It determines the position of the box, and from it derives its subsequent actions. Essentially, the robot does not follow a rigid programme, but rather applies a set of rules governing its behaviour which tell it what action is required under specific given conditions.
Robot detects individual objects
A further special feature of the picking robot is that it is not only able to pick complete load carriers, such as pallets, from the shelf, but even individual product items. Its laser sensors and 3D camera system enable it to autonomously detect individual objects on the shelf. Magazino has developed a proprietary object recognition method for the purpose. The system, known as Sheet-of-Light, projects a cross laser comprising two perpendicular laser lines onto the object it is detecting. A 2D camera captures the reflected laser beams and gauges the object based on the position of the lines in the camera image. The method is designed for cuboid objects. Curved surfaces, such as the spines of books, can also be detected. Fewer 3D points are generated than with a 3D camera, so much less computing power is required, meaning the algorithm can be run on a mini-computer for example.
“Our aim in the deploying new technologies is always to make our logistics processes faster and more efficient”
Jens Fliege, Executive, Fliege Logistik
Humans and robots working in parallel
Frederik Brantner, Co-founder and Commercial Director of Magazino, stresses: “It was important right from the beginning that the robots should be able to work in parallel with people. That means part of the picking process can be automated in a flexible, gradual way.” Its safety laser enables the robot to detect not only obstacles in its way but also human employees around it, while also orientating itself within the warehouse. There is no need for reflectors or marker lines on the warehouse floor. Once taught, the connected robot can also share with new robot “colleagues” self-created maps of its surroundings, as well as experience in handling specific objects or meeting particular challenges, by way of its wireless connectivity. The smart robot is not only capable of working with existing shelving systems, it can also adapt to new situations and changes within the warehouse.
(Picture Credits: Istockphoto: Martinina)
The gentle touch: Robotic grippers
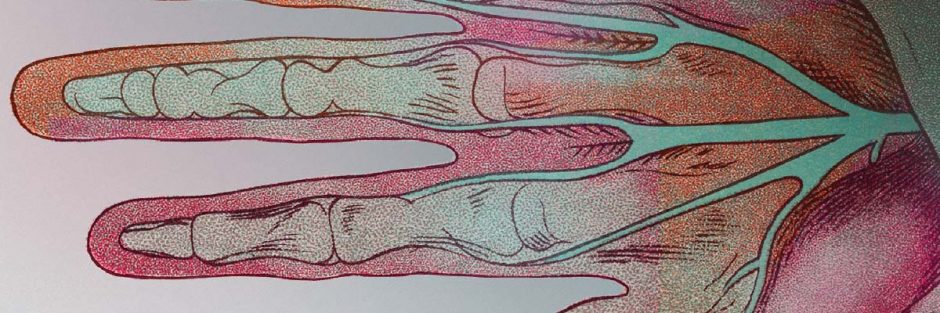
Versatile robots need grippers which are capable of both grasping objects forcefully, yet also handling sensitive items with care and delicacy. To that end, researchers are developing grippers which increasingly emulate the human hand not only in terms of shape, but also in their sense of touch.
The hand is a real miracle of nature. Its 27 bones, 28 joints, 33 muscles and thousands of sensory cells enable it to lift heavy loads as safely as raw eggs. Scientists and engineers have for years been striving to imbue robots’ gripping tools with the flexibility and versatility of the human hand. The need for such versatile gripping tools is becoming even more pressing due to the trend towards flexible production processes within the context of Industry 4.0 and the expansion of robot applications into the wide-ranging gripping tasks of everyday life outside of the factory.
And indeed, more and more solutions are being developed which are capable of at least copying the basic skills of the human hand. The key factors in achieving this are carefully applied gripping force and a sense of touch. The German company Schunk, for example, has already brought to market a gripper which uses specially devised gripping strategies and force measuring jaws in its fingers to adapt its responses in real time based on whether it is grasping an item for processing or possibly a human hand. But the Schunk gripper only has two fingers, meaning complex movements such as rotating or rolling a grasped object are not possible.
Robotic hands are learning to grasp correctly
“Hand manipulation is one of the hardest problems that roboticists have to solve,” says Vikash Kumar, a doctoral student at the University of Washington. “A lot of robots today have pretty capable arms but the hand is as simple as a suction cup or maybe a claw or a gripper.” Kumar is part of a team of computer scientists and engineers seeking to find solutions to these challenges. They have developed a robotic hand, with five fingers, 40 tendons, 24 joints and more than 130 sensors, which is able to execute human-like gripping movements. The scientists have provided this skilful hand with machine-learning algorithms. “There are a lot of chaotic things going on and collisions happening when you touch an object with different fingers, which is difficult for control algorithms to deal with,” comments Sergey Levine, assistant professor at the University of Washington. So the scientists devised an algorithm that models the highly complex behaviour of the five fingers on a hand and plans movements in order to achieve different results. During each manipulation task, the system uses its sensors and motion-capture cameras to collect data on the movements executed, which can then be continuously improved through machine-learning algorithms.
Handling even unknown objects safely
The system enables even complex gripping tasks to be realised. But what if the hand needs to pick up delicate objects such as a raw egg? That task was the focus of a gripper designed by the Computer Science and Artificial Intelligence Laboratory (CSAIL) at MIT. It consists of three soft silicone fingers which wrap around delicate objects, grasping them very safely and protectively. If it encounters a hard or unusually shaped object, it grips it using only its finger tips, providing for greater precision with increased point pressure. The gripper uses sensors to identify the shape of an object and so decide how to handle it. The robot’s processor unit is able to identify the object based on just three data points. To do so, the system compares the data against past gripping tasks. “If we want robots in human-centred environments, they need to be more adaptive and able to interact with objects whose shape and placement are not precisely known,” explains Daniela Rus, Head of the Distributed Robotics Lab at the CSAIL. “Our dream is to develop a robot that, like a human, can approach an unknown object, big or small, determine its approximate shape and size, and figure out how to interface with it in one seamless motion.”
Hairy sensors
This also requires new sensors which not only register the shape of an object, but can also detect whether it is soft, or whether it starts to slip because its grip is not firm enough. As one solution, the Tacterion corporation is developing tactile sensors in the form of a thin polymer-based artificial skin. Similar to the nerve cells in the human skin, its touch-sensitive sensors enable it to “feel” even the lightest pressure and process the corresponding data. Chinese researchers Rongguo Wang and Lifeng Hao at the Harbin Institute of Technology are working on the development of ultra-fine hair sensors for an electronic skin. The sensors are cobalt-based micro-wires encased in a thin layer of glass and embedded in a robust, rubber-like skin sensor. The hairy robot skin is able to detect a fly landing on it, the feel of a light breeze, or a five kilogram weight. The innovative sensor can also sense when an object it is gripping is starting to slip. “The sensor has a number of extraordinary capabilities, including the ability to detect air draughts, and to characterise material properties, as well as offering excellent robustness against damage,” the researchers claim.
(Picture Credits: Istockphoto: CSA Images)
Autonomous Mars Rover
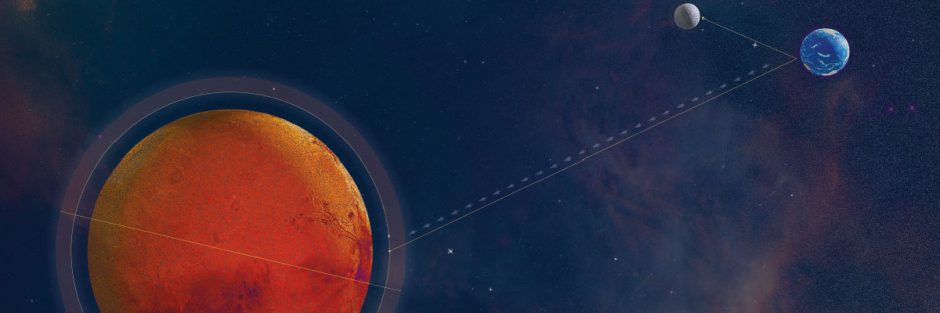
If we are going to conquer the universe, we will need help from autonomous vehicles, such as the HDPR Mars Rover. They have to travel over unfamiliar terrain without the possibility of human intervention – and must sometimes show a little courage as well.
As an extension of humans out in the wider universe, intelligent robots can change the future of space travel in the long term. Largely autonomous vehicles will land on celestial bodies, explore them, and thereby accelerate the exploration of the Solar System. High mobility, precise manipulation and the durability and reliability of the robotic systems are crucial factors for success.
“Remote control is not possible on Mars. That’s why a self-navigating rover is viewed as an essential technology for future missions.”
Levin Gerdes, robotics engineer, ESA
Special conditions require special chips
However, this requires custom-adapted electronics because the environmental conditions in space are much tougher than on Earth. Extreme temperature fluctuations, radiation and high acceleration forces are just some of the loads affecting devices and components. Cosmic radiation in particular – solar wind and other charged particles from the galactic background – can cause structural damage to the chips’ crystal lattice and lead to errors in computing processes. In order to avoid this, the chips have to be constructed using different methods to their terrestrial counterparts: for example, the silicon substrate used for conventional chips is replaced with aluminium oxide. This is due to the fact that silicon, unlike aluminium oxide, can lose its insulating effect under the influence of cosmic radiation. However, because these “toughening” measures are expensive and the chips cannot be manufactured in current production plants, old tried-and-tested chips are still in use. For example, NASA’s standard spaceflight processor, the RAD750, is based on a PowerPC 750 processor that was introduced in 1998. Although it operates reliably in a temperature range between -55 and 125 °C, and is resistant to a total radiation dose of up to 200,000 rad, it is also very slow compared to present-day standards. Its maximum performance is 200 MHz. By way of comparison, a modern chip operates at a clock frequency of up to 5 GHz.
Spacecraft with eyes and a brain
Autonomous vehicles, however, require fast processors. For this reason, NASA’s Goddard Space Flight Center demonstrated a new processor technology back in 2009: the SpaceCube processor, which is faster than the RAD750 by a factor of 10 to 100. To achieve this, the Goddard engineers have combined radiation-resistant circuits, which perform specific computing tasks simultaneously, with algorithms that can detect and repair radiation-induced errors in the data. This system is almost as reliable as the RAD750, but due to its considerably higher speed, it is able to perform complex calculations that have hitherto been limited to terrestrial systems. The processor is used in the Raven module, for example: in the future, this system will enable supply carriers to dock autonomously with the ISS. To this end, Raven is equipped with camera, infrared and lidar sensors, as well as algorithms for machine vision. “The sensors serve as the eyes. SpaceCube acts as the brain, analysing data and telling components what to do,” says Ben Reed, Deputy Division Director of SSPD.
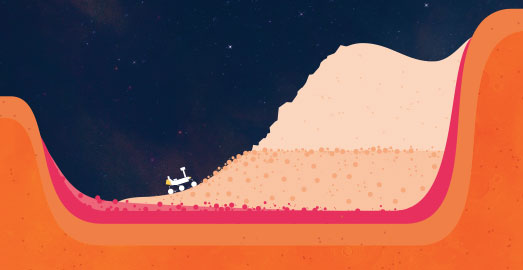
Humans can still intervene on the moon
These “toughened” chips are also essential for the rovers participating in the Google Lunar XPRIZE competition: the first privately funded team that succeeds in sending a rover approximately 400,000 kilometres to the moon, making it travel 500 metres, and having it transmit HD videos and images back to Earth by the end of 2017, will be awarded 20 million dollars. XPRIZE has recently confirmed that five teams have secured contracts to launch their spacecraft in 2017. Lunar vehicles such as the Tesla Prospector and Surveyor from the Synergy Moon Team are already able to perform basic autonomous functions. They can monitor themselves and carry out exploration missions autonomously. However, there is always the option of controlling them remotely from Earth.
Left to their own devices on Mars
“The Moon is close enough for direct remote control, albeit with a slight time delay,” explains robotics engineer Levin Gerdes from the European Space Agency (ESA). “But for Mars, the distance involved makes that impossible. Instead Martian rovers are periodically uploaded with sets of telecommands to follow. This is a slow process, however. A faster, self-navigating rover is seen as a necessary technology for future missions, like self-driving cars on Earth. But with no roads, the rover will have to work out its own route – first by taking images, then by using these to map the surrounding area, followed by identifying obstacles and planning a path to safely reach its assigned goal.” Gerdes proved that this is indeed possible with an ESA team in Tenerife in summer 2017: the Teide National Park provides a moon-like surface with sand and small boulders, enabling the ESA team to test the autonomous navigation of their Heavy Duty Planetary Rover (HDPR) under realistic conditions. With its seven cameras and sensor data from time-of-flight cameras, lidar and GPS systems, the vehicle actually managed to navigate on its own on the difficult terrain. “We managed a number of runs, the longest of which was more than 100 m – only for the rover to finally inform us that its assigned destination was unreachable, which turned out to be true. There were some slopes which were too steep to guarantee a safe traverse.”
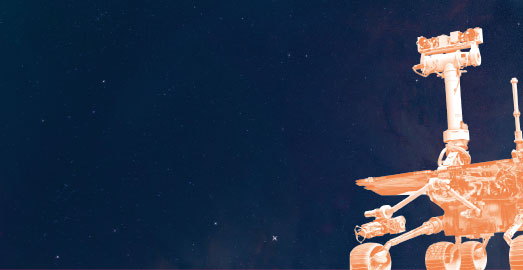
Taking some bold decisions
But safety is not always the top priority: sometimes, a successful mission is considered more important than potentially damaging the hardware. An autonomous Mars Rover must therefore also dare to take risks. And this is precisely what the scientists working on the intelliRISK project want to teach their robots: they should be able to independently assess risks and consciously evaluate situations in order to make decisions. The project uses Lauron, a walking robot developed at the FZI Research Center for Information Technology at the Karlsruhe Institute of Technology, which is capable of moving safely on rough terrain. With its programmed courage, the robot must be able to detect, assess and consciously take risks when confronted with a steep slope or a wide ditch, for example. At the beginning of the mission, the robot may act with caution and restraint but later on, towards the end of its service life, it will be able to make bolder decisions. “The intelliRISK project is making an important contribution to increasing autonomy in the field of robotics,” says Arne Rönnau, who is responsible for the project. In addition to space travel, the system could also be used in other fields. “In the future, robotic risk awareness could also be used in Industry 4.0 applications in order to enhance occupational safety while working with humans and avoid accidents,” says the robotics expert.
(Picture credit: istockphoto: Tokarsky; NASA)
Artificial Intelligence in autonomous vehicles
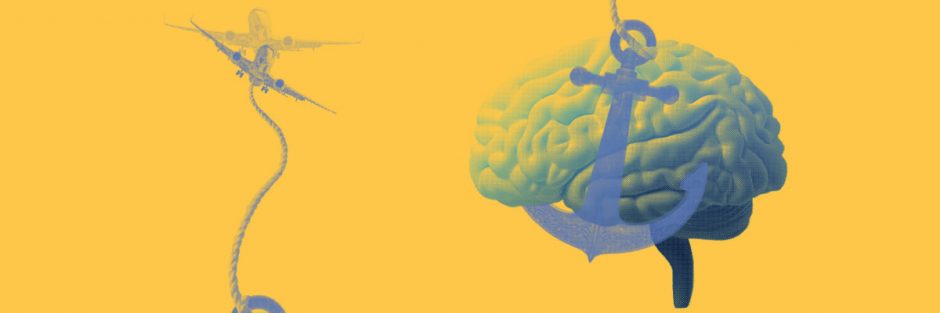
Artificial intelligence is a crucial technology for autonomous vehicles. Adaptive control systems make it possible to process the immense data sets delivered by the surrounding area sensors, then work out which actions should be taken.
For a vehicle to drive autonomously, it is not enough to simply equip it with a large number of sensors for detecting the immediate surroundings. It must also be able to handle the huge volumes of data, and to do so in real time. This overburdens conventional computer systems. The solution comes from electronics and software that provide the means for imitating the functions of the human brain. Artificial intelligence (AI), cognitive computing and machine learning are terms used to describe different aspects of these types of modern computer systems. “In essence, it is all about emulating, supporting and expanding human perception, intelligence and thinking using computers and special software,” says Dr Mathias Weber, IT Services Section Head at the German digital industry association Bitkom.
Growing Demand
Nowadays, artificial intelligence is used as standard; for instance, it is embedded in digital assistants like Siri, Cortana and Echo. The basic assumption of AI is that human intelligence results from a variety of calculations. This allows artificial intelligence itself to be created by different means. There are now systems whose main purpose is to detect patterns and take appropriate actions accordingly. In addition, there are variants known as knowledge-based AI systems. These attempt to solve problems using the knowledge stored in a database. In turn, other systems use methods derived from probability theory to respond appropriately to given patterns. “An artificial-intelligence system continuously learns from experience and by its ability to discern and recognise its surroundings,” says Luca De Ambroggi, Principal Automotive and Semiconductor Analyst at IHS Technology. “It learns, as human beings do, from real sounds, images, and other sensory inputs. The system recognises the car’s environment and evaluates the contextual implications for the moving car.” In terms of AI systems built into infotainment and driver assistance systems alone, IHS expects sales to increase to 122 million units by 2025. By comparison, the 2015 figure was only 7 million.
New Processors for Artificial Intelligence
The roll-out of artificial intelligence also has direct impacts on processor technology: conventional computational cores, CPUs, are being replaced with new architectures. Graphics processing units (GPUs) have thus been viewed as a crucial technology for AI for several years. CPU architectures perform tasks as a consecutive series, whereas GPUs – with their numerous small and efficient computer units – process tasks in parallel, making them much faster where large volumes of data are concerned. The new chips’ control algorithms already contain elements of neural networks, which are used in self-learning machines. A neural network of this type consists of artificial neurons and is based on the human brain in terms of its workings and structure. This enables a neural network to make highly realistic calculations.
Tyres with AI
In 2016, tyre manufacturer Goodyear introduced the concept of a spherical tyre featuring artificial intelligence. With the aid of a bionic “outer skin” containing a sensor network, along with a weather-reactive tread, the tyre can act on the information it collects by directly implementing it in the driving experience. It connects and combines information, processing it immediately via its neural network, which uses self-learning algorithms. This allows the Eagle 360 Urban to make the correct decision every time in standard traffic situations. Its artificial intelligence helps it to learn from previous experiences, enabling it to continuously optimise its performance. Consequently, the tyre adds grooves in wet conditions and retightens when dry.
Adaptive Control Systems
Like human beings, cognitive computing systems can integrate information from their immediate surroundings – though rather than eyes, ears and other senses, they use sensors such as cameras, microphones or measuring instruments for this purpose. The new processor architectures give vehicles the ability to evaluate these huge data volumes, and to constantly improve and expand these evaluations. This machine learning is seen as a key technology on the road to artificial intelligence. Machine learning also includes deep learning, which interprets signals not by relying on mathematical rules, but rather knowledge gained from experience. In this case, the software systems change their programming by experimenting themselves – the behaviour that leads most reliably to a desired result “wins”.
Several automotive suppliers are now offering control systems pre-equipped with deep learning capabilities. Contemporary electronic control units (ECUs) in vehicles generally consist of various processing units, each of which controls a system or a specific function. The computing power of these units will no longer be adequate for autonomous driving. AI-based control units, on the other hand, centralise the control function. All information from the various data sources of an autonomous vehicle – including from infrastructure or from other road users – are gathered here and processed with a high-performance AI computing platform. In this way, the control system comes to “understand” the full 360-degree environment surrounding the vehicle in real time. It knows what is happening around the vehicle and can use this to deduce actions. Jensen Huang, CEO of Nvidia, works with his company to partner with various automotive manufacturers in developing control systems of this type. He is certain of one thing: “Artificial intelligence is the essential tool for solving the incredibly demanding challenge of autonomous driving.”
(picture credit: istockphoto: BlackJack3D, Feverpitched)
Cybersecurity – Protection for autonomous vehicles
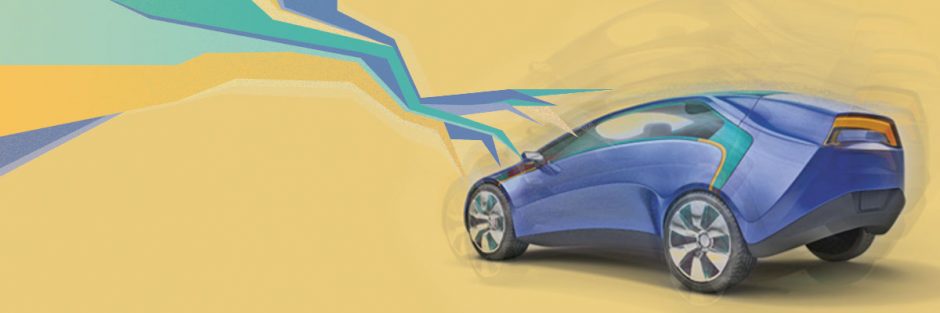
As connectivity advances, protection of vehicles against attacks from cyberspace is becoming ever more important. The only way to assure such protection is with a comprehensive cybersecurity concept, integrated into the development process right from the start.
Autonomous vehicles are nothing other than mobile computers with innumerable communications interfaces – exchanging data with infrastructure or other vehicles, updating on-board software, or accessing real-time navigation maps. However, the increasing number of interfaces aboard vehicles also means there are more potential vulnerabilities for cyber-attacks. “Hundreds of articles on autonomous driving appear in the media every day, but almost none mention the elephant in the room: auto-makers do not yet have a reliable defence against cyber-threats. Period. One serious hack could immediately halt progress in automated driving. But we have the remedy,” says David Uze, Trillium’s CEO. Consequently, the Japanese company founded in 2014 is planning to launch a software-based, multi-layer security solution onto the market in 2018 – at a tenth of the cost of existing solutions. “Since defence must continually evolve, our infrastructure will deliver Security as a Service (SaaS) via real-time-update platforms that auto-makers or insurers can on-sell to car owners.”
There is no wonder-weapon
Whether a purely software-based add-on cybersecurity solution is enough on its own to protect a vehicle against the highly sophisticated attacks of modern-day hackers is questionable, however. “There is no wonder-weapon capable of protecting cars against sophisticated dynamic cyber-attacks,” stresses Ofer Ben-Noon, co-founder and CEO of Israeli vehicle cybersecurity company Argus. “Our customers need protection on multiple levels, so as to be prepared for any conceivable scenario.” The company offers a multi-layer security solution for connected vehicles: it starts with the infotainment and telematics devices, encompasses the internal network communications, and also extends to selected electronic control units (ECUs). ECU security protects vital systems such as the brakes, assistance systems and other key units against attack.
Cybersecurity as part of the development process
Cybersecurity should be integrated into the development process for an autonomous vehicle right from the start as a matter of policy. That principle is affirmed in a manifesto published by FASTR, stating that cybersecurity should begin at the very foundation of the vehicle’s architecture and be coordinated throughout the supply chain. In that way, a connected vehicle can be made “organically secure”. FASTR – which stands for Future of Automotive Security Technology Research – is a neutral, non-profit consortium established in 2016 by three companies: Aeris, Intel Security and Uber.
The provision of such all-round protection should be approached according to the bottom-up principle: the security concept starts with a high-security core (root of trust), implemented by a physically secured cryptographic device such as a Hardware Security Module (HSM). It securely holds cryptographic keys and algorithms, protecting them against being read, modified or deleted. The keys are in turn used to detect and prevent manipulation of the ECU firmware. This then also ensures that the software-based security functions in the firmware can be used safely for on-board communications. At the same time, it means on-board networks on different security levels are reliably isolated from one another – preventing access to the engine management system via an entertainment interface, for example. The secure on-board network this creates then also in turn permits secure communication with other vehicles or infrastructure.
Autonomous vehicles are protected against the many types of cyber-attack by multi-layered security concepts.
Hardware security module
More secure E/E architecture
Secure on-board communication
Secure vehicle IT infrastructure
Secure control unit
Secure V2X communication
Security systems must be updateable
The challenges of cybersecurity are changing continually, however. Security experts are continually having to confront new conditions and methods of attack. That means a vehicle’s on-board security systems must be capable of being regularly updated throughout the product life-cycle. Consequently, a security solution for autonomous vehicles should be designed right from the start in such a way that vital security parameters and functions are held in modifiable storage devices (such as HSMs with firmware update facilities). Also, available IT resource capacities should not be fully utilised right from the start – leaving adequate spare storage space, for example. With appropriate update mechanisms, new security patches can then be downloaded “over the air” – and the vehicle can stay protected against attacks from cyberspace even ten years down the line.
“Car hacking is a very real threat that will continue to increase as we move towards greater connectivity and autonomous vehicles, with more and more new technologies becoming part of the Internet of Things,” says Saar Dickman, Vice President, Automotive Cyber Security at Harman. “Automotive cybersecurity is an increasingly critical piece in enabling connectivity and autonomous driving.”
(picture credit: istockphoto: Kodochigov)
Communication in autonomous vehicles
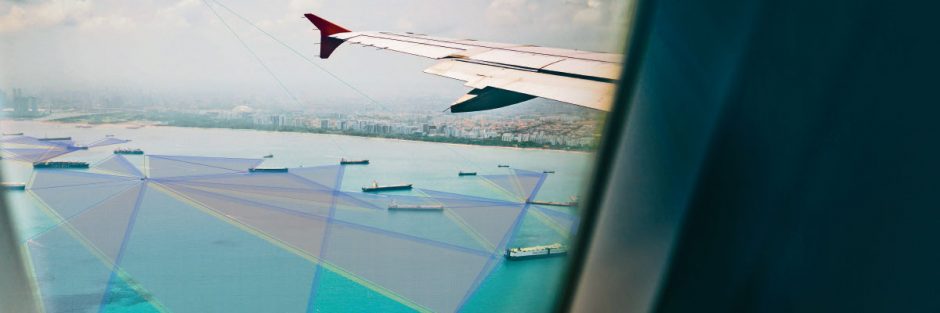
Communication in autonomous vehicles will be a key technology in autonomous driving. They can benefit from the other vehicles’ experiences through the cloud, download data that they have gathered onto freely accessible maps in real time and send danger warnings to their surrounding area via WLAN.
Nowadays, sensor systems such as lidar, radar and cameras can already provide a highly precise image of an autonomous vehicle’s environment. These vehicles can react to what is happening around them in combination with artificial intelligence and complex algorithms – almost like a human being. Yet like people, the vehicles can only use these sensors to react to things in their field of view. Consequently, to be able to “look around the corner”, autonomous vehicles will have to be connected and capable of communicating with their surrounding area and infrastructure.
“The transfer of data to vehicles is a core technology for automated driving, for real-time application, as well as for maintenance and service,” Armin G. Schmidt, CEO of Advanced Telematic Systems (ATS), emphasises. The German software company focuses on connected vehicles and develops solutions for the future of mobility, for which it relies on the establishment of industry-wide standards and open-source technology. “The exchange of data between vehicles, such as between different provider platforms, also requires further standardisation development,” Schmidt continues.
Warning the Surrounding Area in Milliseconds
Standard ITS-G5 of the European Telecommunications Standards Institute (ETSI) has been established for the communication of vehicles in road traffic. This is a variant of the IEEE 802.11 WLAN standard that has been optimised for data exchange between vehicles and has since also been recognised in the United States. It uses the 5.9-GHz frequency band and makes communication possible over short distances in close to real time. The technology ensures a reliable transfer at a high vehicle speed, as well as allowing direct communication between individual vehicles (car-to-car) and between the vehicle and infrastructure (car-to-X, vehicle-to-X or V2X) without a router. This means that sudden events or hazardous situations can be communicated to the surrounding area within only a few milliseconds. For example, if a car has been involved in an accident or a bank of fog forms ahead, it can automatically warn approaching traffic heading in the same direction. “The benefits of safety and awareness of V2X as a sensor – with its ability to ‘see around the corner’ – have already been proven beyond doubt as a means to providing relevant and reliable early warning messages for advanced driver assistance systems,” explains Jozef Kovacs, CEO of US firm Commsignia, a provider of software and hardware solutions for connected cars.
In the future, however, road vehicles will most likely not only be equipped with communications technology. Initial solutions are implementing an “adaptive hybrid network concept”. This includes integrating various wireless technologies – such as ITS-G5, LTE mobile communications or 60-GHz technologies – into a communications stack (the waveform and the typical receiver algorithms of the IEEE 802.11ad WLAN standard are used here, providing a common framework for vehicle communication and radar technologies at 60 GHz). The ideal communication technology is selected adaptively, meaning that it depends on the situation in real time. Criteria for selecting wireless technology include its predicted availability or its signal quality.
Quantity of data generated each day by an autonomous vehicle.
Source: IntelCameras: 20-24 MB each second
Lidar: 10-70 MB each second
Sonar: 10-100 KB each second
Radar: 10-100 KB each second
GPS: 50 KB each second
To the Cloud with 5G
Wireless technologies offer the advantage of unlimited range, whereas ITS-G5 can only bridge distances of up to one kilometre. For example, LTE networks are already in use in the automatic emergency call system ECall. Though yet to be implemented, the 5G network will assume this role in the future. “We expect 5G to become the worldwide dominating mobile communications standard of the next decade,” says Dr Christoph Grote, Senior Vice President of Electronics at BMW, adding that: “for the automotive industry, it is essential that 5G fulfills the challenges of the era of digitalisation and autonomous driving.” For this reason, BMW, along with Audi, Daimler, Ericsson, Huawei, Intel, Nokia and Qualcomm, founded the 5G Automotive Association which aims to develop, test and promote communications solutions. “Cloud, communications and networking technologies and innovations have the potential to transform the car into a fully connected device to revolutionise the driver experience and address society’s mobility needs,” explains Dr Marc Rouanne, Chief Innovation & Operating Officer at Nokia.
“Cloud, communications and networking technologies and innovations have the potential to transform the car into a fully connected device to revolutionise the driver experience.”
Dr Marc Rouanne, Chief Innovation & Operating Officer, Nokia
Swarm Data Paves Way for Automated Driving
These types of technologies, for instance, provide the means to share the experiences that an adaptive autonomous vehicle has gained with other vehicles via the Cloud. Cloud technologies are also opening up new possibilities for navigation. By way of example, Mobileye, an Israeli company that creates accident prevention and automated driving technologies, has developed the camera-based mapping and localisation technology Road Experience Management (REM). Real-time data from numerous vehicles – a swarm of cars – is collected via crowdsourcing before being used for precise localisation and to record high-definition lane data. To accomplish this, the vehicles deploy optical sensor systems to detect road markings and road information, which flow to the Cloud in compressed format. This fleet data is used to continuously improve HD navigation maps with high-precision localisation capability and, in turn, is a basic necessity for automated driving and for assistance systems to be further developed. “The future of autonomous driving depends on the ability to create and maintain precise high-definition maps and scale them at minimal cost,” co-founder and Chief Technology Officer of Mobileye, Prof. Amnon Shashua, summarises. At the start of 2017, Mobileye made an agreement with the Volkswagen Group to implement a new navigation standard for automated driving from 2018 onwards. Future Volkswagen models will use REM. The agreement will facilitate the worldwide consolidation of data from different automobile manufacturers into an “HD world map”, the first of its kind. That will form an industry-wide standard. According to Dr Frank Welsch, Member of the Board of Management of the Volkswagen brand with responsibility for development: “Every day, millions of Volkswagen vehicles drive on our streets. Many of them are equipped with sensors to monitor the surroundings. We can now utilise this swarm to obtain various environmental data in anonymised form related to traffic flow, road conditions and available parking places, and we can make these highly up-to-date data available in higher-level systems. More services are planned which will be able to utilise these data and make car driving and mobility easier with greater convenience and comfort overall.”
(picture credit: Unsplash)
Logistics revolution: Transport Robots
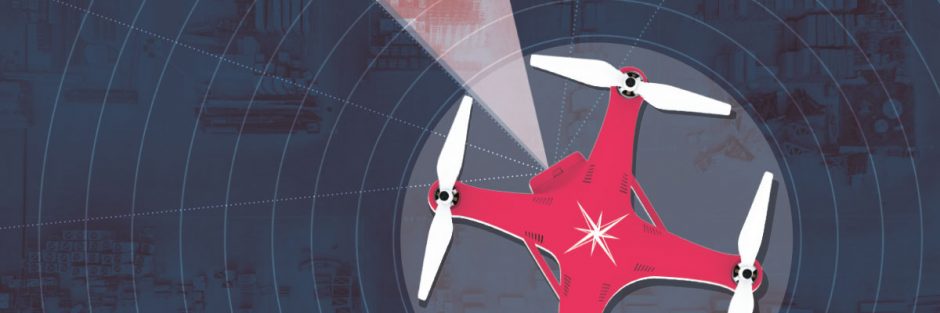
Thanks to autonomous navigation, transport robots inside factories and production facilities are now becoming as versatile and flexible as a human solution.
The ability to navigate autonomously will revolutionise the world of in-house logistics. That’s what the market analysts at IDTechEx say, anyway. “Automated guided vehicles barely made a dent in this industry. This is because their navigational rigidity put a low ceiling on their total market scope,” says Dr Khasha Ghaffarzadeh, Research Director at IDTechEx. “Autonomous mobile robots are radically different, however, because they will ultimately enable automation to largely keep the flexibility and versatility of human-operated vehicles.” It is his opinion that mobile robotics in material handling and logistics will become a 75 billion dollar market by 2027. It will then more than double by 2038.
Immediate increase in productivity
We are still very much in the starting phase. Mobile robots are still relatively expensive. However, there are already some examples of practical applications. A Jungheinrich APM (Auto Pallet Mover) has been in use at Hero España, a Spanish manufacturer of baby food and jams, since 2011. The APM is a standard high-level order picker or pallet truck that has been modified with an automation package, transportation control software and a personal protection system. The vehicle transports pallets made ready in the automated warehouse to the picking stations and from there to the dispatch area. While the vehicle is in motion, a rotating laser scans the surrounding area, using reflectors that are positioned along the route to determine the exact position of the Auto Pallet Mover. The layout of the warehouse and the routes taken by the vehicle can be changed in a matter of minutes without having to adjust the reflectors. Sensors on the forks also enable the pallets to be picked up or set down up to a height of two metres in autonomous mode. Juan Francisco García Gambín, Warehouse Manager at Hero España: “Since the Jungheinrich APM was commissioned, the increase in productivity has been noticeable from the very first day as a result of the autonomy with which the machine carries out its tasks.” Soon after the system was commissioned, Hero España started to notice higher efficiency levels in its intra-logistical processes as well as a marked reduction in errors associated with goods-in, goods transfer and dispatch tasks.
Transport robots are a milestone in digitalisation
Mobile robots are also being used for transportation purposes at the BMW plant in Wackersdorf, Germany. Ten of these Smart Transport Robots move components around in warehousing and production. Able to measure the distance to wireless transmitters and equipped with an accurate digital map of the production hall, the robot can calculate its exact position and this route to its destination. The battery-powered transmitters are mounted on the walls of the hall. The solution is flexible and can be extended to other logistics areas relatively inexpensively. The vehicle’s sensors also enable it to identify and respond to critical situations so that it can share the route with human personnel and other vehicles. A future phase of development will see the introduction of a 3D camera system to make navigation even more accurate. “The development of the Smart Transport Robot is an important milestone for the BMW Group when it comes to digitalisation and autonomisation in production logistics. This innovation project makes an important contribution to the agility of the supply chain in logistics and production. It enables the supply chain to adapt to changing external conditions quickly and flexibly,” comments Dr Dirk Dreher, Vice President of Foreign Supply at the BMW Group.
Despite Transport Robots: Challenging tasks are the preserve of human beings
Tobias Zierhut, Head of Product Management Warehouse Trucks at Linde Material Handling, believes that warehouse workers will benefit too: “In the future, automated vehicles will be able to take over simple tasks that are repeated at regular intervals. This will enable human personnel to concentrate on more challenging and complex tasks.” Since 2015, Linde Material Handling has been working in close collaboration with Balyo, a French robotics specialist, on developing and producing automated vehicles. The vehicles do not rely on reflectors, induction cables or magnets installed in the warehouse. As part of the installation process, a map of the area in which the vehicles will be used is created. This information is transferred to the vehicle, which automatically positions itself using invisible laser-controlled geonavigation.
Stocktaking with a drone
Balyo and Linde have also developed a drone to be used for stocktaking in warehouses. Approximately 50 centimetres wide, equipped with six rotors, a camera, a barcode scanner and a telemeter, the “Flybox” stocktaking drone slowly makes its way up the front of each individual rack, taking a photo of every pallet storage location and capturing the barcodes of the stored goods. “The decisive new feature of this invention is that we use the drone together with an autonomous industrial truck,” says Zierhut. During its stocktaking mission, the drone is guided by an automated pallet stacker. The two vehicles are connected via a self-adjusting cable. With this innovative coupling, Linde has resolved two challenges that have hitherto impeded the use of drones in warehouses: power supply on one hand (drone batteries usually only last 15 minutes), and the localisation under the hall roof without GPS reception on the other.
Autonomous Aircraft: A new way to fly
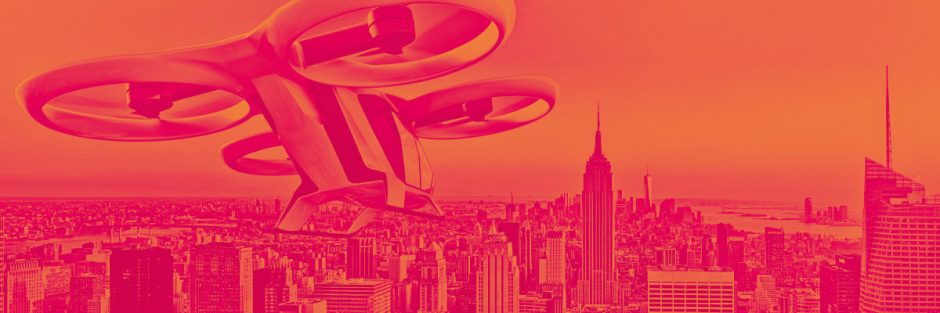
The technology that will allow autonomous aircraft to fly without pilots is here and is already undergoing successful testing. This may mean a whole new way of travelling, in particular when it comes to mobility in cities.
Now that we have driverless cars and increasingly intelligent drones, an autonomous aircraft without a pilot on board hardly seems visionary any more. In fact, as far back as some ten years ago, the IFATS (Innovative Future Air Transport System) project demonstrated the technical feasibility of aircraft without pilots, although it was thought at the time that this would not happen before 2050. The biggest hurdle was considered to be passenger acceptance. However, acceptance levels are likely to increase every time there is a pilots’ strike or an aviation accident, because up to 90 per cent of aviation accidents are attributable to pilot error. This explains why all large aircraft manufacturers – along with many small start-ups – are working on unmanned aircraft.
90% Pilot Error: The majority of all aviation accidents can be traced back to human error. It is hoped that autonomous systems will considerably reduce this figure.
Nothing escapes the electronic eye
British manufacturer BAE Systems started testing a pilotless aircraft at the end of 2016. A standard Jetstream 31 plane was converted to provide a flying test bed. The machine has an antenna which detects transponder signals from other aircraft as well as a cockpit-mounted camera acting as an electronic eye. This links to the aircraft’s computer systems and enables the Jetstream to “see” potential hazards, even if no signals are being emitted. The electronic eye of the Jetstream can also recognise different cloud types and, if needed, plot a course that allows evasive action from challenging weather conditions. Pilots on board the aircraft remain responsible for take-off and landing, but as soon as the Jetstream is in the air, it flies autonomously. It has already clocked up almost 500 kilometres of flying at a height of approximately 4.5 kilometres. “Our priority, as always, is to demonstrate the safe and effective operation of autonomous systems,” explains Maureen McCue, BAE Systems’ Head of Research and Technology for the military aircraft and information business. “The trials will give us technology options that could be applied to our own manned and unmanned aircraft, as well as potentially enabling us to take some new unmanned aircraft technologies to market.”
Requiring continuous corrections about all three axes, a helicopter is much more difficult to control than a fixed wing aircraft like the Jetstream. Yet for this type of craft too, the first test flights are already under way. In June 2017, Airbus Heli-copters started test flights with a preliminary study involving the VSR700. The prototype of the light optionally piloted vehicle (OPV) helicopter is due to take off in 2018. Optionally piloted means that the machine can fly both autonomously and with a pilot. “The OPV is able to autonomously take-off, hover and perform stabilised flight and manoeuvres,” said Regis Antomarchi, Head of the VSR700 programme at Airbus Helicopters. This phase of flight trials with a safety pilot will focus on refining the automatic flight control system aboard the helicopter, eventually leading to fully autonomous flights without a safety pilot.
A new dawn for local transport
Airbus actually wants to go much further with autonomous aircraft. The Group has a number of visions associated with this technology in its test laboratories, with the focus being on the urban traffic of the future. A3, a subsidiary company based in Silicon Valley, is currently working on a project called Vahana. Vahana is a self-piloted flying vehicle platform for individual passenger and cargo transport – an air taxi, essentially. “The ability to be transported safely and quickly through a city in a self-piloted aircraft is no longer science fiction,” says Rodin Lyasoff, CEO of A3. “Advances in propulsion, battery performance, air traffic management, autonomy and connectivity mean that this mode of transportation is capable of benefitting millions of people in years, not decades.” In his view, the only remaining challenges to be overcome are associated with reliable sense-and-avoid systems, which detect hazards or obstacles and invoke evasive action. At the current time, there are not yet any fully developed solutions for aviation. “Urban air mobility will significantly change how we live and work for the better, but bridging from feasibility to reality will require close cooperation between the public and private sectors to define appropriate regulations,” says Lyasoff. Another Airbus vision for urban air traffic is the City Airbus. In terms of technology, this aircraft is comparable to a small drone. Like a drone, it relies on several electrically driven propellers to stay in the air. Where it differs from a drone is that it will be designed to carry several passengers. To speed up its time to market, the machine will initially be controlled by a pilot. The intention, though, is that it will fly autonomously at a later point in time and passengers will use an app to hail a ride. A feasibility study has already been successfully completed.
(Picture credit: Airbus)
Radar technology in autonomous vehicles
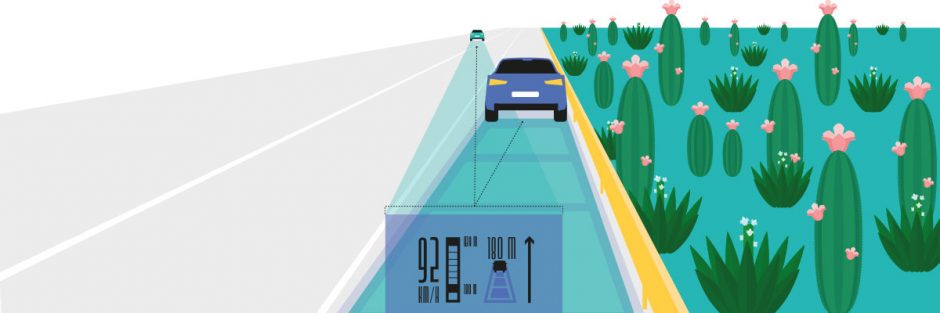
Radar Technology is nothing new. Yet in view of current developments, radar technology in autonomous vehicles is becoming ever more precise and powerful.
Radar is a fundamental part of the automated driving equation,” explains Peter Austen, Global Portfolio Director, Driver Assist Systems at ZF’s Active & Passive Safety Technology division – or ZF TRW for short. “When combined with cameras, intelligent control units and actuators, it can help to enable partially automated driving functions.” ZF TRW has been designing and developing radar in Brest since 1999.
Luxury early on
As early as the start of World War II, radar technology was being used on board aeroplanes and ships. Despite this, its first appearance in cars wasn’t until 1998, when Mercedes-Benz introduced a distance radar in the S-Class. Still, the cost of this technology was very high at the time, owing to the fact that until 2009, the required semiconductors could only be manufactured using gallium arsenide (GaAS) – an expensive and difficult material to process. A further disadvantage is the low level of integration; the ability to combine an increasing number of functions on an unchanging chip area. Only with the manufacturing of sensors in silicon germanium technology (SiGe) – the most frequently used materials for semiconductor manufacturing – did the systems become affordable. This enabled tried-and-tested standard processes to be implemented in mass production for the first time. What’s more, a whole host of functions could now be condensed onto just two SiGe components, where previously up to eight GaAs chips had been required.
Detection is becoming ever more powerful
Radar systems applied in autonomous vehicles work with millimetre precision – nowadays in the range of either 24/26 GHz or 77/79 GHz. This means that high resolutions are possible for detecting objects and determining their position and movement with centimetre accuracy. When compared to other technologies, such as camera-based methods, the radar works reliably even in conditions of poor visibility such as snow, fog, torrential rain and dazzling backlight. What’s more, the complete systems are not much bigger than a matchbox.
One fundamental difference can be drawn between two types of radar: the frequency-modulated continuous-wave (FMCW) and the impulse radar. Unlike an impulse radar, which emits only one pulse, FMC radars transmit pulses continually. With the FMCW method, the signal is modulated over the entire range during transmission, meaning that the frequency varies over time – this is called chirp. This chirp is repeated in cycles and enables FMC radars to simultaneously measure the absolute distance between the transmitter and object in addition to the differential speed between the two. The devices have admittedly had a weakness – until now: if objects approach at different speeds, it is possible that the radar might “overlook” one of them. Previous devices could therefore only reliably detect objects up to a relative speed of 50 km/h. One solution is to increase the modulation rate. What is referred to as fast chirp modulation (FCM) brings about an increase in the accuracy of distance measurement, enabling a wider range of target object speeds to be covered. Yet an increase in outside temperature causes the pulses of standard CMOS signal generators to slow, resulting in errors. To tackle this issue head-on, Fujitsu released a CMOS-based millimetre-wave signal generator in late 2016, which is capable of maintaining its modulation rate reliably and precisely even at temperatures of 150° Celsius. This allows detection errors to be reduced and even objects approaching the vehicle at a relative speed of 200 km/h are reliably detected.
Over 100 years old
Yet radar technology is in fact much older: it was as early as 1904 in Düsseldorf that the German engineer Christian Hülsmeyer developed the first practical application for the reflection of electromagnetic waves on objects – the Telemobiloscope. Similar to modern radar sensors, it transmitted focussed electromagnetic radiation – radio waves. In modern radar systems, analysing the reflected radiation allows objects to be detected along with their respective distance and speed.
Radar navigation
Despite all this, radar devices are not used in autonomous vehicles merely to detect and locate objects. At some point in the future at least, radar is intended to be used for navigation. With this in mind, Bosch and the Dutch map and traffic information provider TomTom have now become the first to create a high-resolution map with a localisation layer using radar reflection points – albeit solely for road vehicles. So far, video data has been used for this purpose. Bosch’s “radar road signature” is made up of billions of individual reflection points. These are formed everywhere that radar signals hit – for example, on crash barriers or road signs – and reproduce the course a road takes. Automated vehicles can use the map to determine their exact location in a lane down to a few centimetres. The huge advantage of the radar map is its robustness: localisation with the radar road signature also works reliably at night and in conditions of poor visibility. Moreover, only five kilobytes of data are transmitted to a Cloud per kilometre. This data volume is at least twice as high using a video map. It is expected that by 2020 at the latest, the first vehicles will provide data for the radar road signature in Europe and the U.S. “Cars arriving on the market in years to come with the assistance functions of tomorrow will be running the map for the automated vehicles of the future,” says Bosch Board Member Dr Dirk Hoheisel.